Learning Representations From Imperfect Time Series Data Via Tensor Rank Regularization
57TH ANNUAL MEETING OF THE ASSOCIATION FOR COMPUTATIONAL LINGUISTICS (ACL 2019)(2019)
摘要
There has been an increased interest in multimodal language processing including multimodal dialog, question answering, sentiment analysis, and speech recognition. However, naturally occurring multimodal data is often imperfect as a result of imperfect modalities, missing entries or noise corruption. To address these concerns, we present a regularization method based on tensor rank minimization. Our method is based on the observation that high-dimensional multimodal time series data often exhibit correlations across time and modalities which leads to low-rank tensor representations. However, the presence of noise or incomplete values breaks these correlations and results in tensor representations of higher rank. We design a model to learn such tensor representations and effectively regularize their rank. Experiments on multimodal language data show that our model achieves good results across various levels of imperfection.
更多查看译文
关键词
imperfect time series data,tensor rank
AI 理解论文
溯源树
样例
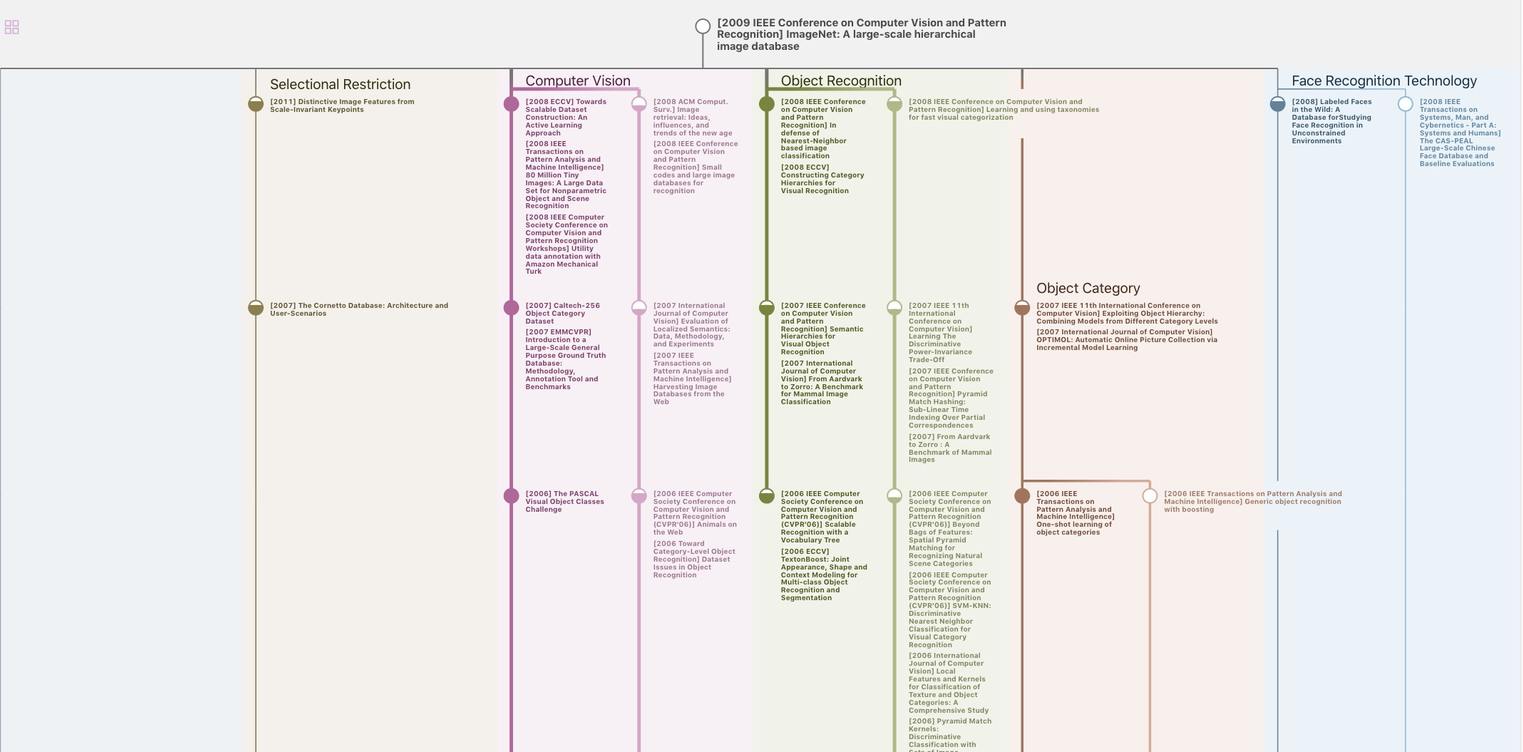
生成溯源树,研究论文发展脉络
Chat Paper
正在生成论文摘要