Composite Neural Learning-Based Nonsingular Terminal Sliding Mode Control of MEMS Gyroscopes.
IEEE transactions on neural networks and learning systems(2020)
摘要
The efficient driving control of MEMS gyroscopes is an attractive way to improve the precision without hardware redesign. This paper investigates the sliding mode control (SMC) for the dynamics of MEMS gyroscopes using neural networks (NNs). Considering the existence of the dynamics uncertainty, the composite neural learning is constructed to obtain higher tracking precision using the serial–parallel estimation model (SPEM). Furthermore, the nonsingular terminal SMC (NTSMC) is proposed to achieve finite-time convergence. To obtain the prescribed performance, a time-varying barrier Lyapunov function (BLF) is introduced to the control scheme. Through simulation tests, it is observed that under the BLF-based NTSMC with composite learning design, the tracking precision of MEMS gyroscopes is highly improved.
更多查看译文
关键词
Micromechanical devices,Gyroscopes,Artificial neural networks,Estimation,Sliding mode control,Uncertainty,Damping
AI 理解论文
溯源树
样例
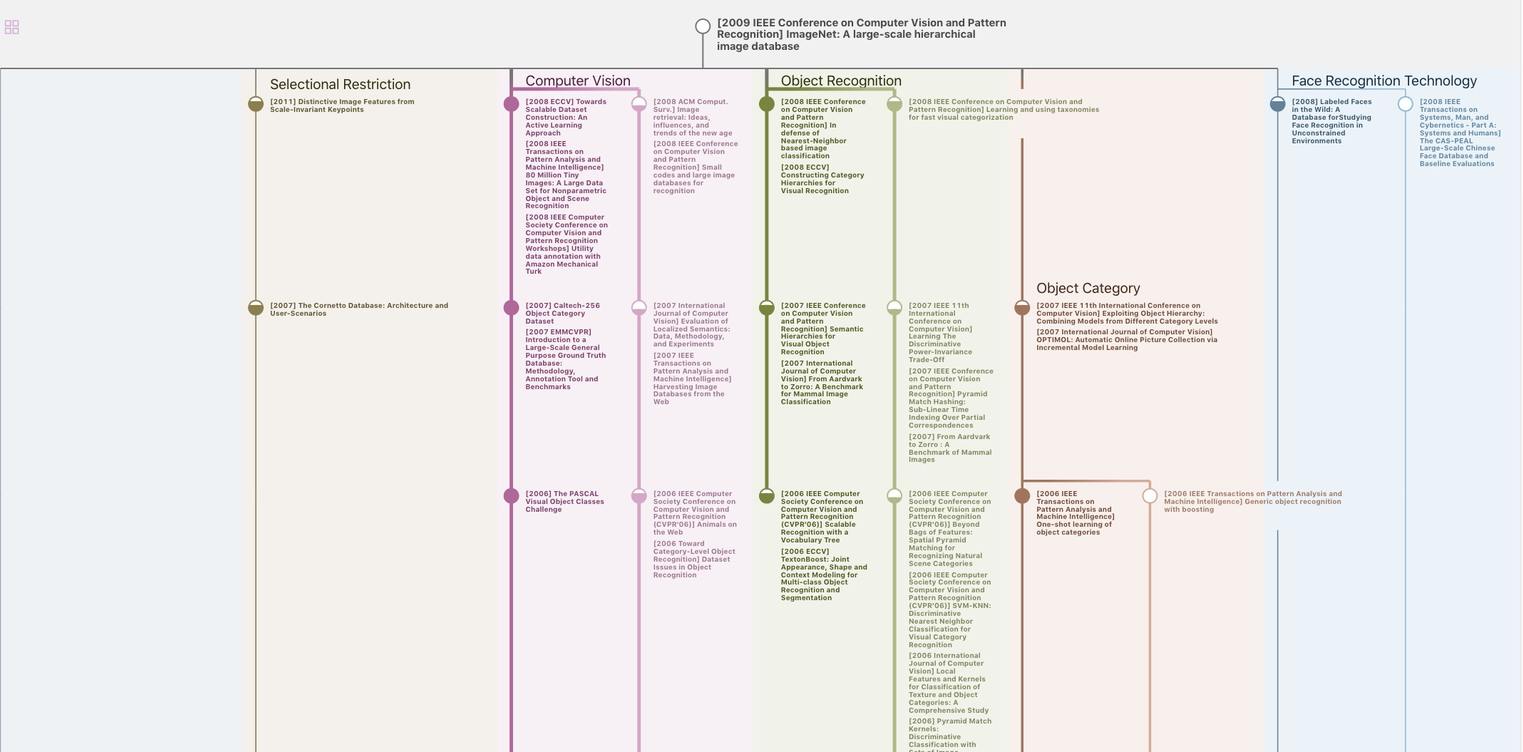
生成溯源树,研究论文发展脉络
Chat Paper
正在生成论文摘要