Coloringwith Limited Data: Few-Shot Colorization Via Memory Augmented Networks
2019 IEEE/CVF Conference on Computer Vision and Pattern Recognition (CVPR)(2019)
摘要
Despite recent advancements in deep learning-based automatic colorization, they are still limited when it comes to few-shot learning. Existing models require a significant amount of training data. To tackle this issue, we present a novel memory-augmented colorization model MemoPainter that can produce high-quality colorization with limited data. In particular, our model is able to capture rare instances and successfully colorize them. We also propose a novel threshold triplet loss that enables unsupervised training of memory networks without the need of class labels. Experiments show that our model has superior quality in both few-shot and one-shot colorization tasks.
更多查看译文
关键词
Deep Learning,Computational Photography, Computer Vision Theory, Image and Video Synthesis, Vision + Graphics , Vi
AI 理解论文
溯源树
样例
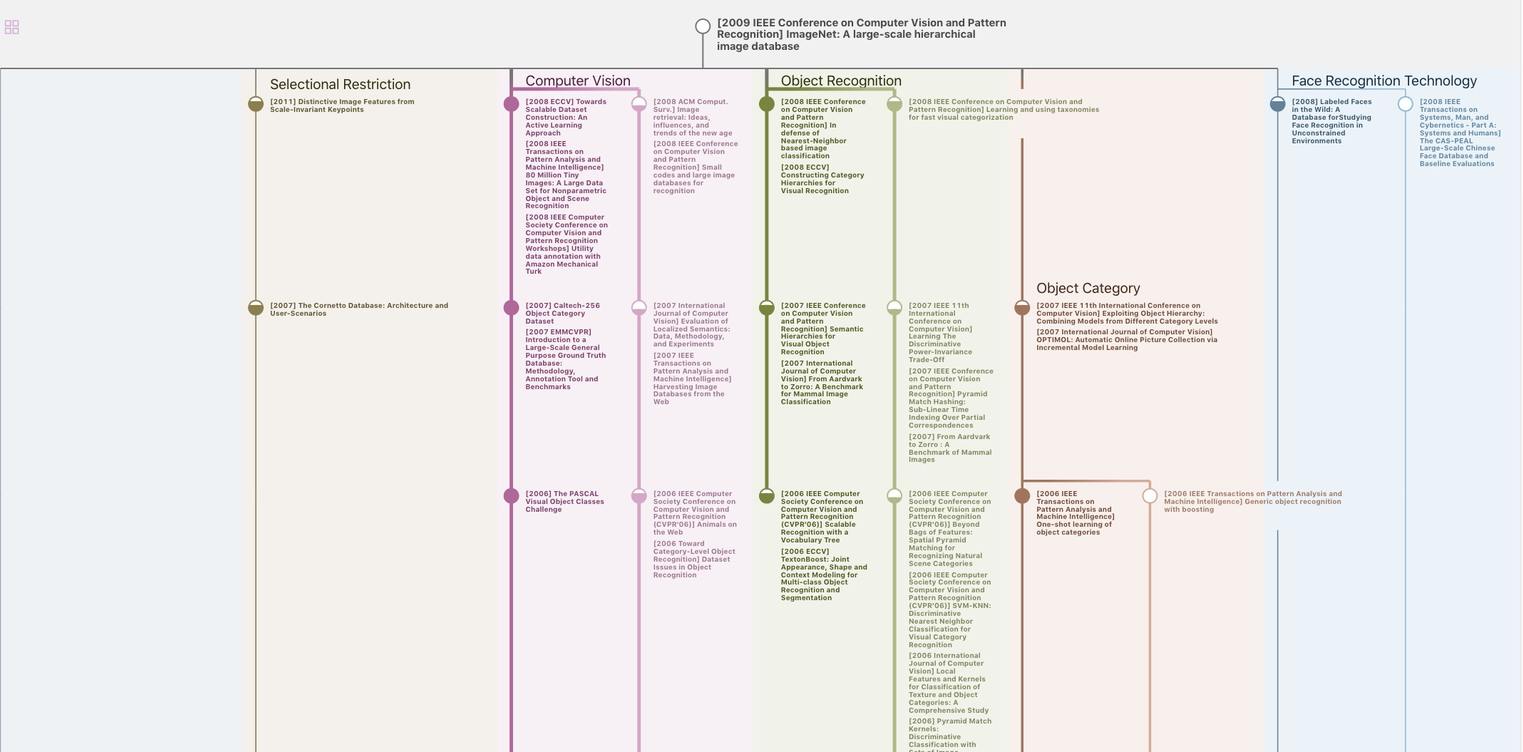
生成溯源树,研究论文发展脉络
Chat Paper
正在生成论文摘要