Deceptive Reviews Detection Using Deep Learning Techniques.
Lecture Notes in Computer Science(2019)
摘要
With the increasing influence of online reviews in shaping customer decision-making and purchasing behavior, many unscrupulous businesses have a vested interest in generating and posting deceptive reviews. Deceptive reviews are fictitious reviews written deliberately to sound authentic and deceive the consumers. Traditional deceptive reviews detection methods are based on various handcrafted features, including linguistic and psychological, which characterize the deceptive reviews. However, the proposed deep learning methods have better self-adaptability to extract the desired features implicitly and outperform all traditional methods. We have proposed multiple Deep Neural Network (DNN) based approaches for deceptive reviews detection and have compared the performances of these models on multiple benchmark datasets. Additionally, we have identified a common problem of handling the variable lengths of these reviews. We have proposed two different methods - Multi-Instance Learning and Hierarchical architecture to handle the variable length review texts. Experimental results on multiple benchmark datasets of deceptive reviews have outperformed existing state-of-the-art. We evaluated the performance of the proposed method on other review-related task-like review sentiment detection as well and achieved state-of-the-art accuracies on two benchmark datasets for the same.
更多查看译文
关键词
Deceptive reviews,Fake reviews,Deep learning,Convolutional neural network,Recurrent neural network,Word embedding
AI 理解论文
溯源树
样例
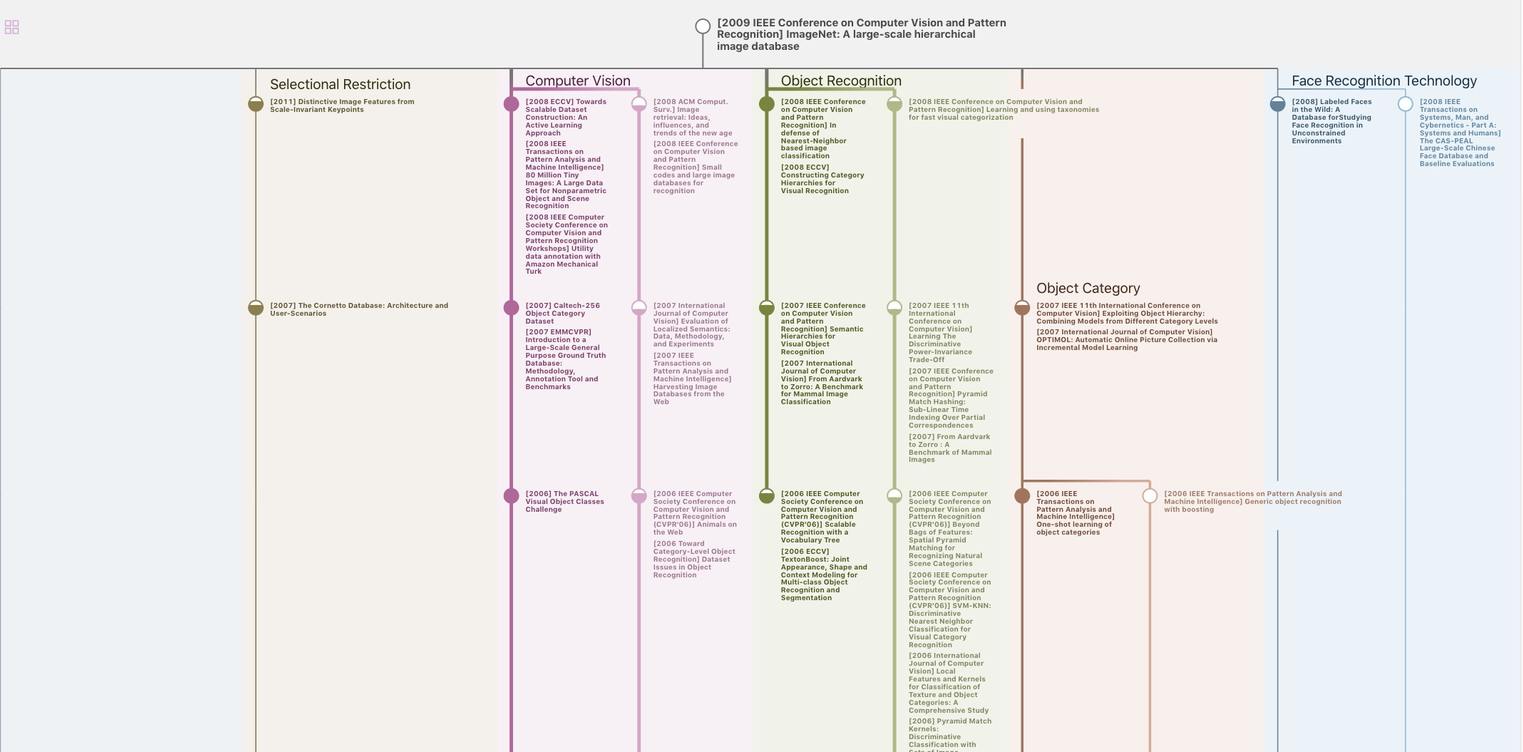
生成溯源树,研究论文发展脉络
Chat Paper
正在生成论文摘要