Explaining Deep Learning Models with Constrained Adversarial Examples
PRICAI 2019: TRENDS IN ARTIFICIAL INTELLIGENCE, PT I(2019)
摘要
Machine learning algorithms generally suffer from a problem of explainability. Given a classification result from a model, it is typically hard to determine what caused the decision to be made, and to give an informative explanation. We explore a new method of generating counterfactual explanations, which instead of explaining why a particular classification was made explain how a different outcome can be achieved. This gives the recipients of the explanation a better way to understand the outcome, and provides an actionable suggestion. We show that the introduced method of Constrained Adversarial Examples (CADEX) can be used in real world applications, and yields explanations which incorporate business or domain constraints such as handling categorical attributes and range constraints.
更多查看译文
关键词
Explainable AI,Adversarial examples,Counerfactual explanations
AI 理解论文
溯源树
样例
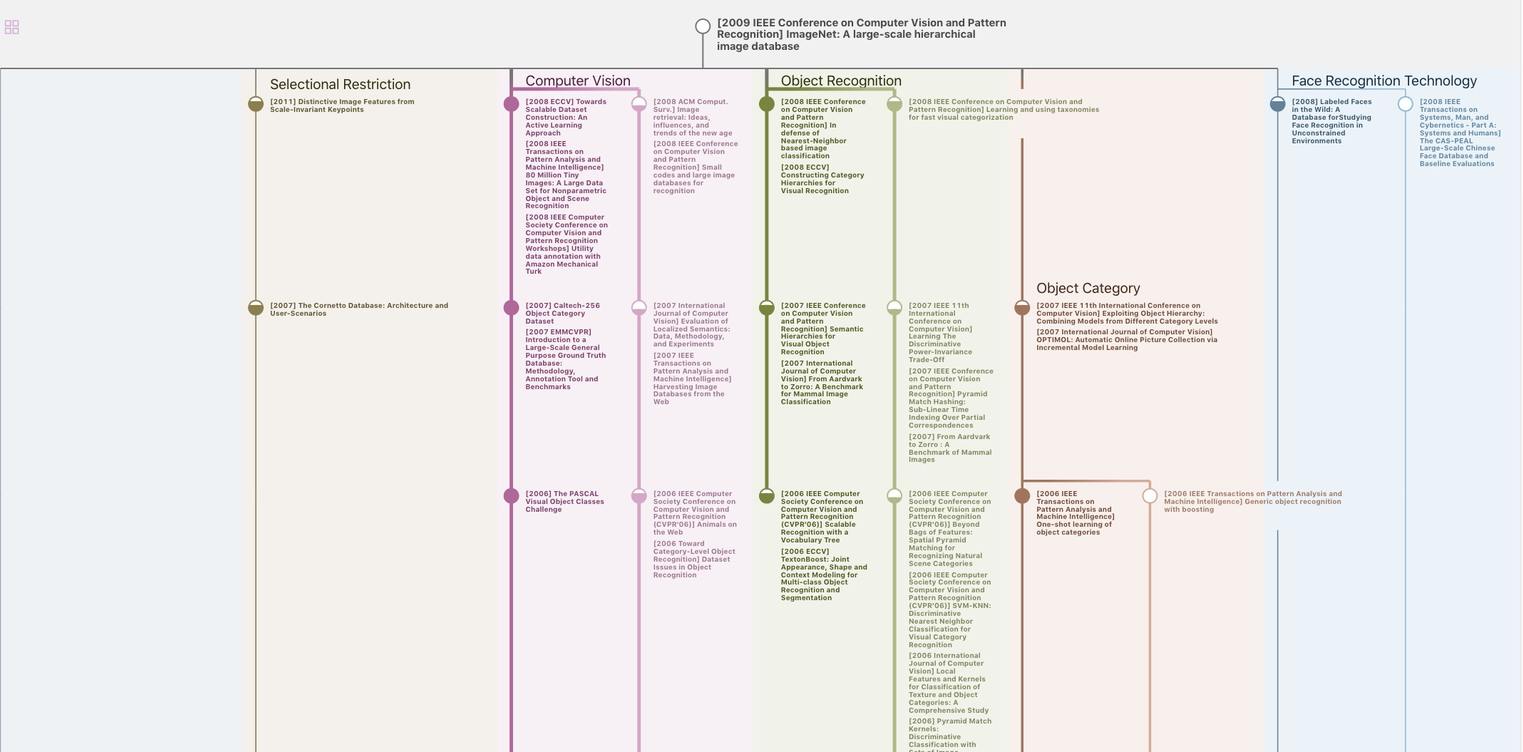
生成溯源树,研究论文发展脉络
Chat Paper
正在生成论文摘要