Complexity of Highly Parallel Non-Smooth Convex Optimization.
ADVANCES IN NEURAL INFORMATION PROCESSING SYSTEMS 32 (NIPS 2019)(2019)
摘要
A landmark result of non-smooth convex optimization is that gradient descent is an optimal algorithm whenever the number of computed gradients is smaller than the dimension d. In this paper we study the extension of this result to the parallel optimization setting. Namely we consider optimization algorithms interacting with a highly parallel gradient oracle, that is one that can answer poly(d) gradient queries in parallel. We show that in this case gradient descent is optimal only up to (O) over tilde(root d) rounds of interactions with the oracle. The lower bound improves upon a decades old construction by Nemirovski which proves optimality only up to d(1/3) rounds (as recently observed by Balkanski and Singer), and the suboptimality of gradient descent after root d rounds was already observed by Duchi, Bartlett and Wainwright. In the latter regime we propose a new method with improved complexity, which we conjecture to be optimal. The analysis of this new method is based upon a generalized version of the recent results on optimal acceleration for highly smooth convex optimization.
更多查看译文
AI 理解论文
溯源树
样例
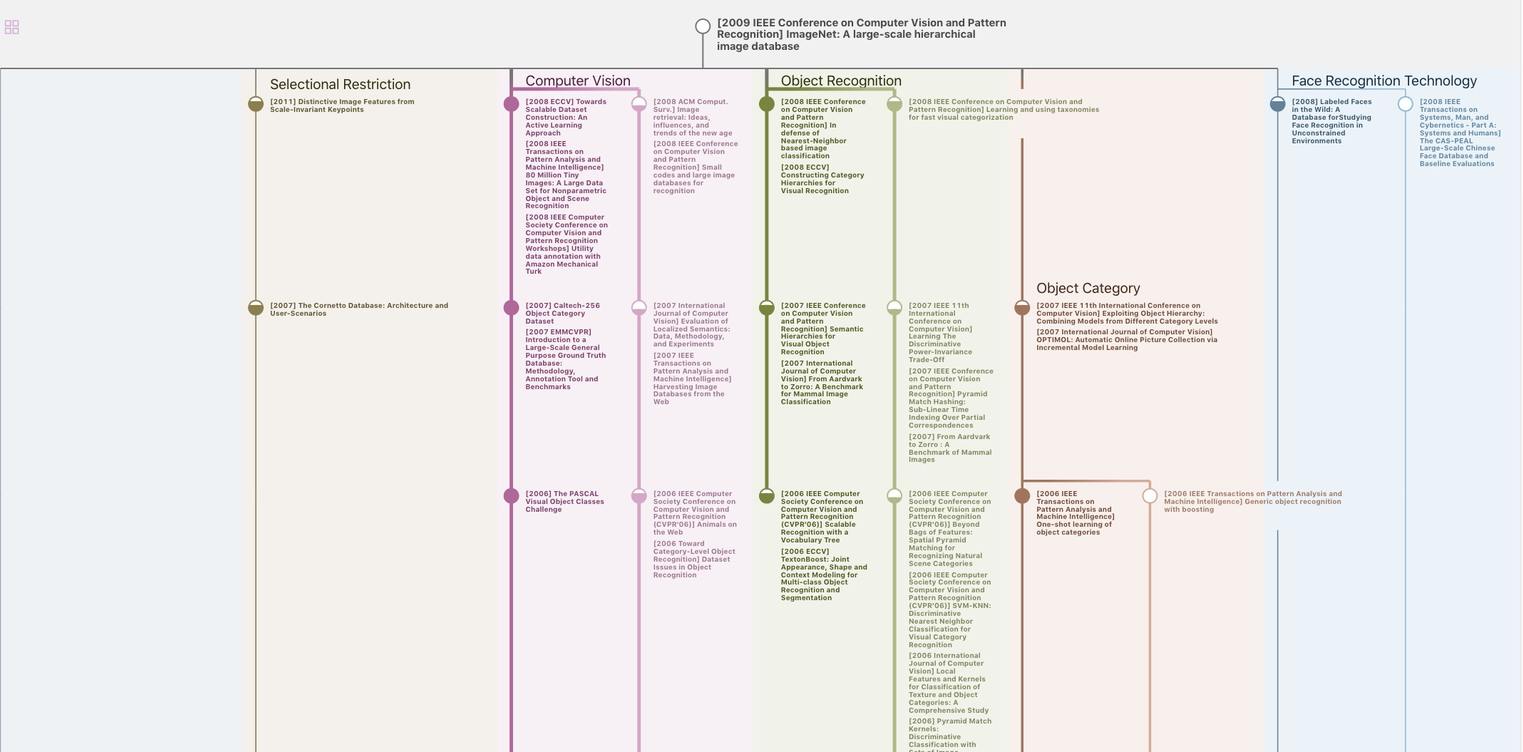
生成溯源树,研究论文发展脉络
Chat Paper
正在生成论文摘要