Bayesian clustering for continuous-time hidden Markov models
arxiv(2023)
摘要
We develop clustering procedures for longitudinal trajectories based on a continuous-time hidden Markov model (CTHMM) and a generalized linear observation model. Specifically, in this article we carry out finite and infinite mixture model-based clustering for a CTHMM and achieve inference using Markov chain Monte Carlo (MCMC). For a finite mixture model with a prior on the number of components, we implement reversible-jump MCMC to facilitate the trans-dimensional move between models with different numbers of clusters. For a Dirichlet process mixture model, we utilize restricted Gibbs sampling split-merge proposals to improve the performance of the MCMC algorithm. We apply our proposed algorithms to simulated data as well as a real-data example, and the results demonstrate the desired performance of the new sampler. (C) 2021 Statistical Society of Canada
更多查看译文
关键词
Continuous-time hidden Markov models,mixture models,model-based clustering,nonparametric Bayesian inference,reversible-jump MCMC,split-merge proposal
AI 理解论文
溯源树
样例
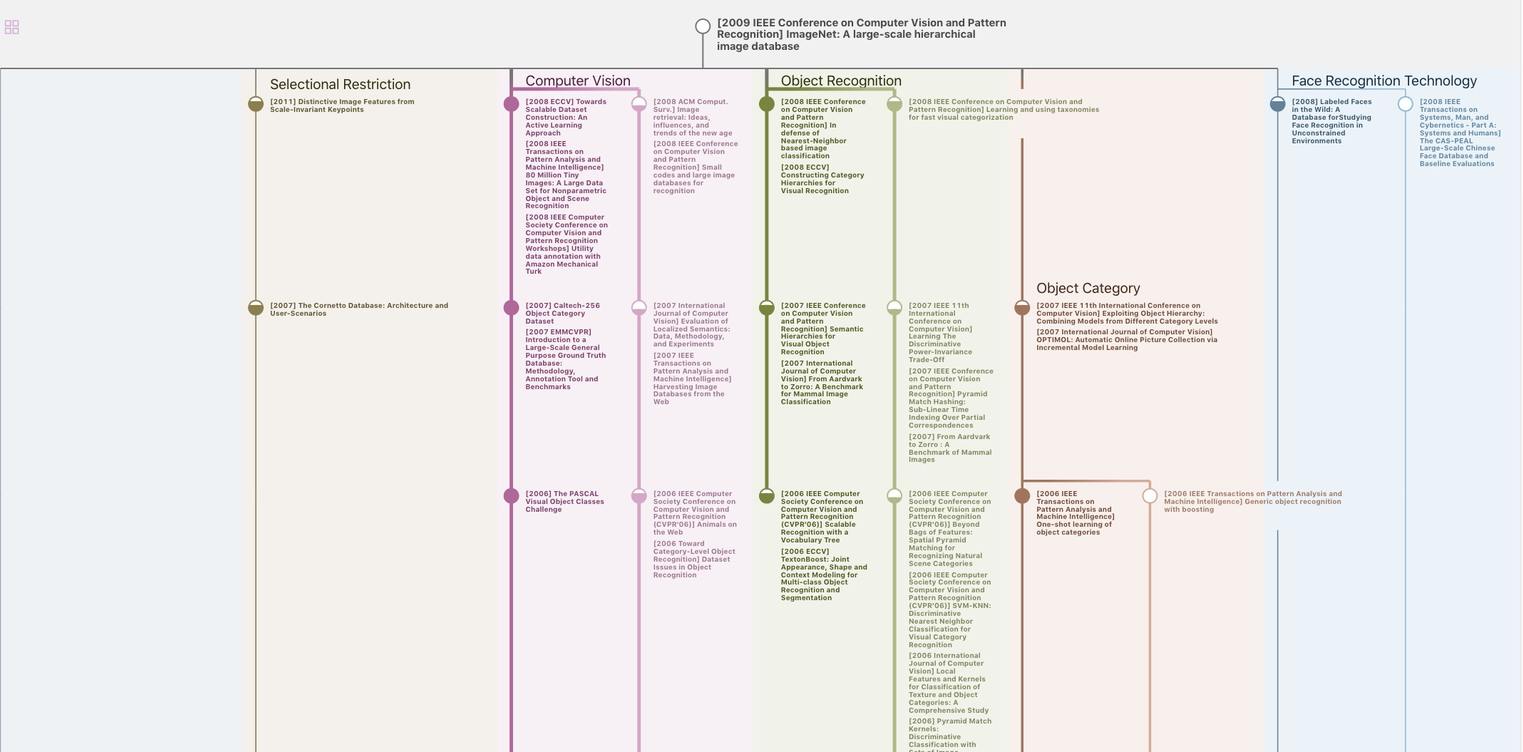
生成溯源树,研究论文发展脉络
Chat Paper
正在生成论文摘要