Towards a unified model of event-related potentials as phases of stimulus-to-response processing.
Neuropsychologia(2019)
摘要
This study demonstrates the utility of combining principles of connectionist theory with a sophisticated statistical approach, structural equation modeling (SEM), to better understand brain-behavior relationships in studies using event-related potentials (ERPs). The models show how sequential phases of neural processing measured by averaged ERP waveform components can successfully predict task behavior (response time; RT) while accounting for individual differences in maturation and sex. The models assume that all ERP measures are affected by individual differences in physical and mental state that inflate measurement error. ERP data were collected from 154 neurotypical children (7-13 years, M = 10.22, SD = 1.48; 74 males) performing a cued Go/No-Go task during two separate sessions. Using SEM, we show a latent variable path model with good fit (e.g., χ2(51) = 56.20, p = .25; RMSEA = .03; CFI = .99; SRMR = .06) yielding moderate-to-large predictive coefficients from N1 through the E-wave latent variables (N1 β = -.29 → P2 β = -.44 → N2 β = .28 → P3 β =.64→ E-wave), which in turn significantly predicted RT (β =.34, p = .02). Age significantly related to N1 and P3 latent variables as well as RT (β =.31, -.58, & -.40 respectively), and Sex significantly related to the E-wave latent variable and RT (β =.36 & 0.21 respectively). Additionally, the final model suggested that individual differences in emotional and physical state accounted for a significant proportion of variance in ERP measurements, and that individual states systematically varied across sessions (i.e., the variance was not just random noise). These findings suggest that modeling ERPs as a system of inter-related processes may be a more informative approach to examining brain-behavior relationships in neurotypical and clinical groups than traditional analysis techniques.
更多查看译文
AI 理解论文
溯源树
样例
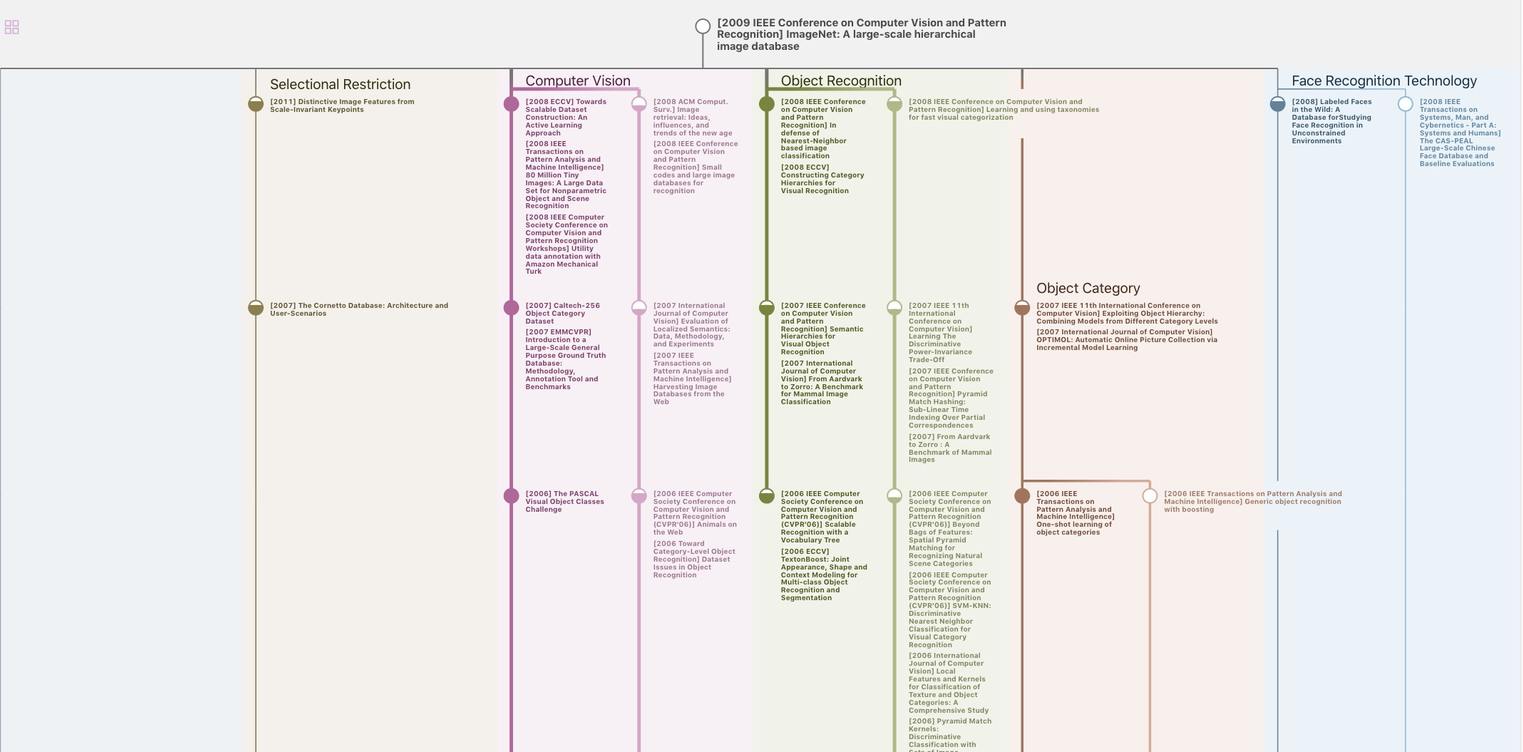
生成溯源树,研究论文发展脉络
Chat Paper
正在生成论文摘要