Scalable Bayesian Dynamic Covariance Modeling With Variational Wishart And Inverse Wishart Processes
ADVANCES IN NEURAL INFORMATION PROCESSING SYSTEMS 32 (NIPS 2019)(2019)
摘要
We implement gradient-based variational inference routines for Wishart and inverse Wishart processes, which we apply as Bayesian models for the dynamic, heteroskedastic covariance matrix of a multivariate time series. The Wishart and inverse Wishart processes are constructed from i.i.d. Gaussian processes, existing variational inference algorithms for which form the basis of our approach. These methods are easy to implement as a black-box and scale favorably with the length of the time series, however, they fail in the case of the Wishart process, an issue we resolve with a simple modification into an additive white noise parameterization of the model. This modification is also key to implementing a factored variant of the construction, allowing inference to additionally scale to high-dimensional covariance matrices. Through experimentation, we demonstrate that some (but not all) model variants outperform multivariate GARCH when forecasting the covariances of returns on financial instruments.
更多查看译文
关键词
gaussian processes,time series,financial instruments
AI 理解论文
溯源树
样例
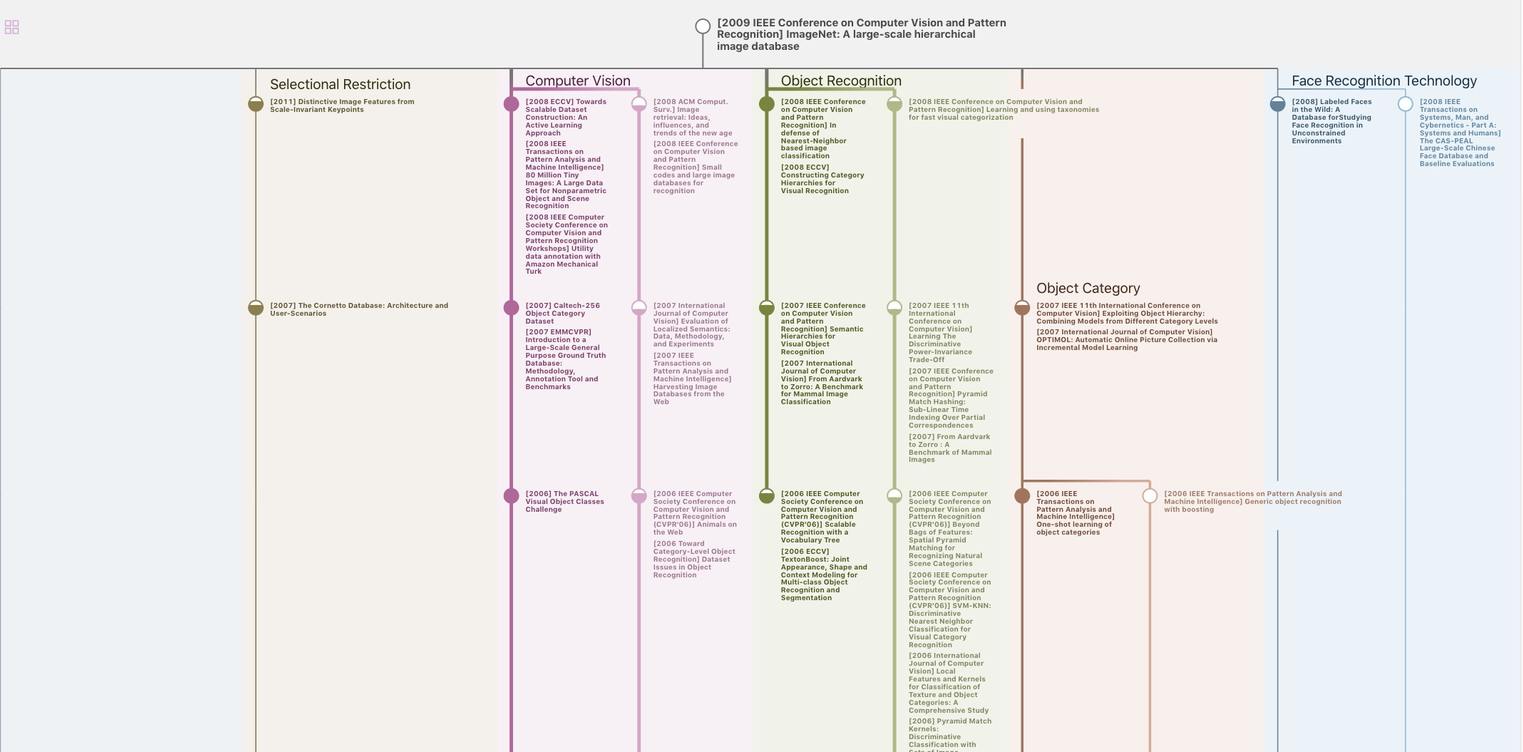
生成溯源树,研究论文发展脉络
Chat Paper
正在生成论文摘要