Approximate Optimization, Sampling, And Spin-Glass Droplet Discovery With Tensor Networks
PHYSICAL REVIEW E(2021)
摘要
We devise a deterministic algorithm to efficiently sample high-quality solutions of certain spin-glass systems that encode hard optimization problems. We employ tensor networks to represent the Gibbs distribution of all possible configurations. Using approximate tensor-network contractions, we are able to efficiently map the lowenergy spectrum of some quasi-two-dimensional Hamiltonians. We exploit the local nature of the problems to compute spin-glass droplets geometries, which provides a new form of compression of the low-energy spectrum. It naturally extends to sampling, which otherwise, for exact contraction, is #P-complete. In particular, for one of the hardest known problem-classes devised on chimera graphs known as deceptive cluster loops and for up to 2048 spins, we find on the order of 1010 degenerate ground states in a single run of our algorithm, computing better solutions than have been reported on some hard instances. Our gradient-free approach could provide new insight into the structure of disordered spin-glass complexes, with ramifications both for machine learning and noisy intermediate-scale quantum devices.
更多查看译文
AI 理解论文
溯源树
样例
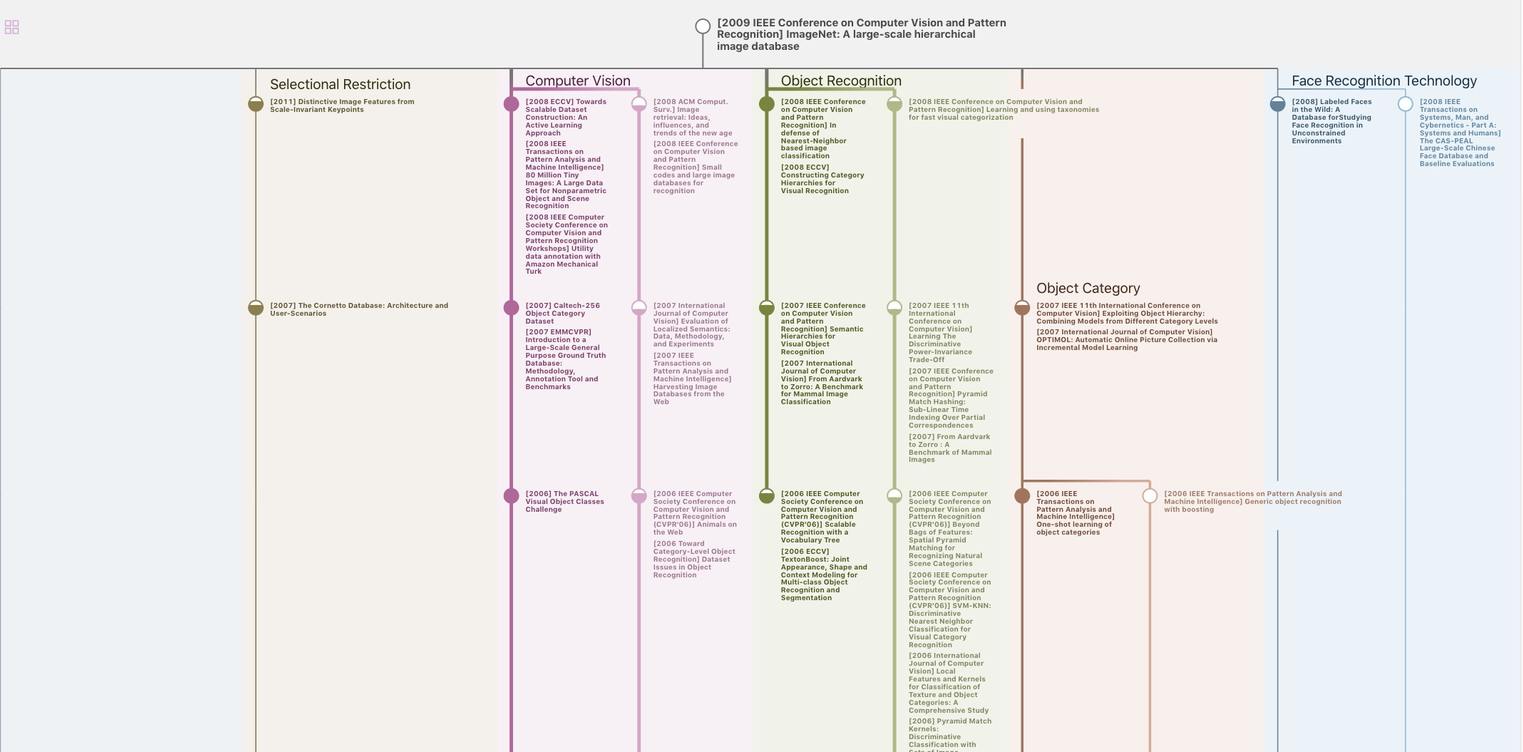
生成溯源树,研究论文发展脉络
Chat Paper
正在生成论文摘要