Pursuing Optimal Prediction Of Discharge Time In Icus With Machine Learning Methods
ARTIFICIAL INTELLIGENCE IN MEDICINE, AIME 2019(2019)
摘要
In hospital intensive care units (ICU), patients are under continuous evaluation. One of the purposes of this evaluation is to determine the expected number of days to discharge. This value is important to manage ICUs. Some studies show that health care professionals are good at predicting short-term discharge times, but not as good at long-term predictions. Machine learning methods can achieve 1.79-day average prediction error. We performed a study on 3,787 patient-days in the ICU of the Hospital Joan XXIII (Spain) to obtain a data-driven model to predict the discharge time of ICU patients, in a daily basis. Our model, which is based on random forest technology, obtained an error of 1.34 days. We studied the progression of the model as more data are available and predicted that the number of instances required to reduce the error below one day is 4,745. When we trained the model with all the available data, we obtained a mean error of less than half a day with a coefficient of determination (R2) above 97% in their predictions on either ICU survivors and not survivors. Similar results were obtained differentiating by patients' gender and age, confirming our approach as a good means to achieve optimal performance when more data will be available.
更多查看译文
关键词
Intelligent data analysis, Intensive care units, Discharge time prediction, Data-driven models
AI 理解论文
溯源树
样例
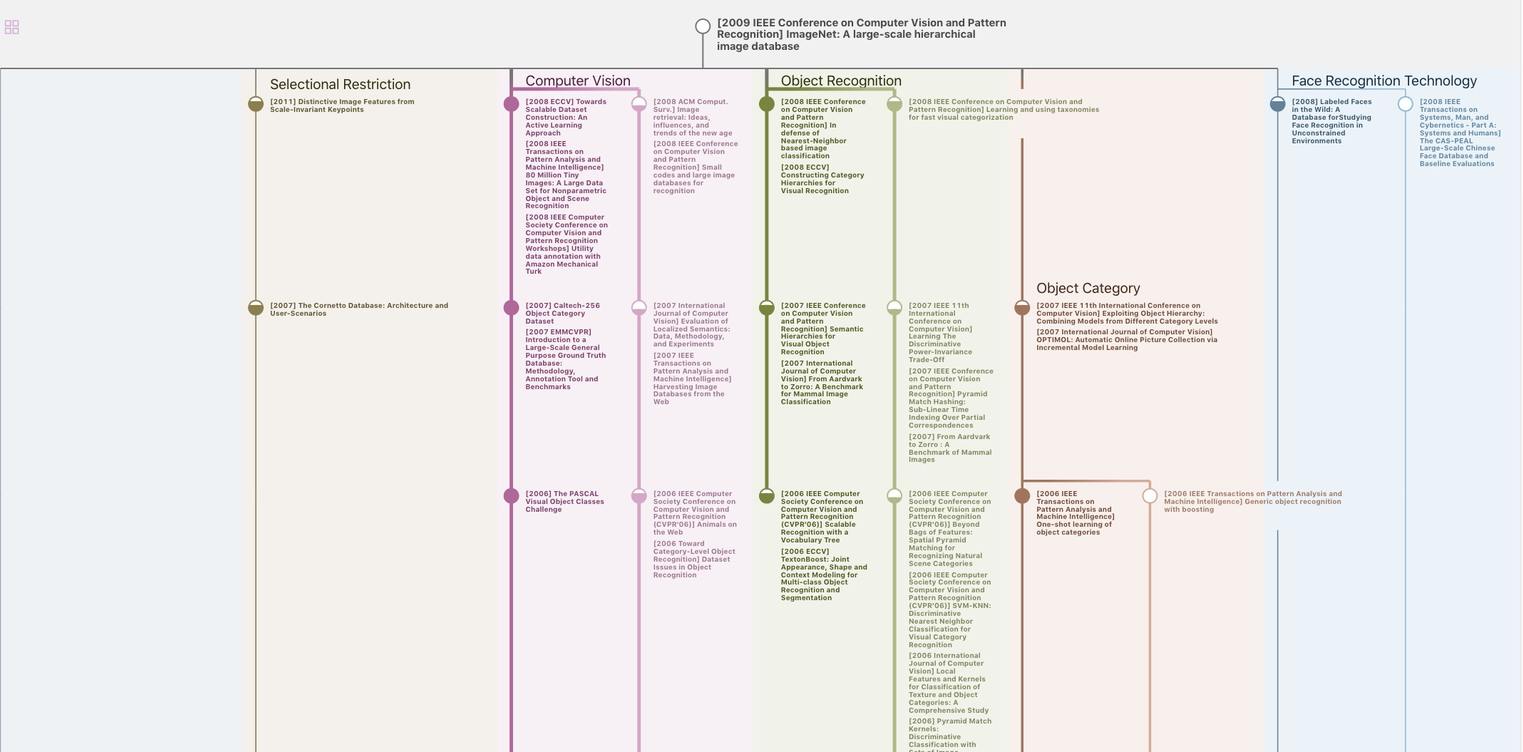
生成溯源树,研究论文发展脉络
Chat Paper
正在生成论文摘要