Multimodal Deep Neural Networks Based Ensemble Learning For X-Ray Object Recognition
COMPUTER VISION - ACCV 2018 WORKSHOPS(2018)
摘要
X-ray object recognition is essential to reduce the workload of human inspectors regarding X-ray baggage screening and improve the throughput of X-ray screening. Traditionally, researchers focused on single-view or multiple views object recognition from only one type of pseudo X-ray image generated from X-ray energy data (e.g., dual-energy or mono-energy X-ray image). It is known that different types of X-ray images represent different object characteristics (e.g., material or density). Thus, effectively using different types of X-ray images as multiple modalities is promising to achieve more reliable recognition performance. In this paper, we explore different stage of ensemble approaches for X-ray object-recognition and propose an approach that exploits a classifier ensemble by using the multimodality information of X-ray images on a single-view object. We use a deep neural network to learn a good representation for each modality, which is used to train the base classifiers. To ensure high overall classification performance, the reliabilities of the base classifiers are estimated by taking the inherent features (e.g., color and shape) of an object in an X-ray image into consideration. We conducted experiments to evaluate the competitive performance of our method using a 15 classes dataset.
更多查看译文
AI 理解论文
溯源树
样例
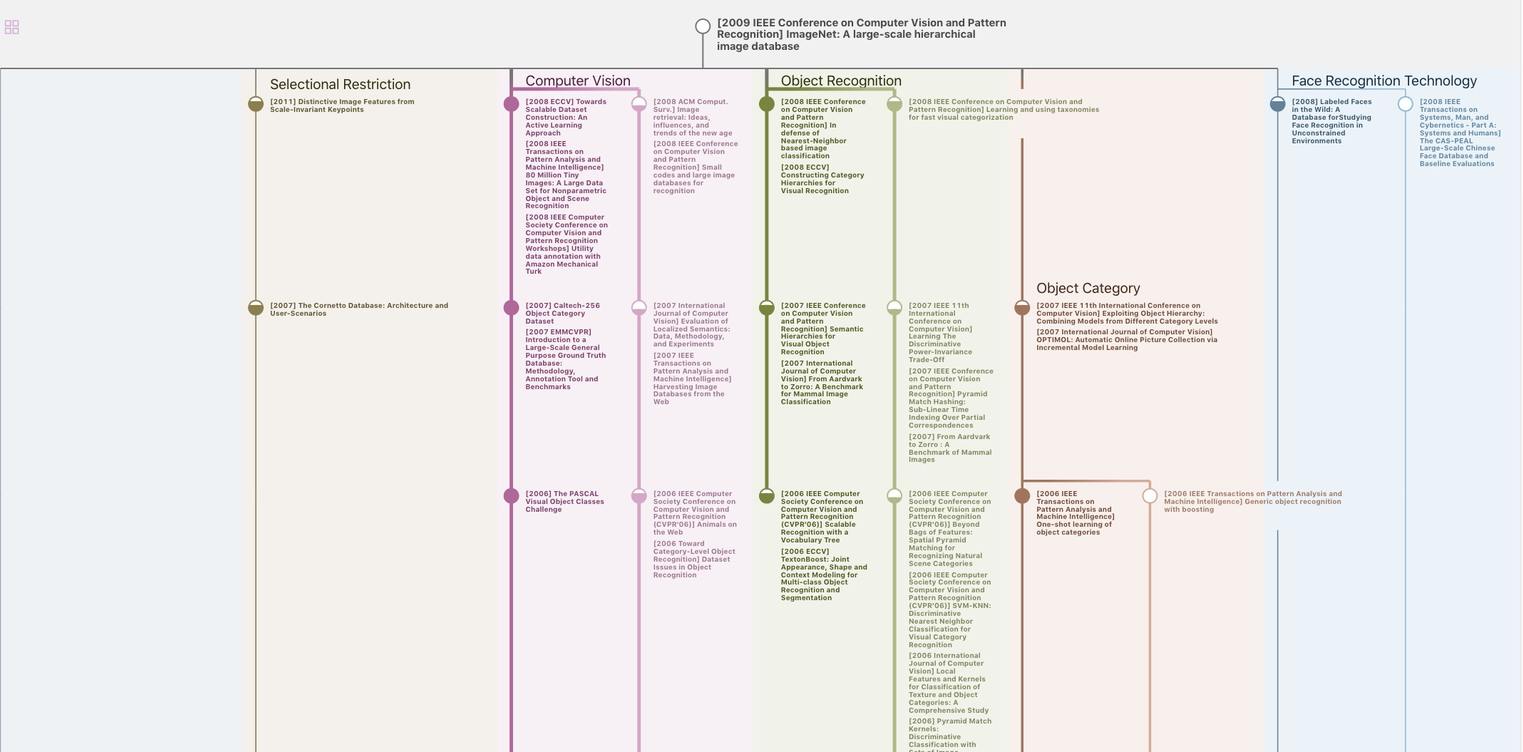
生成溯源树,研究论文发展脉络
Chat Paper
正在生成论文摘要