NFVdeep: Adaptive Online Service Function Chain Deployment with Deep Reinforcement Learning
2019 IEEE/ACM 27th International Symposium on Quality of Service (IWQoS)(2019)
摘要
With the evolution of network function virtualization (NFV), diverse network services can be flexibly offered as service function chains (SFCs) consisted of different virtual network functions (VNFs). However, network state and traffic typically exhibit unpredictable variations due to stochastically arriving requests with different quality of service (QoS) requirements. Thus, an adaptive online SFC deployment approach is needed to handle the real-time network variations and various service requests. In this paper, we firstly introduce a Markov decision process (MDP) model to capture the dynamic network state transitions. In order to jointly minimize the operation cost of NFV providers and maximize the total throughput of requests, we propose NFVdeep, an adaptive, online, deep reinforcement learning approach to automatically deploy SFCs for requests with different QoS requirements. Specifically, we use a serialization-and-backtracking method to effectively deal with large discrete action space. We also adopt a policy gradient based method to improve the training efficiency and convergence to optimality. Extensive experimental results demonstrate that NFVdeep converges fast in the training process and responds rapidly to arriving requests especially in large, frequently transferred network state space. Consequently, NFVdeep surpasses the state-of-the-art methods by 32.59% higher accepted throughput and 33.29% lower operation cost on average.
更多查看译文
关键词
Network Function Virtualization (NFV),Deep Reinforcement Learning,Service Function Chain,QoS-Aware Resource Management
AI 理解论文
溯源树
样例
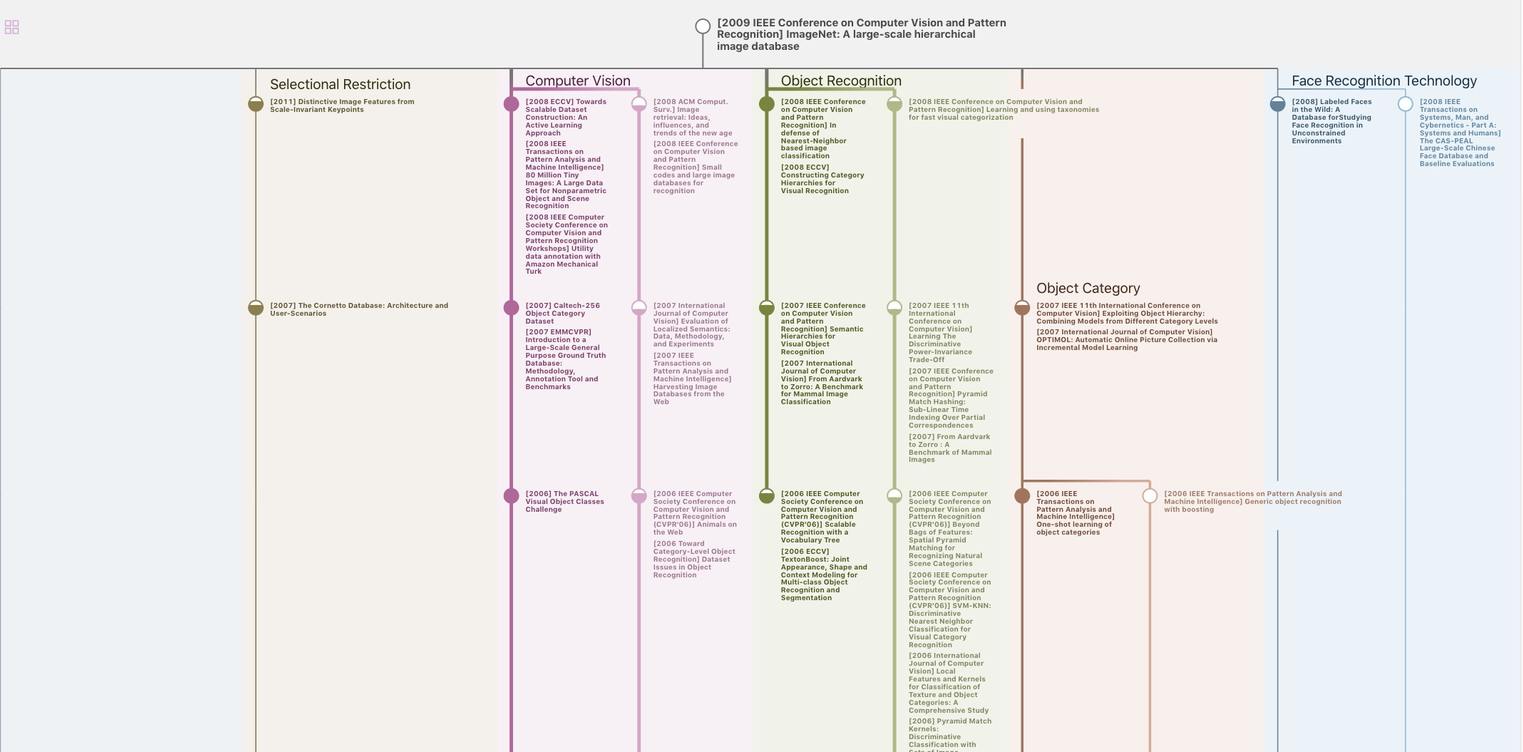
生成溯源树,研究论文发展脉络
Chat Paper
正在生成论文摘要