O(2)A: One-Shot Observational Learning With Action Vectors
FRONTIERS IN ROBOTICS AND AI(2021)
摘要
We present O(2)A, a novel method for learning to perform robotic manipulation tasks from a single (one-shot) third-person demonstration video. To our knowledge, it is the first time this has been done for a single demonstration. The key novelty lies in pre-training a feature extractor for creating a perceptual representation for actions that we call "action vectors". The action vectors are extracted using a 3D-CNN model pre-trained as an action classifier on a generic action dataset. The distance between the action vectors from the observed third-person demonstration and trial robot executions is used as a reward for reinforcement learning of the demonstrated task. We report on experiments in simulation and on a real robot, with changes in viewpoint of observation, properties of the objects involved, scene background and morphology of the manipulator between the demonstration and the learning domains. O(2)A outperforms baseline approaches under different domain shifts and has comparable performance with an Oracle (that uses an ideal reward function). Videos of the results, including demonstrations, can be found in our: project-website.
更多查看译文
关键词
observational learning, visual perception, reinforcement learning, transfer learning, robotic manipulation
AI 理解论文
溯源树
样例
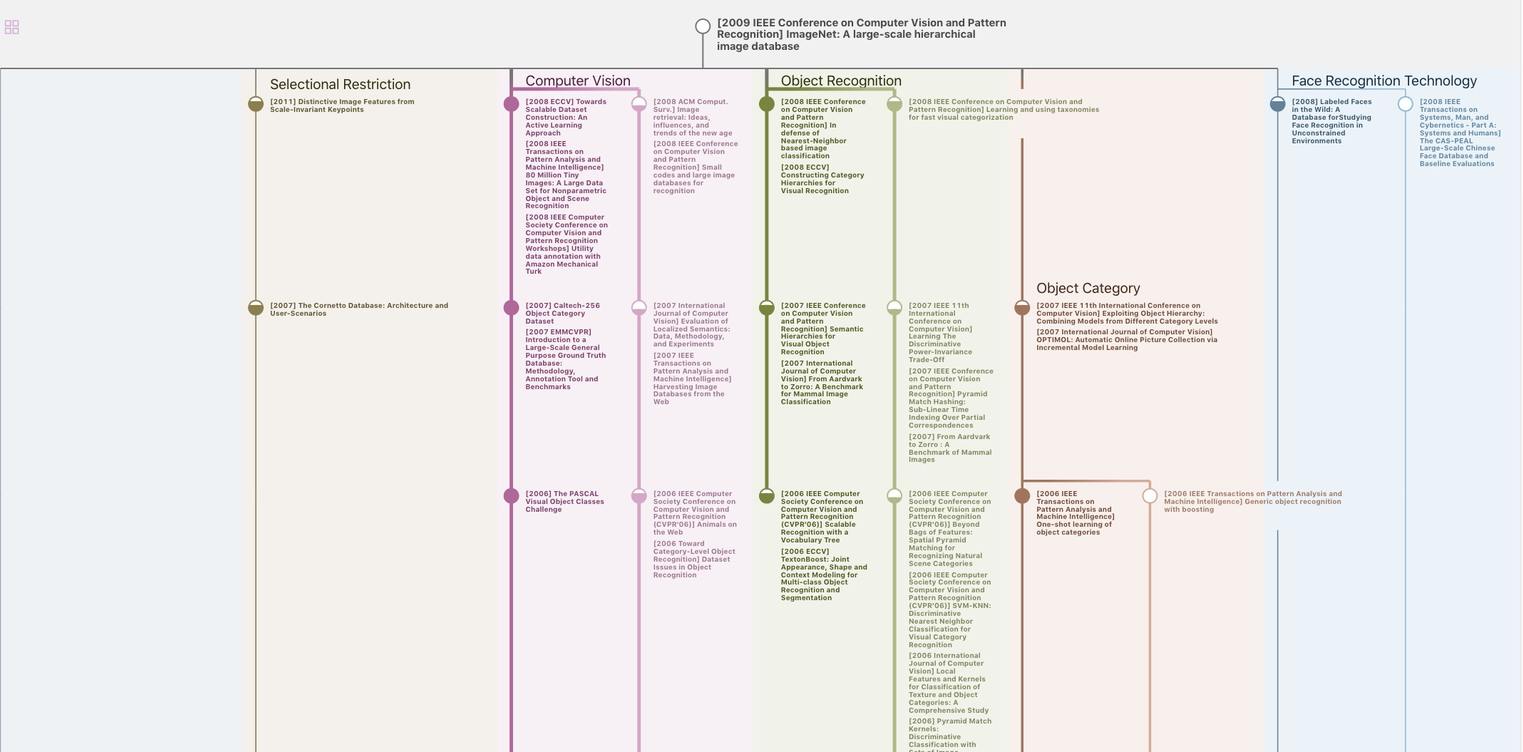
生成溯源树,研究论文发展脉络
Chat Paper
正在生成论文摘要