Deep Mixture Of Mrfs For Human Pose Estimation
COMPUTER VISION - ACCV 2018, PT III(2019)
摘要
In this paper, we propose a new geometric model based on mixture of Markov Random Fields (MRFs) for human pose estimation. We build on previous work that expresses the global constraints on the relative locations of the body joints using an auto-encoder ConvNet which performs dimensionality reduction on the heat maps, and recovers in this manner a low dimensional manifold on which the global pose of the human body lies. To address the shortcomings of this architecture, and obtain more meaningful vectors that span the low dimensional pose space, we propose to replace the auto-encoder network layer with a layer that implements a Gaussian mixture model (GMM) that provides a soft clustering of the human pose predictions in an online fashion. We show that: (a) a large number of meaningful global poses is feasible, this way preserving the underlying structure of informative body poses; (b) the clustering helps to properly initialize the MRF filters of each different global pose, (c) a body joint masking data augmentation procedure can be better exploited and that (d) the system stability is significantly improved. To the best of our knowledge, this is the first time that a clustering algorithm like GMM is used in an online fashion for the problem of 2D human pose estimation. The efficacy of our framework has been demonstrated through extensive experiments on widely used public benchmarks.
更多查看译文
关键词
Human pose estimation, Deep learning, Markov Random Fields
AI 理解论文
溯源树
样例
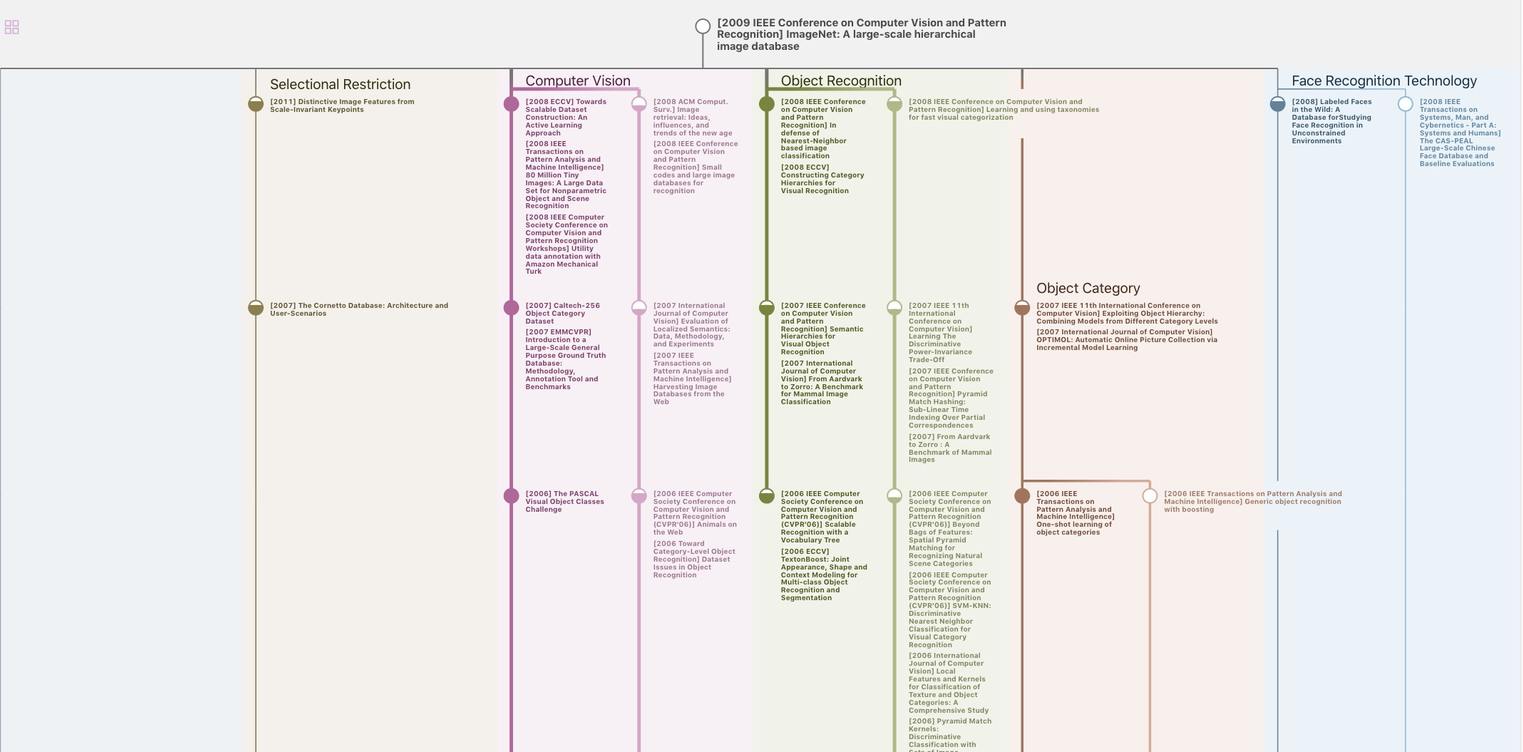
生成溯源树,研究论文发展脉络
Chat Paper
正在生成论文摘要