Machine learning-assisted early ignition prediction in a complex flow
Combustion and Flame(2019)
摘要
Machine learning methods are used to improve the efficiency by which turbulence-resolved simulations predict whether a hydrogen jet in air crossflow will successfully ignite. The flush-mounted jet issues perpendicularly from the wall of a low-speed wind tunnel into a turbulent boundary layer wherein a laser-induced optical breakdown (LIB) hotspot is deposited. A detailed hydrogen chemical mechanism is used to model the radicals and any subsequent chemical reactions. A dielectric-barrier discharge actuator generates body forces and hydrogen radicals near the jet orifice. We focus on the success or not of the ignition based on LIB location. A challenge is that definitive determination of this requires long simulations, up to 440 µs after the LIB deposition. This is particularly expensive since multiple simulations are required to find the threshold. To reduce the computational effort, three short-time (91 µs) criteria are proposed, evaluated, and compared: a constructed criterion based on detailed observations of radicals near the stoichiometric surface and two machine learning approaches, each trained on 38 realizations. The constructed criterion provides a low-cost estimate of the ignition boundary that is unambiguous in 45 of the 50 training and test trials, so only 10% of them would need to be simulated longer. The trained neural networks correctly predict outcomes in all cases evaluated, with the more automated procedure—a convolutional neural network (CNN) trained on two-dimensional images—providing the most definitive outcome prediction. From the CNN, a sensitivity analysis is used to determine which kernel features from the two-dimensional input data, as defined by intermediate-layer network weights, are important for identifying ignition.
更多查看译文
关键词
Ignition,Plasma-coupled combustion,Ignition prediction,Machine learning
AI 理解论文
溯源树
样例
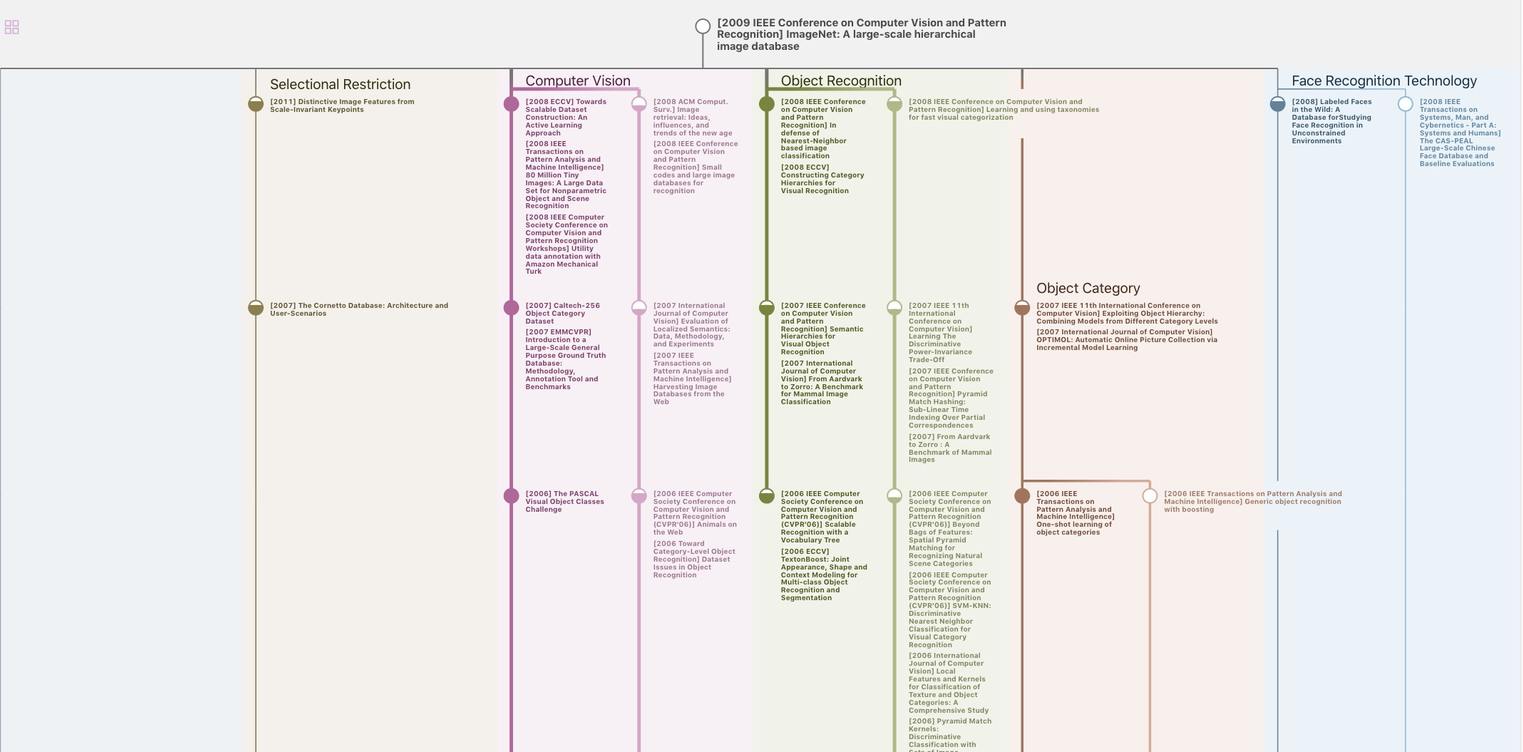
生成溯源树,研究论文发展脉络
Chat Paper
正在生成论文摘要