edge2vec: Representation learning using edge semantics for biomedical knowledge discovery
BMC Bioinformatics(2019)
摘要
Background Representation learning provides new and powerful graph analytical approaches and tools for the highly valued data science challenge of mining knowledge graphs. Since previous graph analytical methods have mostly focused on homogeneous graphs, an important current challenge is extending this methodology for richly heterogeneous graphs and knowledge domains. The biomedical sciences are such a domain, reflecting the complexity of biology, with entities such as genes, proteins, drugs, diseases, and phenotypes, and relationships such as gene co-expression, biochemical regulation, and biomolecular inhibition or activation. Therefore, the semantics of edges and nodes are critical for representation learning and knowledge discovery in real world biomedical problems. Results In this paper, we propose the edge2vec model, which represents graphs considering edge semantics. An edge-type transition matrix is trained by an Expectation-Maximization approach, and a stochastic gradient descent model is employed to learn node embedding on a heterogeneous graph via the trained transition matrix. edge2vec is validated on three biomedical domain tasks: biomedical entity classification, compound-gene bioactivity prediction, and biomedical information retrieval. Results show that by considering edge-types into node embedding learning in heterogeneous graphs, edge2vec significantly outperforms state-of-the-art models on all three tasks. Conclusions We propose this method for its added value relative to existing graph analytical methodology, and in the real world context of biomedical knowledge discovery applicability.
更多查看译文
关键词
Knowledge graph, Heterogeneous network, Biomedical knowledge discovery, Representation learning, Graph embedding, Node embedding, Edge semantics, Applied machine learning, Data science, Linked data, Semantic web, Network science, Systems biology
AI 理解论文
溯源树
样例
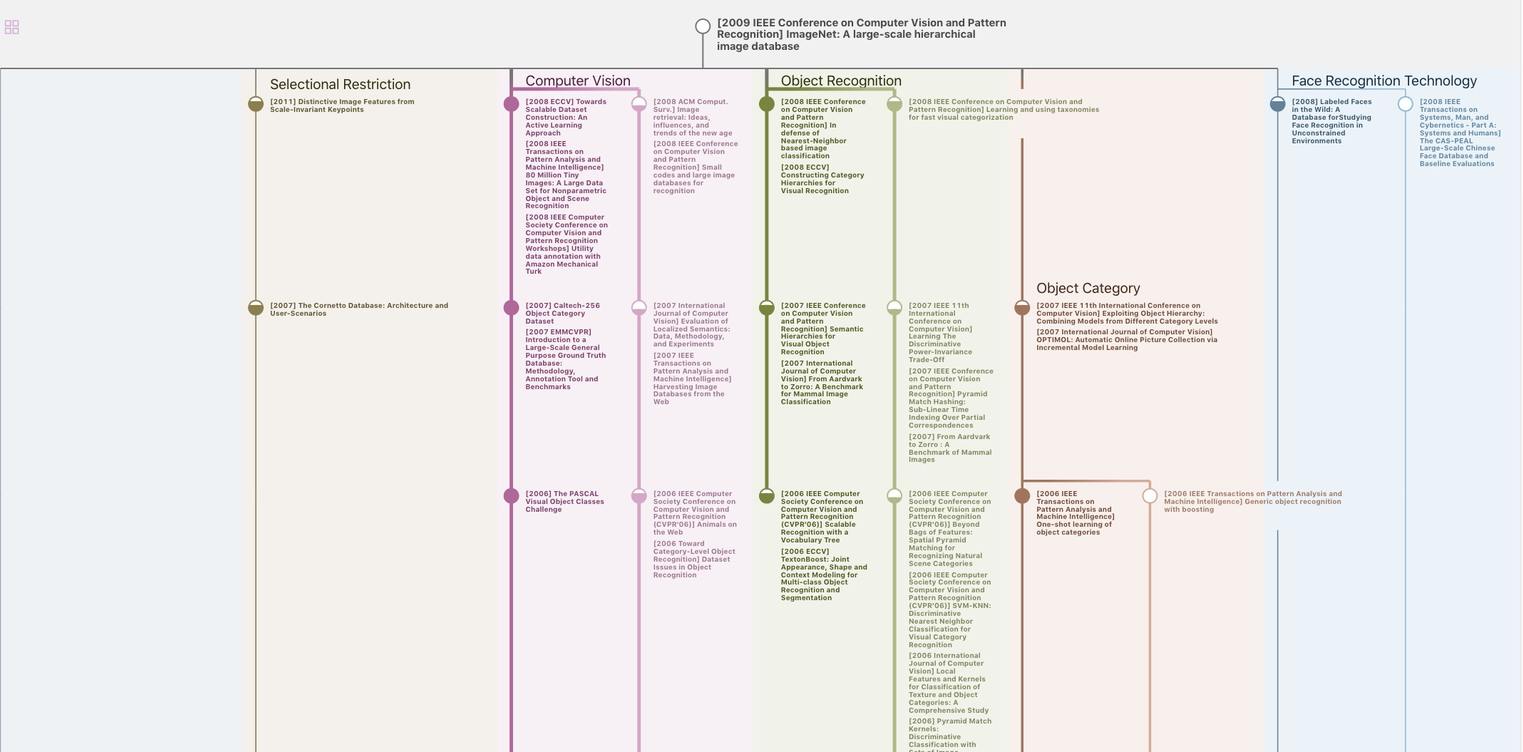
生成溯源树,研究论文发展脉络
Chat Paper
正在生成论文摘要