Mapping the activation landscape of the MAPKERK pathway for variants of unknown significance (VUS) using convolutional neural networks (CNN).
Journal of Clinical Oncology(2019)
摘要
e14615 Background: The MAPK\ERK signaling pathway is a major determinant in the control of diverse cellular processes. This pathway is often up-regulated in human tumors and as such has been an attractive target for the development of anticancer drugs. Mapping the activation landscape of MAPK\ERK is a necessary step in understanding tumor progression and determining the significance of a mutation as well as the relevance of available treatments. Here we present a CNN model, combined with an in-vitro system to investigate the activation landscape of various members of the MAPK\ERK pathway Methods: A novel multi-resolution multi-channel deep neural network was trained to classify images of cells that were transfected with known activating mutated forms of MAPK activating proteins versus WT forms of the proteins. The cells were also transfected with a fluorescently tagged ERK2 expression construct that was one of the three channels imaged by a fluorescent microscopy system. The trained network was subsequently tested on its ability to score images of cells that were transfected with VUS forms of the proteins, or images of cells that were transfected with VUS forms of MAPK proteins and were incubated with the relevant targeted therapies Results: We analyzed 6 proteins activating MAPK\ERK carrying over 100 unique VUS as well as many known oncogenic mutations and determined the pathogenicity score outputted by the network. Analysis of the performance of our model showed that our predictions exceed 95% accuracy and AUC > 0.95. Interestingly, analysis of deeper layers of the network revealed that each gene and mutation exhibit a unique ERK2 activation signal which is considerably different between active and non-active mutations as well as the ability to recognize what mutated gene was transfected, which means that each mutated gene induces different phenological attributes to the cells Conclusions: The use of deep-learning algorithms is providing increasing clinical relevance in several medical fields, most notably pathology. We show that using a cell-based assay combined with high throughput microscopy and CNN based analysis can be used to determine the activity of a wide set of mutations and potentially assist the prediction of targeted therapy outcome. Our results highlight the role of functional interpretation of molecular profiles, enabling more accurate prediction of oncogenic mutations.
更多查看译文
AI 理解论文
溯源树
样例
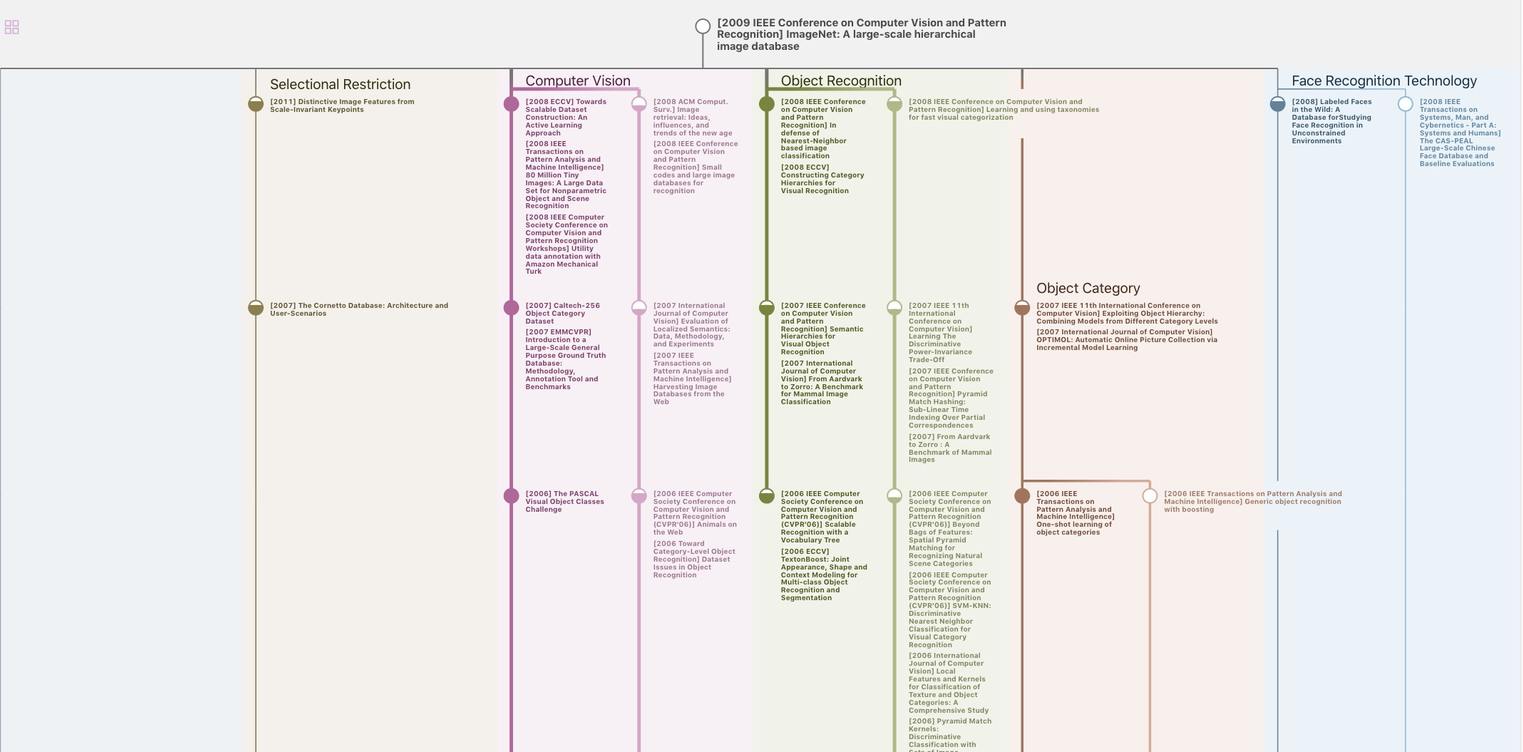
生成溯源树,研究论文发展脉络
Chat Paper
正在生成论文摘要