Noise-Robust Hyperspectral Image Classification via Multi-Scale Total Variation
IEEE Journal of Selected Topics in Applied Earth Observations and Remote Sensing(2019)
摘要
In this paper, a novel multi-scale total variation method is proposed to extract structural features from hyperspectral images (HSIs), which consists of the following steps. First, the spectral dimension of the HSI is reduced with an averaging-based method. Then, the multi-scale structural features (MSFs), which are insensitive to image noise, are constructed with a relative total variation-based structure extraction technique. Finally, the MSFs are fused together using kernel principal component analysis (KPCA), so as to obtain the KPCA-fused MSFs for classification. Experimental results on three publicly available hyperspectral datasets, including both well-known, long-used data, and a recent dataset obtained from an international contest, demonstrate the competitive performance over several state-of-the-art classification approaches in this field. Moreover, the robustness of the proposed method to the small-sample-size problem and serious image noise is also demonstrated.
更多查看译文
关键词
Feature extraction,Hyperspectral imaging,Kernel,Principal component analysis,Noise robustness
AI 理解论文
溯源树
样例
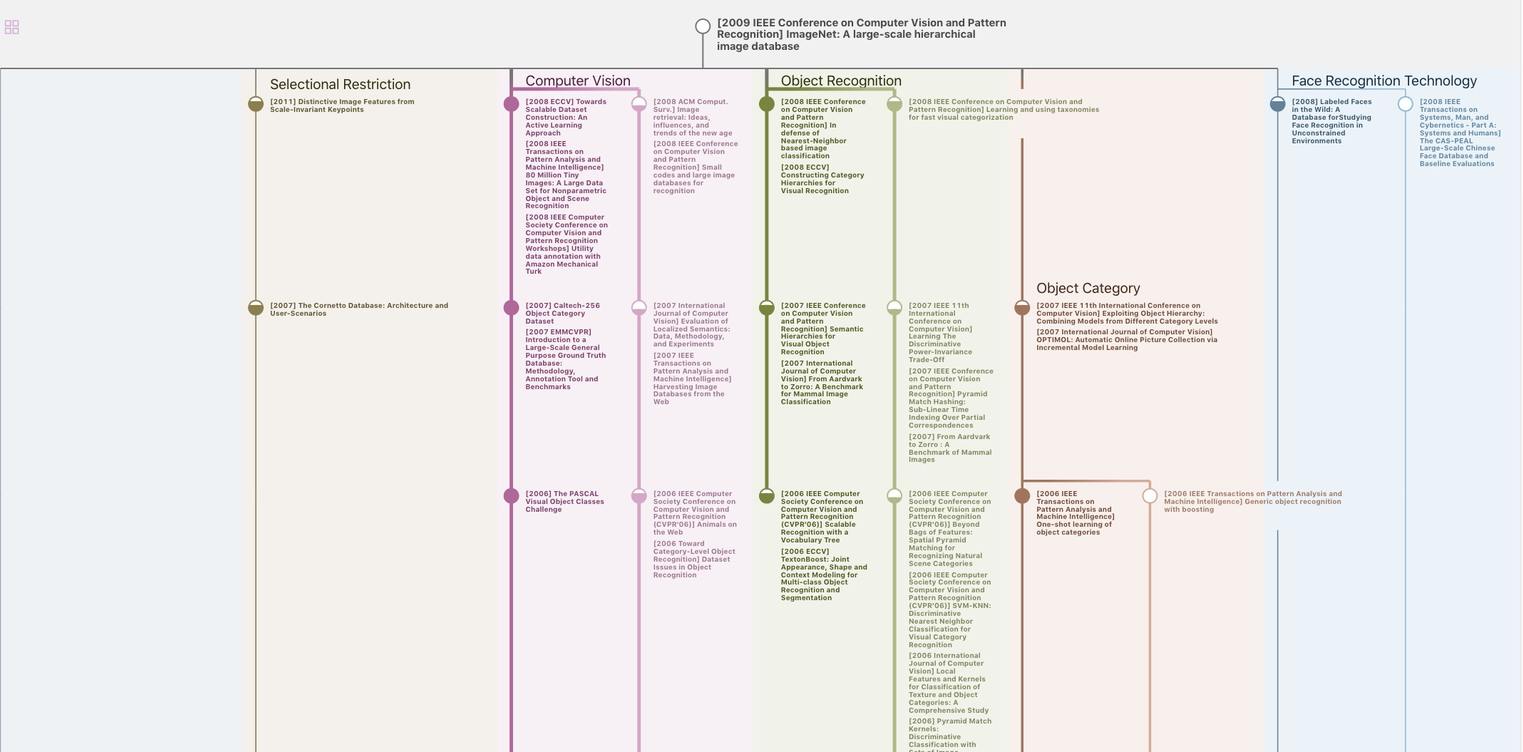
生成溯源树,研究论文发展脉络
Chat Paper
正在生成论文摘要