Illustration of the Flexibility of Generalized Gamma Distribution in Modeling Right Censored Survival Data: Analysis of Two Cancer Datasets
Annals of Data Science(2019)
摘要
In this paper, our main objective is to illustrate the flexibility of the wider class of generalized gamma distribution to model right censored survival data. This distribution contains the commonly used gamma, Weibull, and lognormal distributions as particular cases and this flexibility allows us to carry out a model discrimination, within its class, to choose a lifetime distribution that provides the best fit to a given data. A detailed Monte Carlo simulation study is carried out to display the flexibility of the generalized distribution using likelihood ratio test and information-based criteria. The maximum likelihood estimates of the parameters are obtained by using inbuilt optimization techniques available in R statistical software. We also display the performance of the estimation technique by calculating the bias, mean square error, and coverage probabilities of the confidence intervals for different confidence levels. Finally, we illustrate the advantage of using the generalized gamma distribution using two real datasets and we motivate the use of an extended version of the generalized gamma distribution.
更多查看译文
关键词
Maximum likelihood estimates, Mixture chi-square, Likelihood ratio test, Goodness-of-fit, Model discrimination, 62N02
AI 理解论文
溯源树
样例
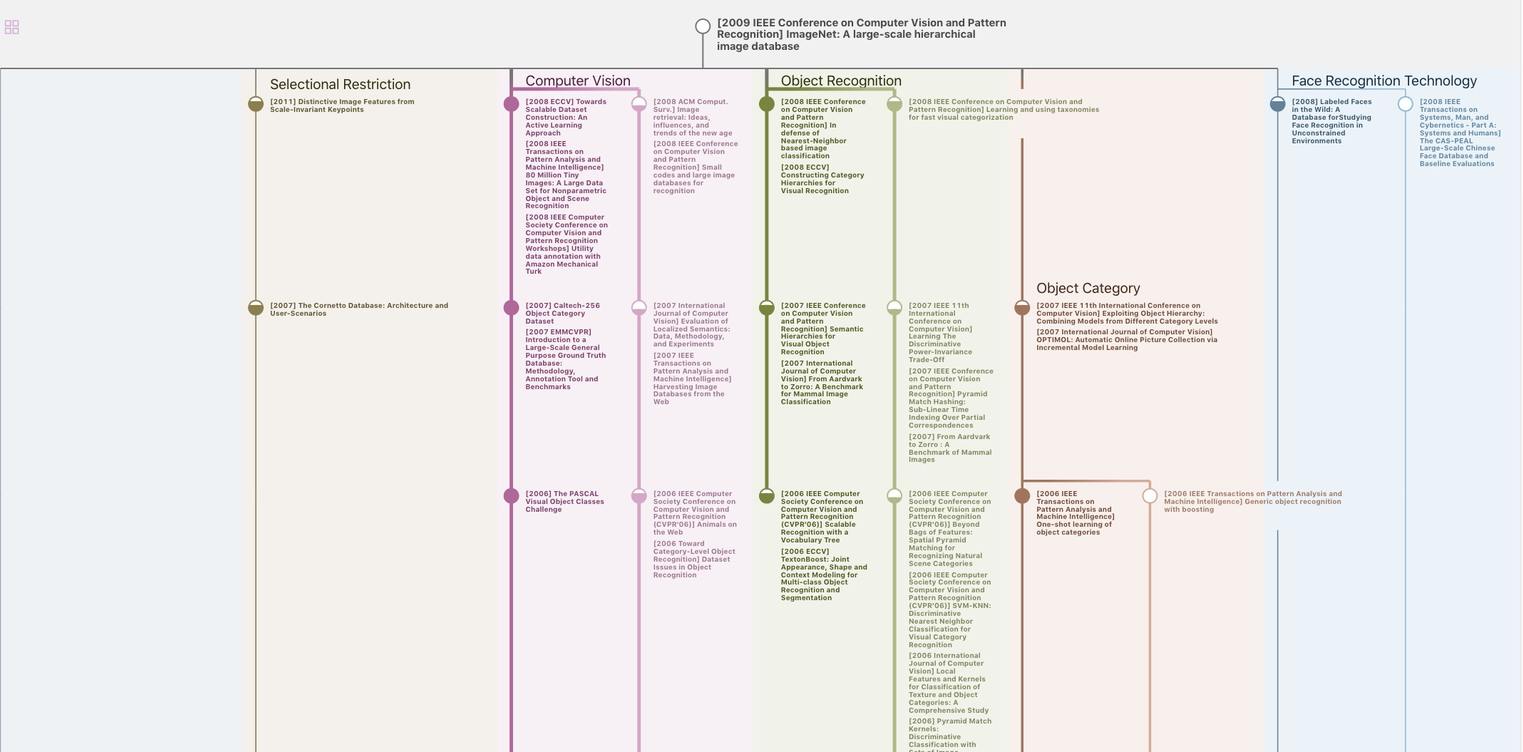
生成溯源树,研究论文发展脉络
Chat Paper
正在生成论文摘要