Deep Point-based Scene Labeling with Depth Mapping and Geometric Patch Feature Encoding
Graphical Models(2019)
摘要
This paper presents a deep CNN approach for point-based semantic scene labeling. This is challenging because 3D point clouds do not have a canonical domain and can have complex geometry and substantial variation of sampling densities. We propose a novel framework where the convolution operator is defined on depth maps around sampled points, which captures characteristics of local surface regions. We introduce Depth Mapping (DM) and Reverse Depth Mapping (RDM) operators to transform between the point domain and the depth map domain. Our depth map based convolution is computationally efficient, robust to scene scales and sampling densities, and can capture rich surface characteristics. We further propose to augment each point with feature encoding of the local geometric patches resulted from multi-method through patch pooling network (PPN). The patch features provide complementary information and are fed into our classification network to achieve semantic segmentation.
更多查看译文
关键词
Segmentation,Semantics labeling,CNN,Deep learning,Patch,Scene understanding
AI 理解论文
溯源树
样例
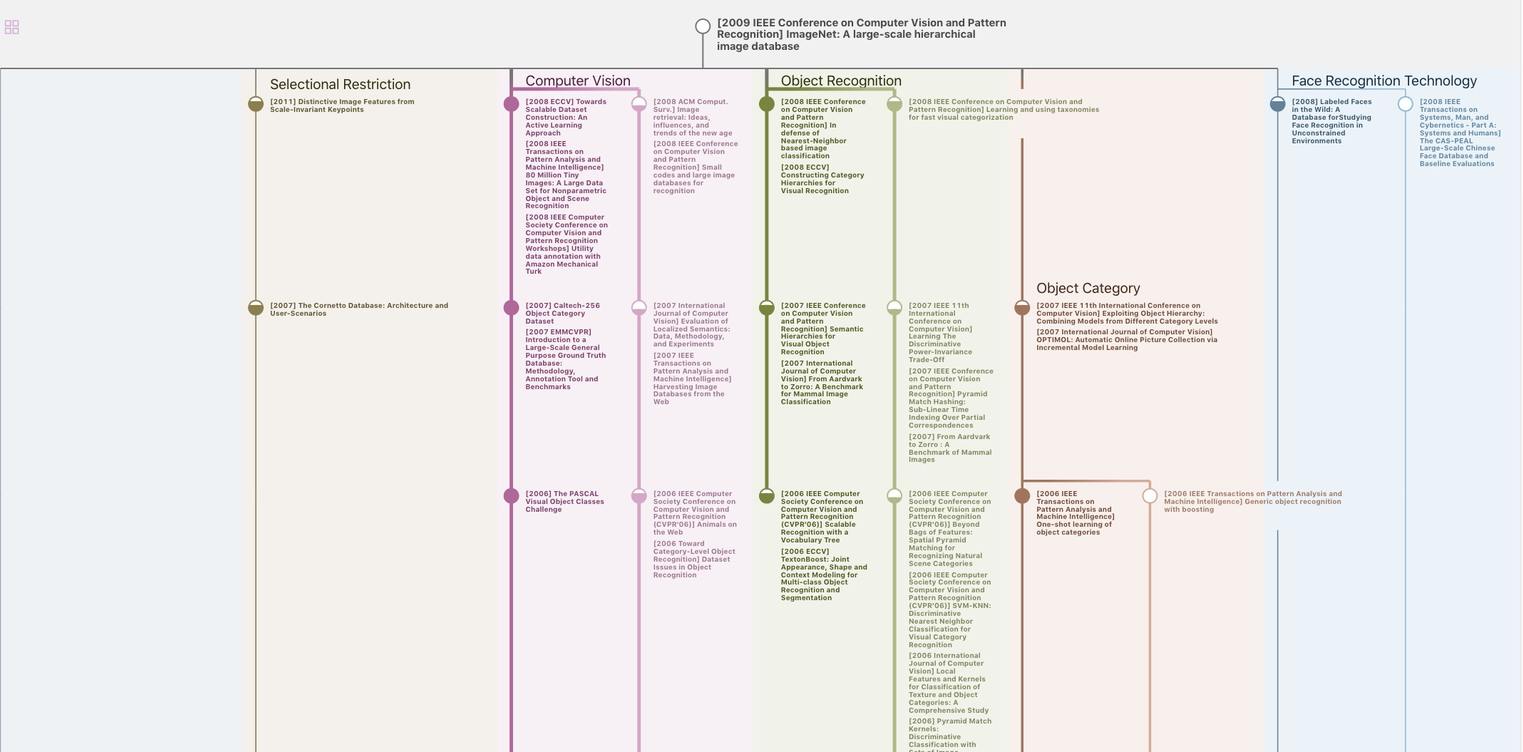
生成溯源树,研究论文发展脉络
Chat Paper
正在生成论文摘要