Ensemble of multi-objective metaheuristic algorithms for multi-objective unconstrained binary quadratic programming problem
Applied Soft Computing(2019)
摘要
Metaheuristics have been widely utilized for solving NP-hard optimization problems. However, these algorithms usually perform differently from one problem to another, i.e., one may be effective on a problem but performs badly on another problem. Therefore, it is difficult to choose the best algorithm in advance for a given problem. In contrast to selecting the best algorithm for a problem, selection hyper-heuristics aim at performing well on a set of problems (instances). This paper proposes a selection hyper-heuristic based algorithm for multi-objective optimization problems. In the proposed algorithm, multiple metaheuristics exhibiting different search behaviors are managed and controlled as low-level metaheuristics in an algorithm pool, and the most appropriate metaheuristic is selected by means of a performance indicator at each search stage. To assess the performance of the proposed algorithm, an implementation of the algorithm containing four metaheuristics is proposed and tested for solving multi-objective unconstrained binary quadratic programming problem. Experimental results on 50 benchmark instances show that the proposed algorithm can provide better overall performance than single metaheuristics, which demonstrates the effectiveness of the proposed algorithm.
更多查看译文
关键词
Multi-objective optimization,Selection hyper-heuristic,Multi-objective unconstrained binary quadratic programming problem
AI 理解论文
溯源树
样例
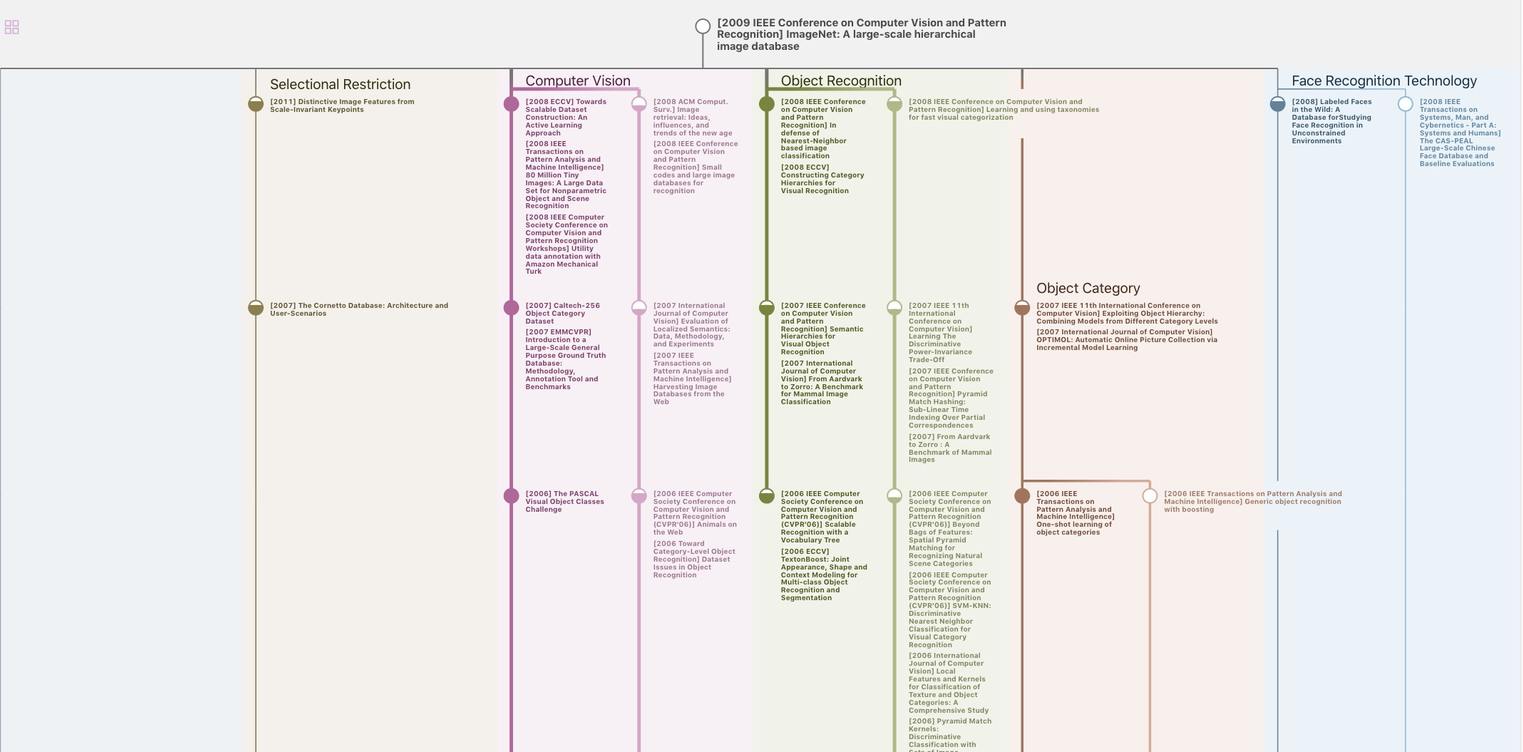
生成溯源树,研究论文发展脉络
Chat Paper
正在生成论文摘要