Study on novel signal processing and simultaneous-fault diagnostic method for wind turbine:
TRANSACTIONS OF THE INSTITUTE OF MEASUREMENT AND CONTROL(2019)
摘要
High-precision fault diagnosis is important for the widely installed complex industrial product, the wind turbine. However, intelligent monitoring is difficult due to the fuzzy boundaries and individual different variations of the unseen single or simultaneous-fault of such intricate equipment. To solve this problem, this study proposes an ensemble fault diagnostic framework for simultaneous and coupling failure. First, this paper develops novel signal processing methods for effective feature learning and mapping from the non-stationary and nonlinear raw vibrational signals. The adapted variational mode decomposition is introduced based on the particle swarm optimization that applies the minimum mean envelope entropy to optimize the parameters settings. Second, the novel ensemble extreme learning machine-based network is proposed to isolate the faults that applies one extreme learning machine network to count the number of fault scenarios, and the other one to identify the specific single or simultaneous-fault labels. With this scheme, the self-adaptive ensemble extreme learning machine-based fault diagnostic framework is more accurate and faster than the prevailing probabilistic classifier-based methods, as the proposed method does not rely on empirically specified decision-making threshold and generates all the candidate fault labels at the same time. Finally, this study builds the test platform and compares the overall results with the existing feature analysis methods and classifiers. The experimental results verify that the proposed framework detects both single and simultaneous-fault accurately and quickly.
更多查看译文
关键词
Variational mode decomposition,empirical mode decomposition,extreme learning machines,fault diagnosis,wind turbine
AI 理解论文
溯源树
样例
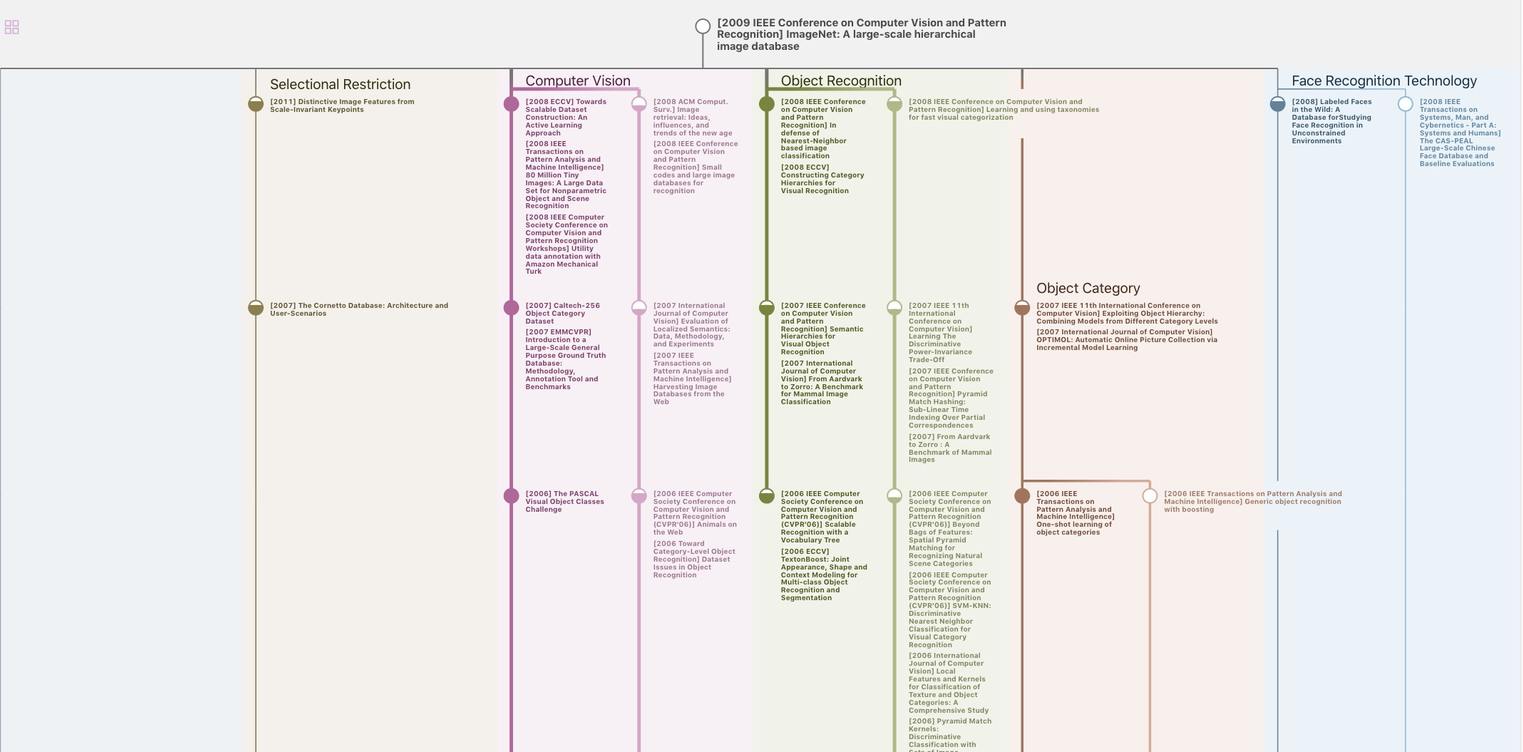
生成溯源树,研究论文发展脉络
Chat Paper
正在生成论文摘要