The space decomposition method for the sum of nonlinear convex maximum eigenvalues and its applications
JOURNAL OF INDUSTRIAL AND MANAGEMENT OPTIMIZATION(2020)
摘要
In this paper, we mainly consider optimization problems involving the sum of largest eigenvalues of nonlinear symmetric matrices. One of the difficulties with numerical analysis of such problems is that the eigenvalues, regarded as functions of a symmetric matrix, are not differentiable at those points where they coalesce. The U-Lagrangian theory is applied to the function of the sum of the largest eigenvalues, with convex matrix-valued mappings, which doesn't need to be affine. Some of the results generalize the corresponding conclusions for linear mapping. In the approach, we reformulate the first- and second-order derivatives of U-Lagrangian in the space of decision variables R-m under some mild conditions in terms of VU-space decomposition. We characterize smooth trajectory, along which the function has a second-order expansion. Moreover, an algorithm framework with superlinear convergence is presented. Finally, an application of VU-decomposition derivatives shows that U-Lagrangian possesses proper execution in matrix variable.
更多查看译文
关键词
Eigenvalue optimization,matrix-convex,nonsmooth optimization,VU-decomposition,second-order derivative
AI 理解论文
溯源树
样例
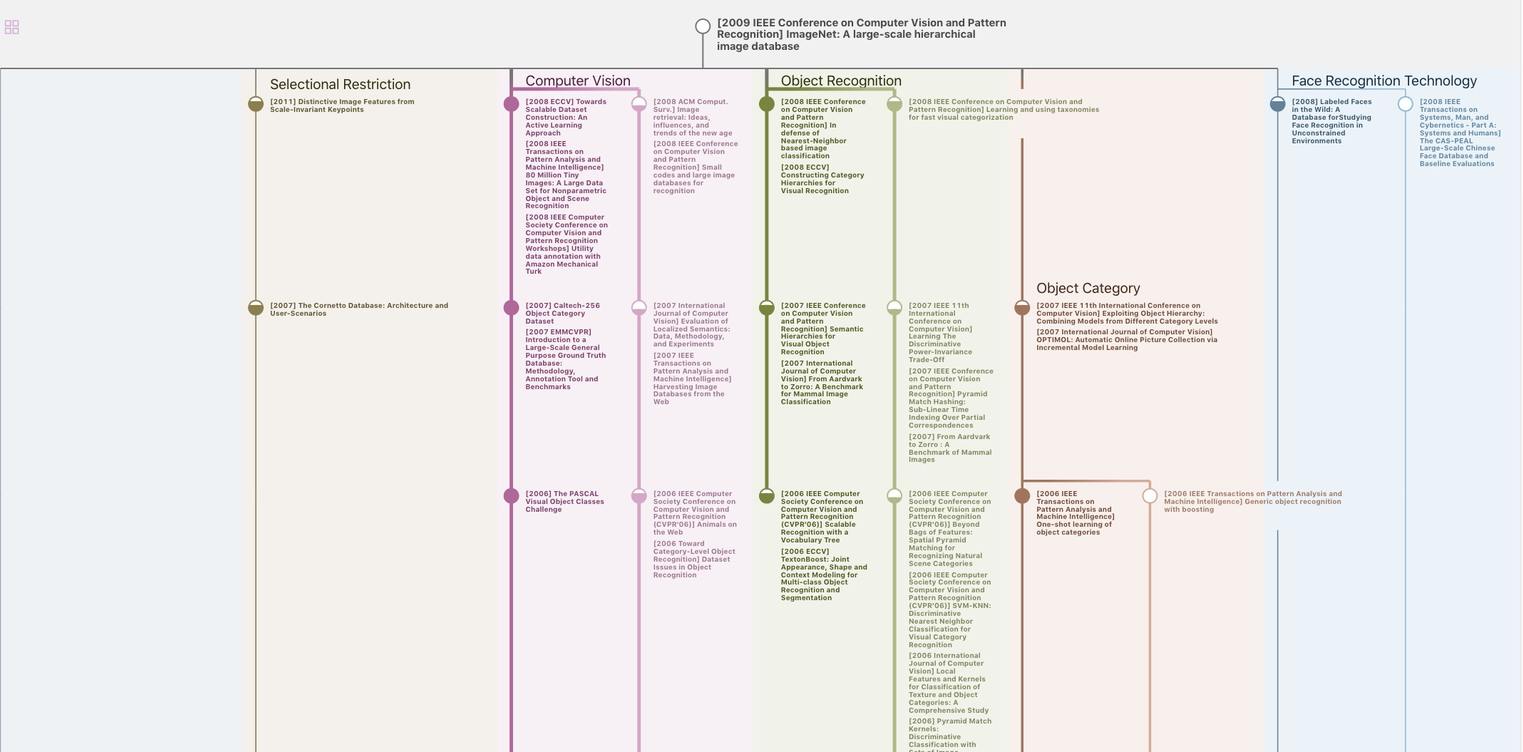
生成溯源树,研究论文发展脉络
Chat Paper
正在生成论文摘要