On the use of approximate Bayesian computation Markov chain Monte Carlo with inflated tolerance and post-correction
BIOMETRIKA(2020)
摘要
Approximate Bayesian computation enables inference for complicated probabilistic models with intractable likelihoods using model simulations. The Markov chain Monte Carlo implementation of approximate Bayesian computation is often sensitive to the tolerance parameter: low tolerance leads to poor mixing and large tolerance entails excess bias. We propose an approach that involves using a relatively large tolerance for the Markov chain Monte Carlo sampler to ensure sufficient mixing and post-processing the output, leading to estimators for a range of finer tolerances. We introduce an approximate confidence interval for the related post-corrected estimators and propose an adaptive approximate Bayesian computation Markov chain Monte Carlo algorithm, which finds a balanced tolerance level automatically based on acceptance rate optimization. Our experiments show that post-processing-based estimators can perform better than direct Markov chain Monte Carlo targeting a fine tolerance, that our confidence intervals are reliable, and that our adaptive algorithm leads to reliable inference with little user specification.
更多查看译文
关键词
Adaptive algorithm,Approximate Bayesian computation,Confidence interval,Importance sampling,Markov chain Monte Carlo,Tolerance choice
AI 理解论文
溯源树
样例
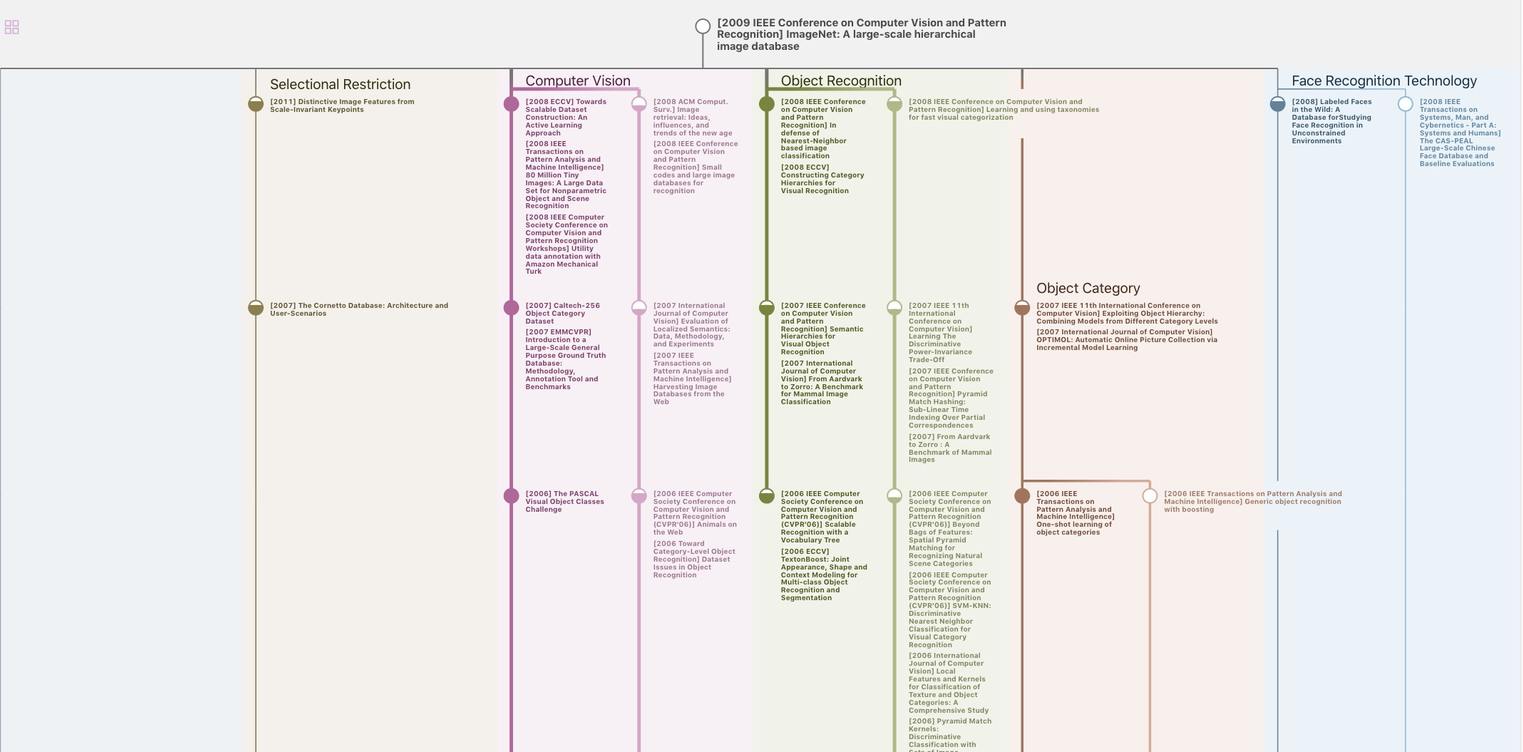
生成溯源树,研究论文发展脉络
Chat Paper
正在生成论文摘要