Feature Extraction From The Hermitian Manifold For Brain-Computer Interfaces
2019 9TH INTERNATIONAL IEEE/EMBS CONFERENCE ON NEURAL ENGINEERING (NER)(2019)
摘要
Riemannian geometry-based methods have shown to be effective in many sorts of Brain-Computer Interface (BCI) applications, but are only capable of measuring the power of the measured signal. This paper proposes a set of novel features derived via the Hilbert transform and applies them to the generalized Riemannian manifold, the Hermitian manifold, to see whether the classification accuracy benefits from this treatment. To validate these features, we benchmark them with the Mother of All BCI Benchmarks framework, a recently introduced tool to make BCI methods research more reproducible. The results indicate that in some settings the analytic covariance matrix can improve BCI performance.
更多查看译文
关键词
classification accuracy benefits,BCI methods research,feature extraction,Hermitian manifold,Riemannian geometry-based methods,measured signal,generalized Riemannian manifold,brain-computer interface applications,BCI benchmarks framework
AI 理解论文
溯源树
样例
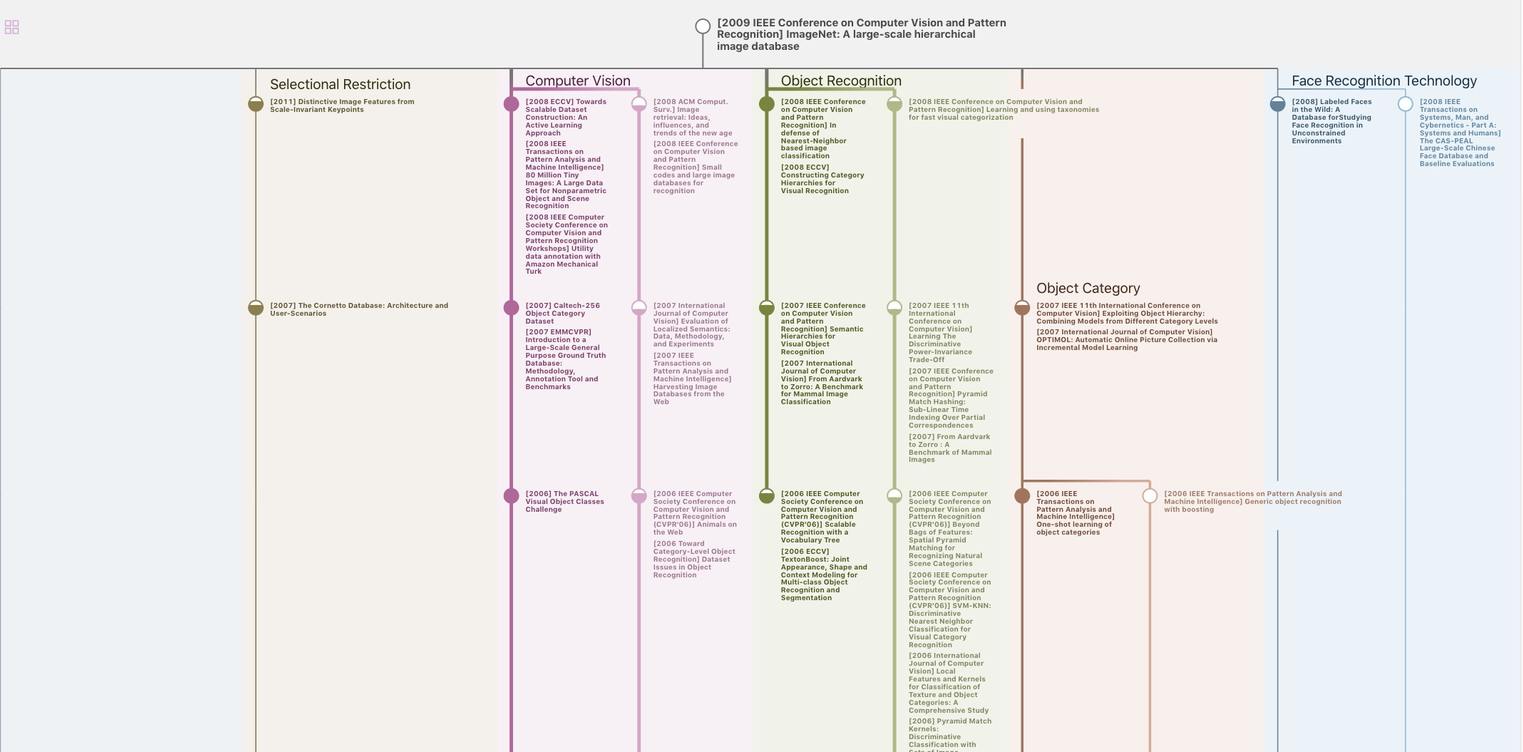
生成溯源树,研究论文发展脉络
Chat Paper
正在生成论文摘要