Compressing Convolutional Neural Networks Via Factorized Convolutional Filters
2019 IEEE/CVF CONFERENCE ON COMPUTER VISION AND PATTERN RECOGNITION (CVPR 2019)(2019)
摘要
This work studies the model compression for deep convolutional neural networks (CNNs) via filter pruning. The workflow of a traditional pruning consists of three sequential stages: pre-training the original model, selecting the pre-trained filters via ranking according to a manually designed criterion (e.g., the norm of filters), and learning the remained filters via fine-tuning. Most existing works follow this pipeline and focus on designing different ranking criteria for filter selection. However, it is difficult to control the performance due to the separation of filter selection and filter learning. In this work, we propose to conduct filter selection and filter learning simultaneously, in a unified model. To this end, we define a factorized convolutional filter (FCF), consisting of a standard real-valued convolutional filter and a binary scalar, as well as a dot product-operator between them. We train a CNN model with factorized convolutional filters (CNN-FCF) by updating the standard filter using back-propagation, while updating the binary scalar using the alternating direction method of multipliers (ADMM) based optimization method. With this trained CNN-FCF model, we only keep the standard filters corresponding to the 1-valued scalars, while all other filters and all binary scalars are discarded, to obtain a compact CNN model. Extensive experiments on CIFAR-I0 and ImageNet demonstrate the superiority of the proposed method over state-of-the-art filter pruning methods.
更多查看译文
关键词
Representation Learning,Deep Learning
AI 理解论文
溯源树
样例
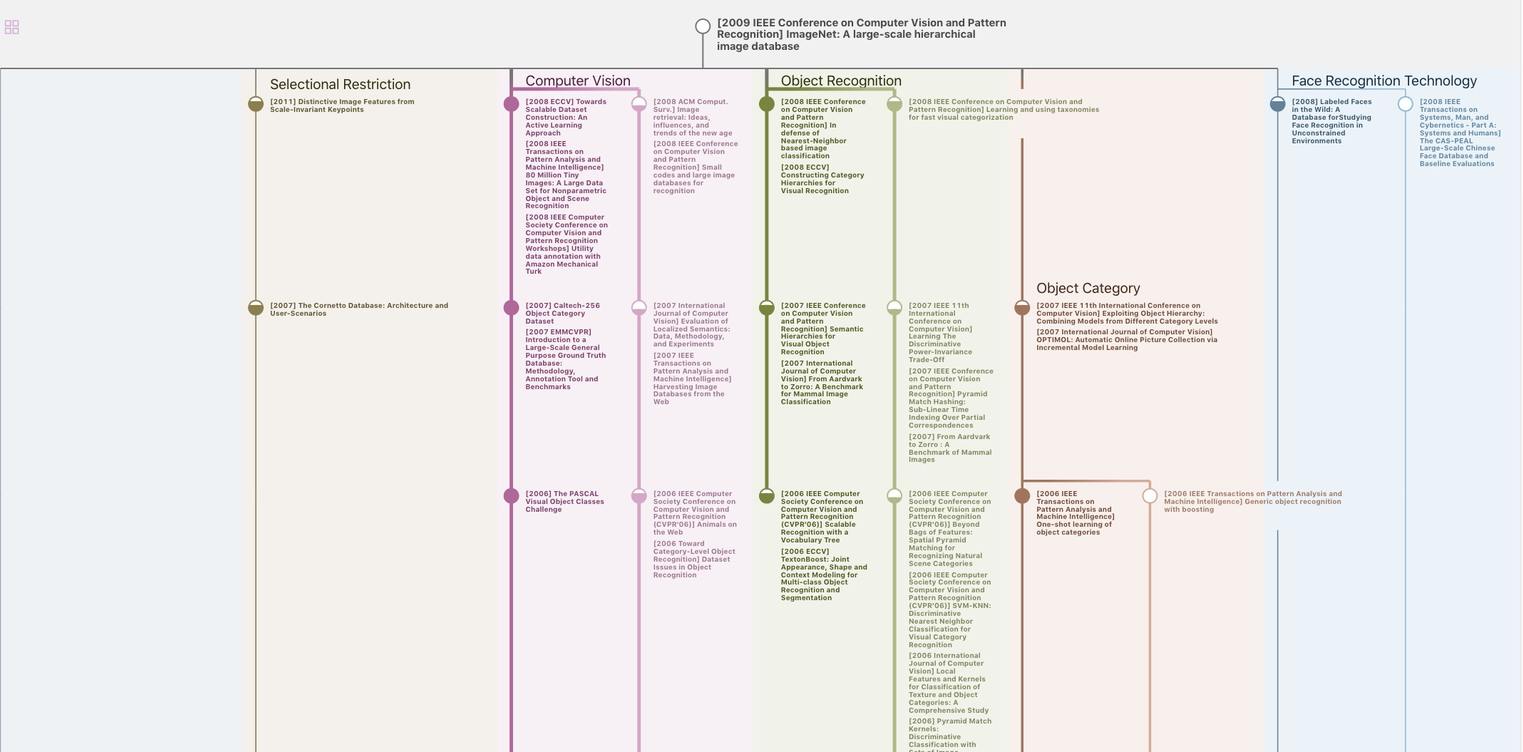
生成溯源树,研究论文发展脉络
Chat Paper
正在生成论文摘要