Feature Extraction For Predicting The Probability Of Detecting Buried Explosive Objects Using Gpr Data
DETECTION AND SENSING OF MINES, EXPLOSIVE OBJECTS, AND OBSCURED TARGETS XXIV(2019)
摘要
Detection of buried explosive objects has been studied extensively and several sensors have been developed. In particular, ground penetrating radar (GPR) has proved to be one of the most successful modalities and many machine learning algorithms have been developed for buried threat detection using this sensor. Large scale experiments that involved multiple detection algorithms and very large data collections have indicated that the relative performance of different algorithms can vary significantly depending on the explosive objects, geographical site, soil and weather conditions, and burial depth. In fact, it is possible for an algorithm that performs well on training data to have low probability of target detection (PD), or high false alarm rate (FAR), on new data collected in a different environment. In this paper, we investigate the possibility of developing an algorithm that can predict the performance of a discrimination algorithm on GPR data collected in different environments. This can be used to select the optimal sensor/algorithm for a given location. It can also be used to select the optimal parameters of a given discriminator for a given site. Our approach combines predictive analysis with adequate feature selection methods to boost PD modeling and improve its prediction accuracy. Starting from raw GPR data, we extract and investigate a large set of potential descriptors that can quantify noise, surface roughness, and (implicit) soil properties. Our objectives are to: (i) Identify the optimal subset of features that can affect the target PDs of a given discriminator; and (ii) Learn a regression model for PD prediction. To validate our approach, we use data collected by a GPR sensor mounted on a vehicle. We extract over 50 different features from background regions and investigate feature selection and regression algorithms to learn a model that can predict the targets PD of a given discrimination algorithm for a given lane segment. We validate our results using different cross-validation methods.
更多查看译文
AI 理解论文
溯源树
样例
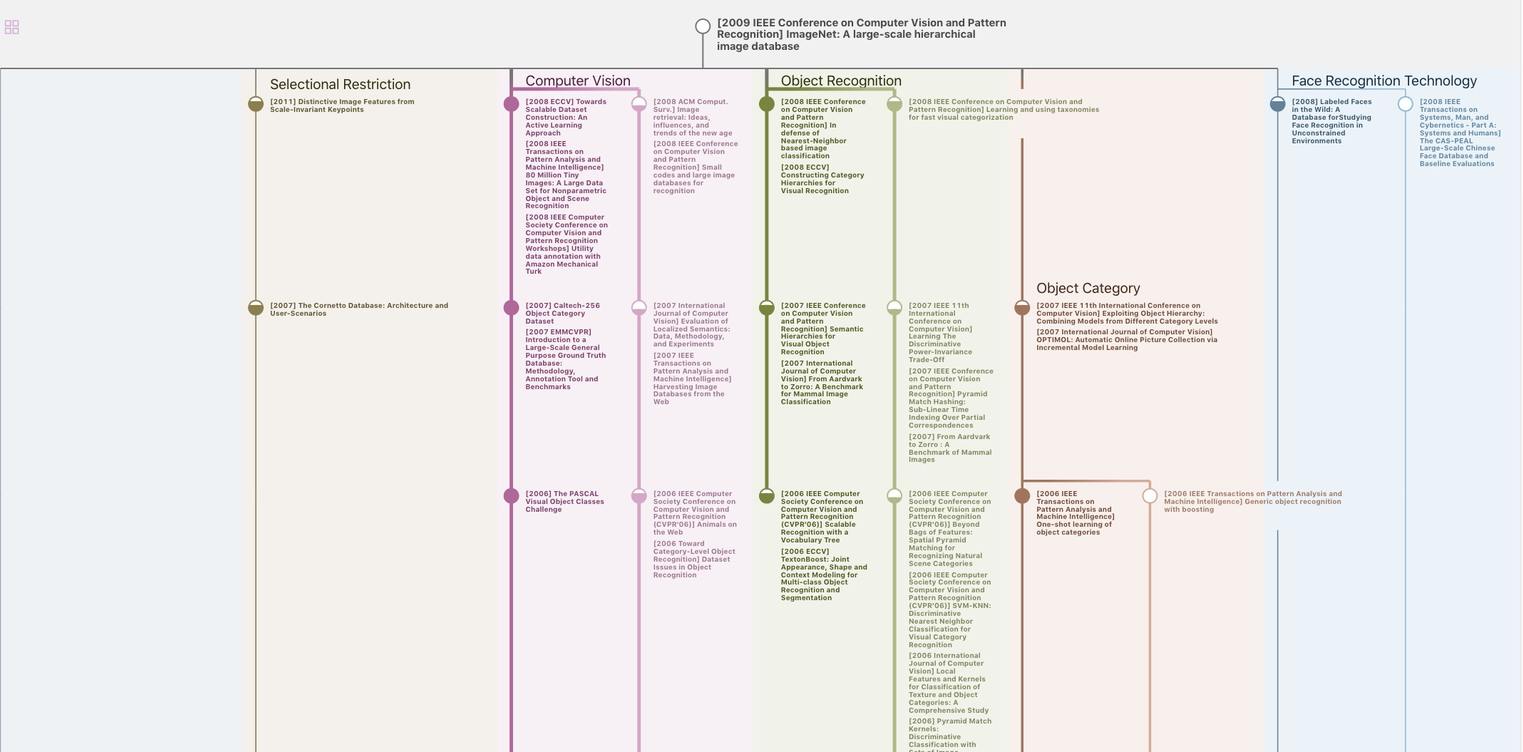
生成溯源树,研究论文发展脉络
Chat Paper
正在生成论文摘要