Recommending Products When Consumers Learn Their Preference Weights
MARKETING SCIENCE(2019)
摘要
Consumers often learn the weights they ascribe to product attributes ("preference weights") as they search. For example, after test driving cars, a consumer might find that he or she undervalued trunk space and overvalued sunroofs. Preference-weight learning makes optimal search complex because each time a product is searched, updated preference weights affect the expected utility of all products and the value of subsequent optimal search. Product recommendations, which take preference-weight learning into account, help consumers search. We motivate a model in which consumers learn (update) their preference weights. When consumers learn preference weights, it may not be optimal to recommend the product with the highest option value, as in most search models, or the product most likely to be chosen, as in traditional recommendation systems. Recommendations are improved if consumers are encouraged to search products with diverse attribute levels, products that are undervalued, or products for which recommendation-system priors differ from consumers' priors. Synthetic data experiments demonstrate that proposed recommendation systems outperform benchmark recommendation systems, especially when consumers are novices and when recommendation systems have good priors. We demonstrate empirically that consumers learn preference weights during search, that recommendation systems can predict changes, and that a proposed recommendation system encourages learning.
更多查看译文
关键词
recommendation systems,learned preferences,multiattribute utility,consumer search
AI 理解论文
溯源树
样例
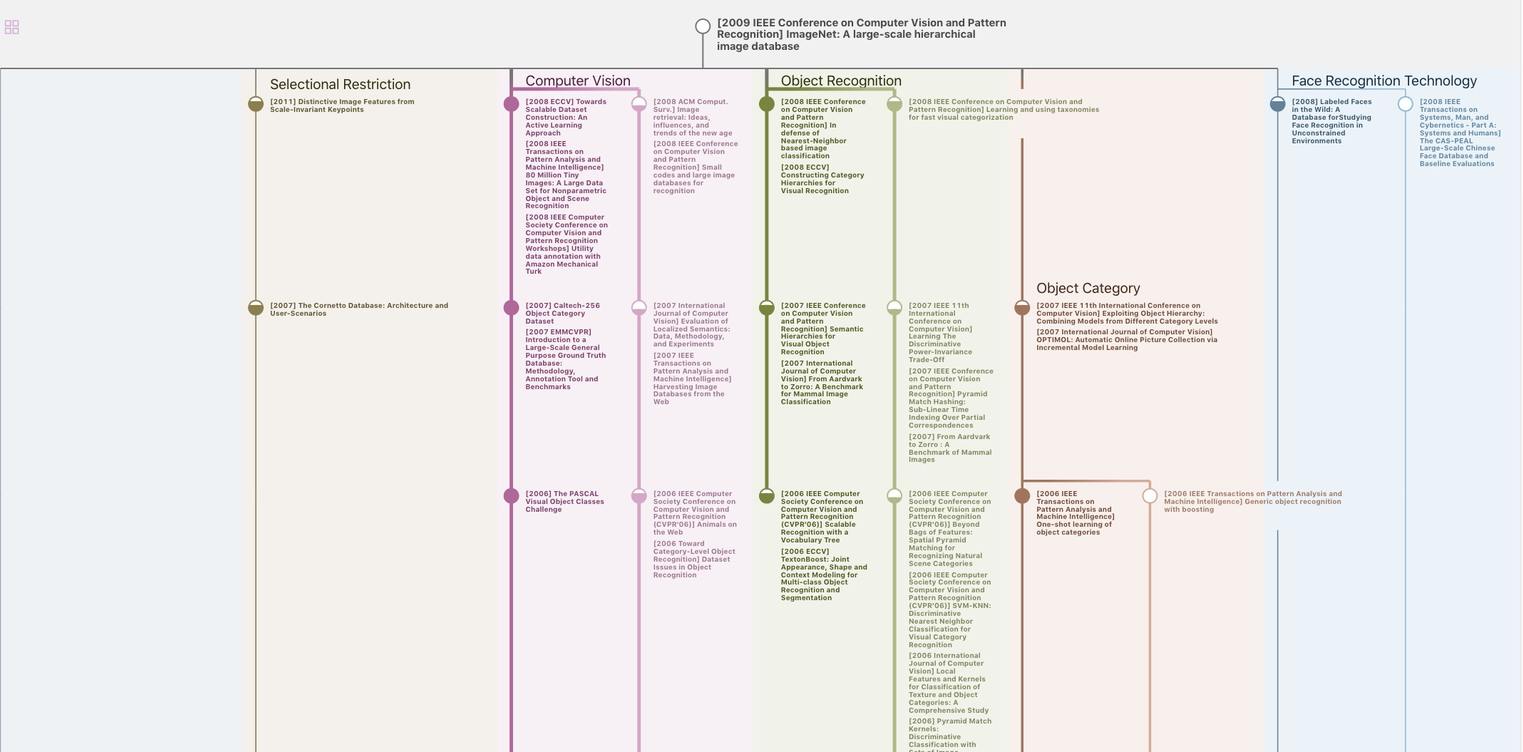
生成溯源树,研究论文发展脉络
Chat Paper
正在生成论文摘要