An advanced spatio-temporal model for particulate matter and gaseous pollutants in Beijing, China
Atmospheric Environment(2019)
摘要
Modeling fine-scale spatial and temporal patterns of air pollutants can be challenging. Advanced spatio-temporal modeling methods were used to predict both long-term and short-term concentrations of six criteria air pollutants (particulate matter with aerodynamic diameter less than or equal to 10 and 2.5 μm [PM10 and PM2.5], SO2, NO2, ozone and carbon monoxide [CO]) in Beijing, China. Monitoring data for the six criteria pollutants from April 2014 through December 2017 were obtained from 23 administrative monitoring sites in Beijing. The dimensions of a large array of geographic covariates were reduced using partial least squares (PLS) regression. A land use regression (LUR) model in a universal kriging framework was used to estimate pollutant concentrations over space and time. Prediction ability of the models was determined using leave-one-out cross-validation (LOOCV). Prediction accuracy of the spatio-temporal two-week averages was excellent for all of the pollutants, with LOOCV mean squared error-based R2 (R2mse) of 0.86, 0.95, 0.90, 0.82, 0.94 and 0.95 for PM10, PM2.5, SO2, NO2, ozone and CO, respectively. These models find ready application in making fine-scale exposure predictions for members of cohort health studies and may reduce exposure measurement error relative to other modeling approaches.
更多查看译文
关键词
Particulate matter,Air pollution,Spatio-temporal model,Geo-statistical model,Beijing
AI 理解论文
溯源树
样例
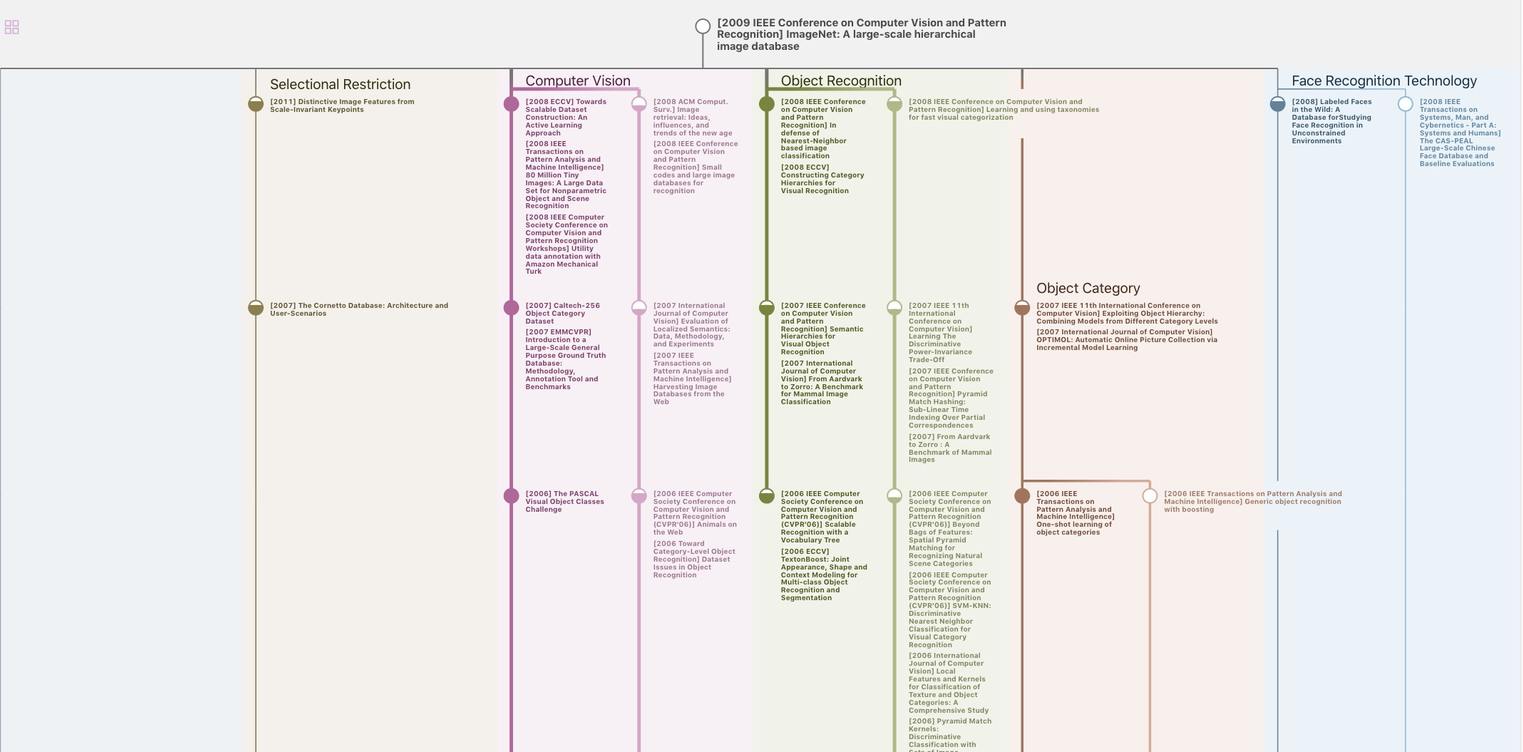
生成溯源树,研究论文发展脉络
Chat Paper
正在生成论文摘要