An extended DMP framework for robot learning and improving variable stiffness manipulation
ASSEMBLY AUTOMATION(2020)
摘要
Purpose The purpose of this paper is to present a method which enables a robot to learn both motion skills and stiffness profiles from humans through kinesthetic human-robot cooperation. Design Methodology Approach Admittance control is applied to allow robot-compliant behaviors when following the reference trajectories. By extending the dynamical movement primitives (DMP) model, a new concept of DMP and stiffness primitives is introduced to encode a kinesthetic demonstration as a combination of trajectories and stiffness profiles, which are subsequently transferred to the robot. Electromyographic signals are extracted from a human's upper limbs to obtain target stiffness profiles. By monitoring vibrations of the end-effector velocities, a stability observer is developed. The virtual damping coefficient of admittance controller is adjusted accordingly to eliminate the vibrations. Findings The performance of the proposed methods is evaluated experimentally. The result shows that the robot can perform tasks in a variable stiffness mode as like the human dose in the teaching phase. Originality Value DMP has been widely used as a teaching by demonstration method to represent movements of humans and robots. The proposed method extends the DMP framework to allow a robot to learn not only motion skills but also stiffness profiles. Additionally, the authors proposed a stability observer to eliminate vibrations when the robot is disturbed by environment.
更多查看译文
关键词
Robotics,Path planning,Man machine interface (MMI),Cooperative robots
AI 理解论文
溯源树
样例
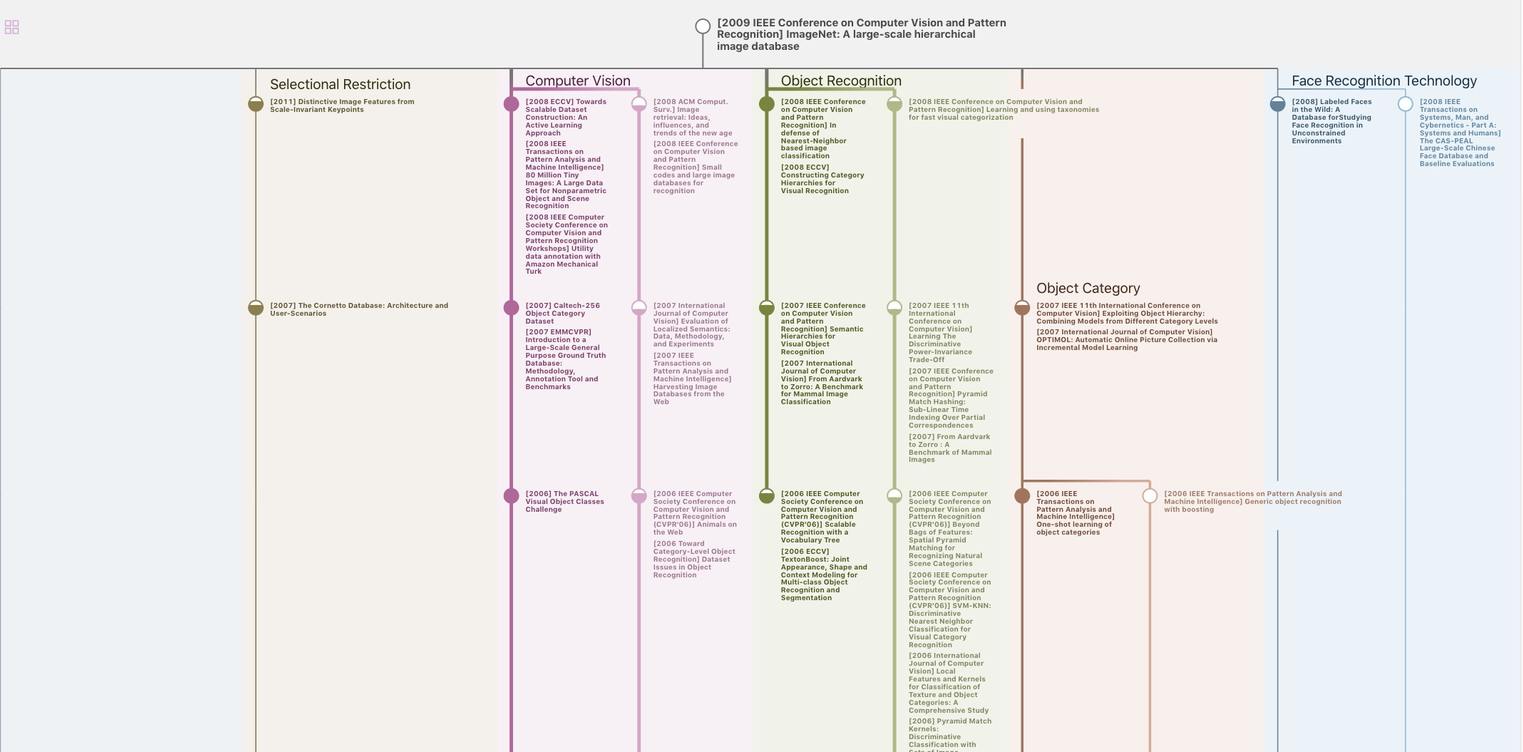
生成溯源树,研究论文发展脉络
Chat Paper
正在生成论文摘要