ANMAT: Automatic Knowledge Discovery and Error Detection through Pattern Functional Dependencies
Proceedings of the 2019 International Conference on Management of Data(2019)
摘要
Knowledge discovery is critical to successful data analytics. We propose a new type of meta-knowledge, namely pattern functional dependencies (PFDs), that combine patterns (or regex-like rules) and integrity constraints (ICs) to model the dependencies (or meta-knowledge) between partial values (or patterns) across different attributes in a table. PFDs go beyond the classical functional dependencies and their extensions. For instance, in an employee table, ID "F-9-107'', "F'' determines the finance department. Moreover, a key application of PFDs is to use them to identify erroneous data; tuples that violate some PFDs. In this demonstration, attendees will experience the following features: (i) PFD discovery -- automatically discover PFDs from (dirty) data in different domains; and (ii) Error detection with PFDs -- we will show errors that are detected by PFDs but cannot be captured by existing approaches.
更多查看译文
关键词
error detection, knowledge discovery, pattern functional dependencies
AI 理解论文
溯源树
样例
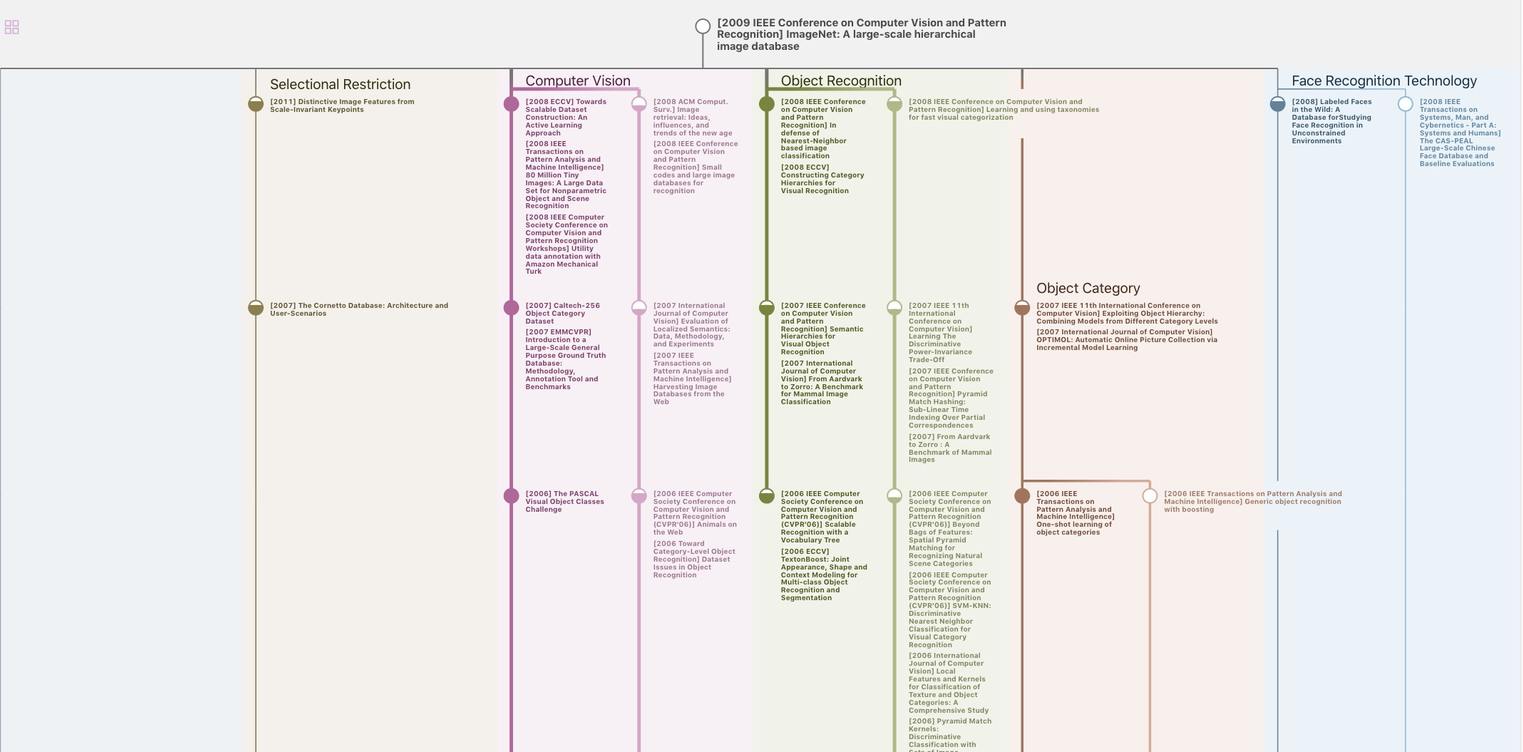
生成溯源树,研究论文发展脉络
Chat Paper
正在生成论文摘要