Nlh: A Blind Pixel-Level Non-Local Method For Real-World Image Denoising
IEEE TRANSACTIONS ON IMAGE PROCESSING(2020)
摘要
Non-local self similarity (NSS) is a powerful prior of natural images for image denoising. Most of existing denoising methods employ similar patches, which is a patch-level NSS prior. In this paper, we take one step forward by introducing a pixel-level NSS prior, i.e., searching similar pixels across a non-local region. This is motivated by the fact that finding closely similar pixels is more feasible than similar patches in natural images, which can be used to enhance image denoising performance. With the introduced pixel-level NSS prior, we propose an accurate noise level estimation method, and then develop a blind image denoising method based on the lifting Haar transform and Wiener filtering techniques. Experiments on benchmark datasets demonstrate that, the proposed method achieves much better performance than previous non-deep methods, and is still competitive with existing state-of-the-art deep learning based methods on real-world image denoising. The code is publicly available at https://github.com/njusthyk1972/NLH.
更多查看译文
关键词
Non-local self similarity,pixel-level similarity,image denoising
AI 理解论文
溯源树
样例
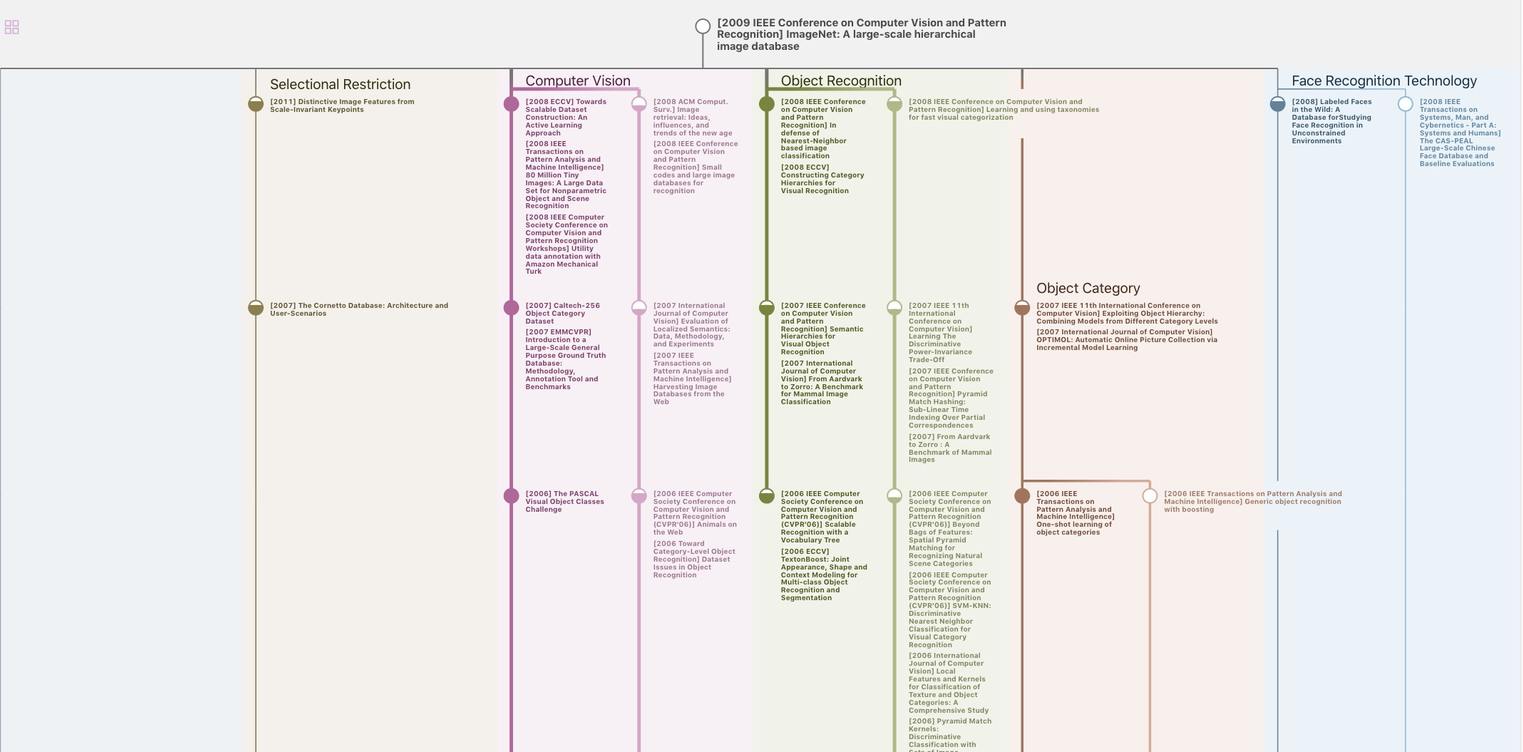
生成溯源树,研究论文发展脉络
Chat Paper
正在生成论文摘要