IMP: Instance Mask Projection for High Accuracy Semantic Segmentation of Things
2019 IEEE/CVF INTERNATIONAL CONFERENCE ON COMPUTER VISION (ICCV 2019)(2019)
摘要
In this work, we present a new operator, called Instance Mask Projection (IMP), which projects a predicted instance segmentation as a new feature for semantic segmentation. It also supports back propagation and is trainable end-to-end. By adding this operator, we introduce a new way to combine top-down and bottom-up information in semantic segmentation. Our experiments show the effectiveness of IMP on both clothing parsing (with complex layering, large deformations, and non-convex objects), and on street scene segmentation (with many overlapping instances and small objects). On the Varied Clothing Parsing dataset (VCP), we show instance mask projection can improve mIOU by 3 points over a state-of-the-art Panoptic FPN segmentation approach. On the ModaNet clothing parsing dataset, we show a dramatic improvement of 20.4% compared to existing baseline semantic segmentation results. In addition, the Instance Mask Projection operator works well on other (non-clothing) datasets, providing an improvement in mIOU of 3 points on "thing" classes of Cityscapes, a self-driving dataset, over a state-of-the-art approach.
更多查看译文
关键词
backpropagation,baseline semantic segmentation results,panoptic FPN segmentation approach,instance segmentation,instance mask projection,ModaNet clothing parsing dataset,Varied Clothing Parsing dataset,street scene segmentation,nonconvex objects,high accuracy semantic segmentation,IMP
AI 理解论文
溯源树
样例
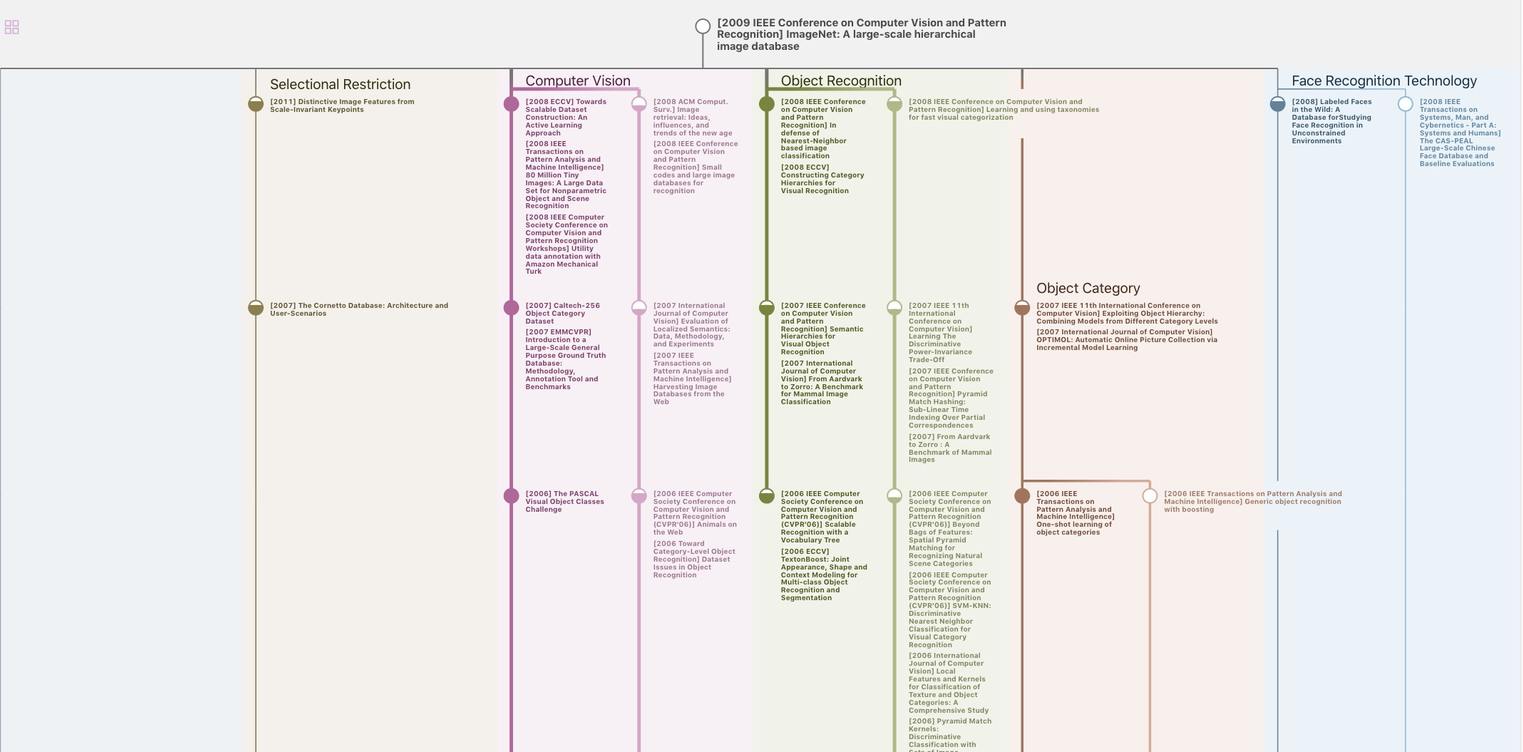
生成溯源树,研究论文发展脉络
Chat Paper
正在生成论文摘要