Quantification of In Vivo Target Engagement Using Microfluidic Activity-Based Protein Profiling.
SLAS TECHNOLOGY(2019)
摘要
Accurate measurement of drug-target interactions in vivo is critical for both preclinical development and translation to clinical studies, yet many assays rely on indirect measures such as biomarkers associated with target activity. Activity-based protein profiling (ABPP) is a direct method of quantifying enzyme activity using active site-targeted small-molecule covalent probes that selectively label active but not inhibitor-bound enzymes. Probe-labeled enzymes in complex proteomes are separated by polyacrylamide gel electrophoresis and quantified by fluorescence imaging. To accelerate workflows and avoid imaging artifacts that make conventional gels challenging to quantify, we adapted protocols for a commercial LabChip GXII microfluidic instrument to permit electrophoretic separation of probe-labeled proteins in tissue lysates and plasma, and quantification of fluorescence (probe/protein labeling ratio of 1:1). Electrophoretic separation on chips occurred in 40 s per sample, and instrument software automatically identified and quantified peaks, resulting in an overall time savings of 3-5 h per 96-well sample plate. Calculated percent inhibition was not significantly different between the two formats. Chip performance was consistent between chips and sample replicates. Conventional gel imaging was more sensitive but required five times higher sample volume than microfluidic chips. Microfluidic chips produced results comparable to those of gels but with much lower sample consumption, facilitating assay miniaturization for scarce biological samples. The time savings afforded by microfluidic electrophoresis and automatic quantification has allowed us to incorporate microfluidic ABPP early in the drug discovery workflow, enabling routine assessments of tissue distribution and engagement of targets and off-targets in vivo.
更多查看译文
关键词
ABPP,LabChip,protein chip,monoacylglycerol lipase,MGLL
AI 理解论文
溯源树
样例
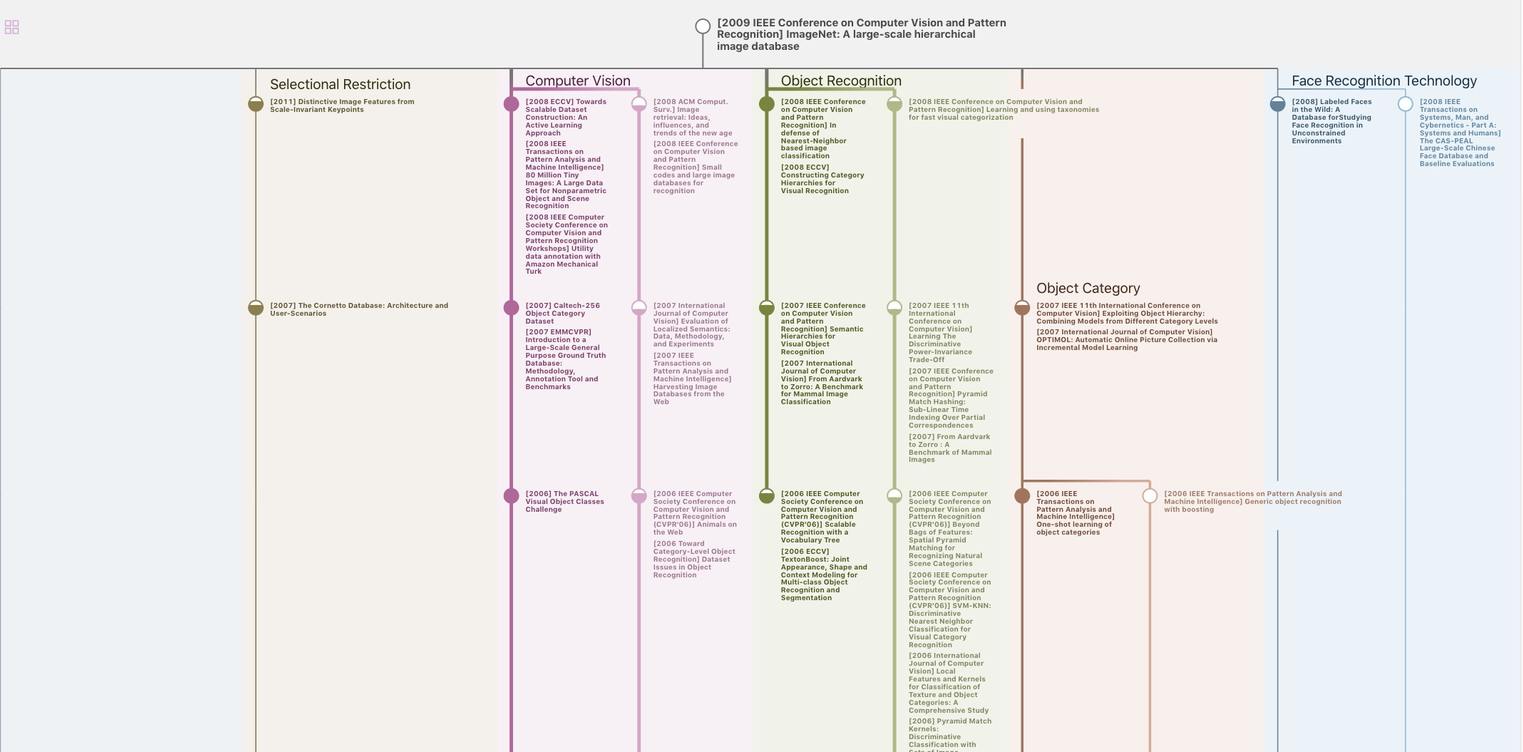
生成溯源树,研究论文发展脉络
Chat Paper
正在生成论文摘要