MMAN: Multi-modality aggregation network for brain segmentation from MR images.
Neurocomputing(2019)
摘要
Brain tissue segmentation from Magnetic resonance (MR) image is significant for assessing both neurologic conditions and brain disease. Manual brain tissue segmentation is time-consuming, tedious and subjective which indicates a need for more efficiently automated approaches. However, due to ambiguous boundaries, anatomically complex structure and individual differences, conventional automated segmentation methods performed poorly. Therefore, more effective feature extraction techniques and advanced segmentation models are in essential demand. Inspired by deep learning concepts, we propose a multi-modality aggregation network (MMAN), which is able to extract multi-scale features of brain tissues and harness complementary information from multi-modality MR images for fast and accurate segmentation. Extensive experiments on the well-known MRBrainS Challenge database corroborate the efficiency of the proposed model. Within approximately thirteen seconds, the MMAN can segment three different brain tissues from MRI data of each individual, that is faster than many existing methods. For the segmentation of gray matter, white matter, and cerebrospinal fluid, the MMAN achieved dice coefficients of 86.40%, 89.70% and 84.86%, respectively. Consequently, the proposed model outperformed many state-of-the-art methods and got the second place in the MRBrainS Challenge. Therefore, the proposed MMAN is promising for automated brain segmentation in clinical applications.
更多查看译文
关键词
Brain segmentation,Segmentation network,Multi-scale feature,Multi-modality MRI
AI 理解论文
溯源树
样例
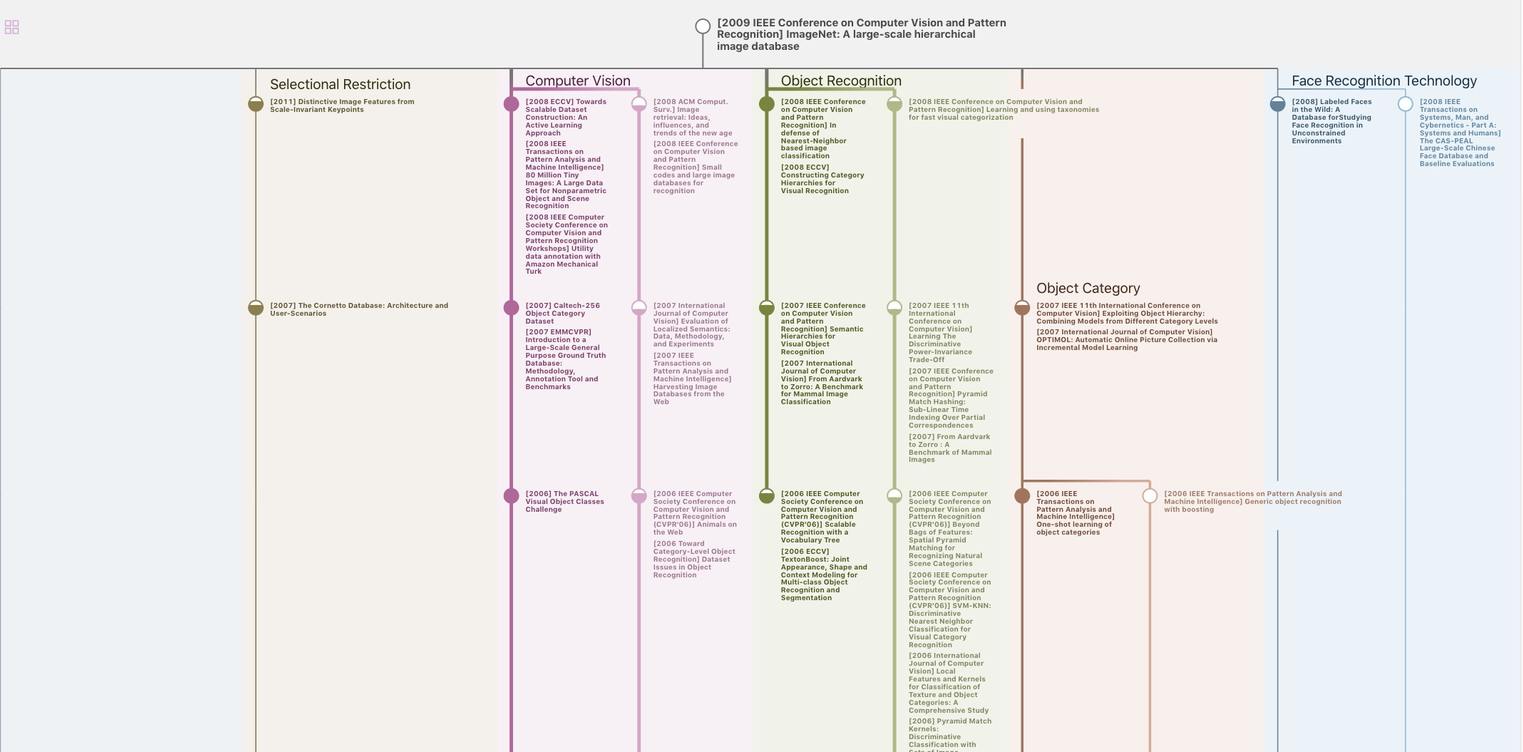
生成溯源树,研究论文发展脉络
Chat Paper
正在生成论文摘要