MFPR: A Personalized Ranking Recommendation with Multiple Feedback
ACM Transactions on Social Computing(2018)
摘要
Recently, recommender systems have played an important role in improving web user experiences and increasing profits. Recommender systems exploit users’ behavioral history (i.e., feedback on items) to build models. The feedback usually includes explicit feedback (e.g., ratings) and implicit feedback (e.g., browsing history, click logs), which are both useful for improving recommendations. However, as far as we are concerned, no existing works have integrated both explicit and multiple implicit feedback simultaneously. Therefore, we propose a unified and flexible model, named Multiple Feedback-based Personalized Ranking (MFPR), to make full use of multiple feedback, which uses a personalized ranking framework. To train model MFPR, we design an algorithm to generate ordered item pairs as labeled data, with consideration of both rating scores and multiple implicit feedback. Extensive experiments on two real-world datasets validate the effectiveness of the MFPR model. With the integration of multiple feedback, MFPR significantly improves recommendation performance.
更多查看译文
关键词
Recommender system, bayesian personalized ranking, multiple feedback
AI 理解论文
溯源树
样例
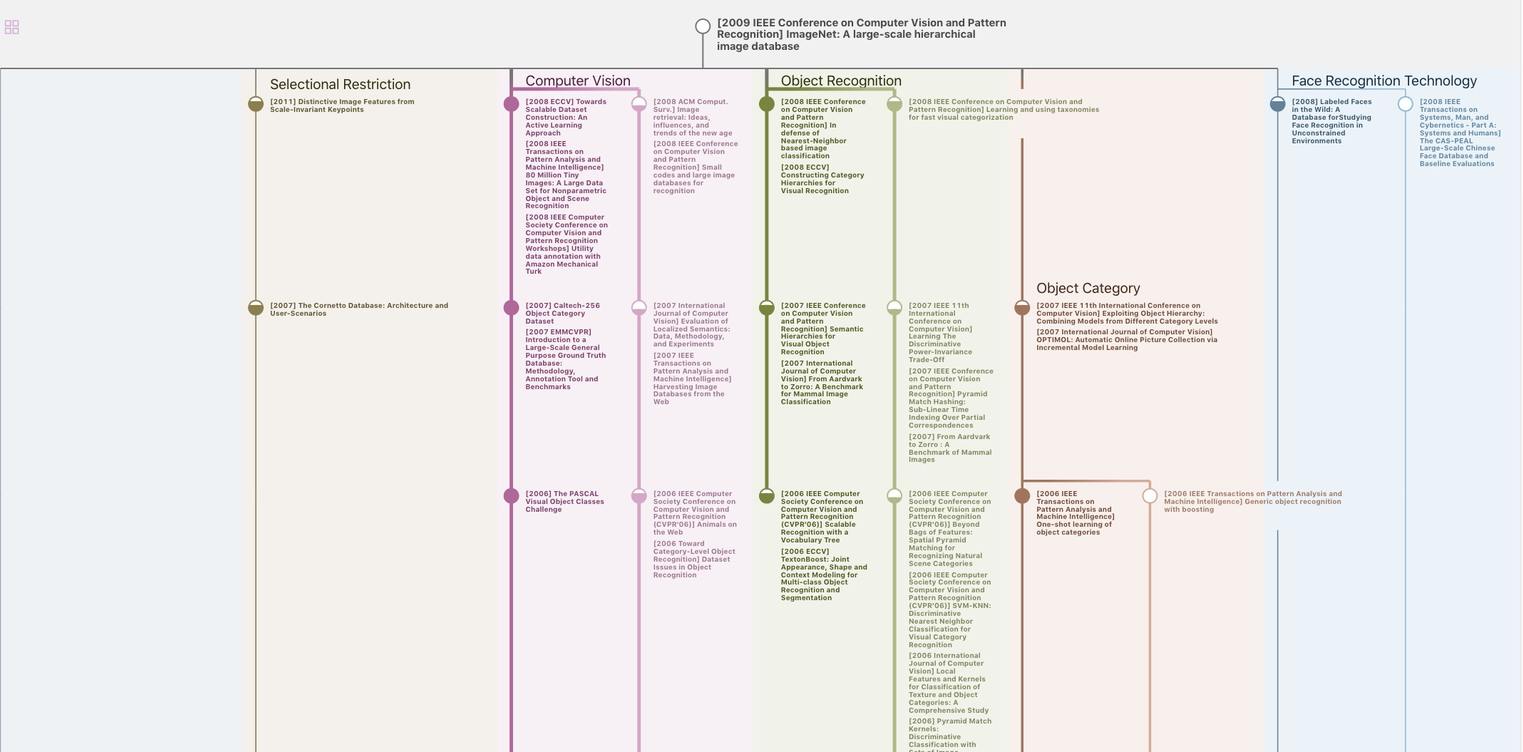
生成溯源树,研究论文发展脉络
Chat Paper
正在生成论文摘要