Detecting the Starting Frame of Actions in Video
2020 IEEE WINTER CONFERENCE ON APPLICATIONS OF COMPUTER VISION (WACV)(2020)
摘要
In this work, we address the problem of precisely localizing key frames of an action, for example, the precise time that a pitcher releases a baseball, or the precise time that a crowd begins to applaud. Key frame localization is a largely overlooked and important action-recognition problem, for example in the field of neuroscience, in which we would like to understand the neural activity that produces the start of a bout of an action. To address this problem, we introduce a novel structured loss function that properly weights the types of errors that matter in such applications: it more heavily penalizes extra and missed action start detections over small misalignments. Our structured loss is based on the best matching between predicted and labeled action starts. We train recurrent neural networks (RNNs) to minimize differentiable approximations of this loss. To evaluate these methods, we introduce the Mouse Reach Dataset, a large, annotated video dataset of mice performing a sequence of actions. The dataset was collected and labeled by experts for the purpose of neuroscience research. On this dataset, we demonstrate that our method outperforms related approaches and baseline methods using an unstructured loss.
更多查看译文
关键词
key frame localization,action recognition,neural activity,structured loss function,neural networks,Mouse Reach Dataset,starting frame detection,video annotation dataset,missed action start detection,extra action start detection,recurrent neural networks,RNN
AI 理解论文
溯源树
样例
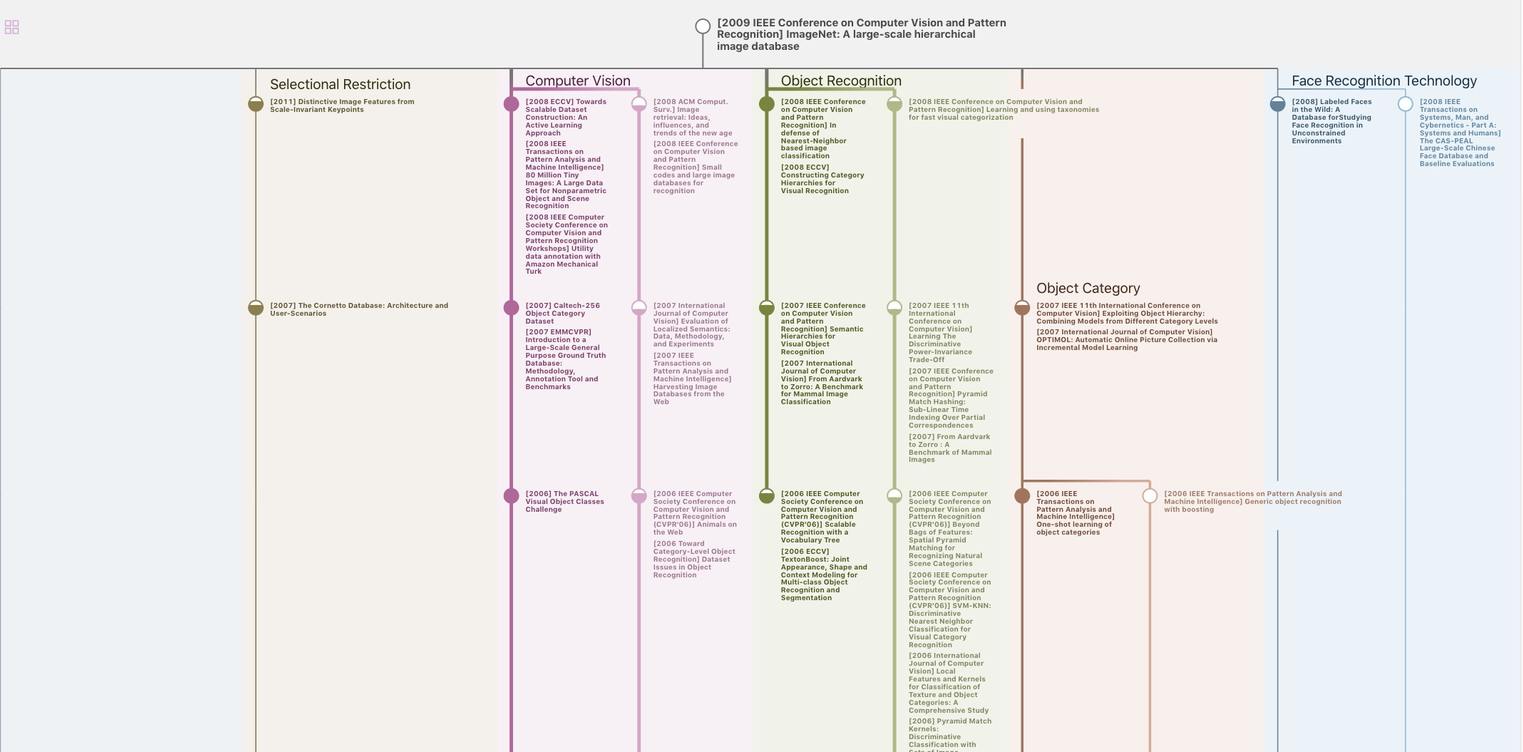
生成溯源树,研究论文发展脉络
Chat Paper
正在生成论文摘要