Adaptive Nonparametric Variational Autoencoder.
CoRR(2019)
摘要
Clustering is used to find structure in unlabeled data by grouping similar objects together. Cluster analysis depends on the definition of similarity in the feature space. In this paper, we propose an Adaptive Nonparametric Variational Autoencoder (AdapVAE) to perform end-to-end feature learning from raw data jointly with cluster membership learning through a Nonparametric Bayesian modeling framework with deep neural networks. It has the advantage of avoiding pre-definition of similarity or feature engineering. Our model relaxes the constraint of fixing the number of clusters in advance by assigning a Dirichlet Process prior on the latent representation in a low-dimensional feature space. It can adaptively detect novel clusters when new data arrives based on a learned model from historical data in an online unsupervised learning setting. We develop a joint online variational inference algorithm to learn feature representations and cluster assignments via iteratively optimizing the evidence lower bound (ELBO). Our experimental results demonstrate the capacity of our modelling framework to learn the number of clusters automatically using data, the flexibility to detect novel clusters with emerging data adaptively, the ability of high quality reconstruction and generation of samples without supervised information and the improvement over state-of-the-art end-to-end clustering methods in terms of accuracy on both image and text corpora benchmark datasets.
更多查看译文
AI 理解论文
溯源树
样例
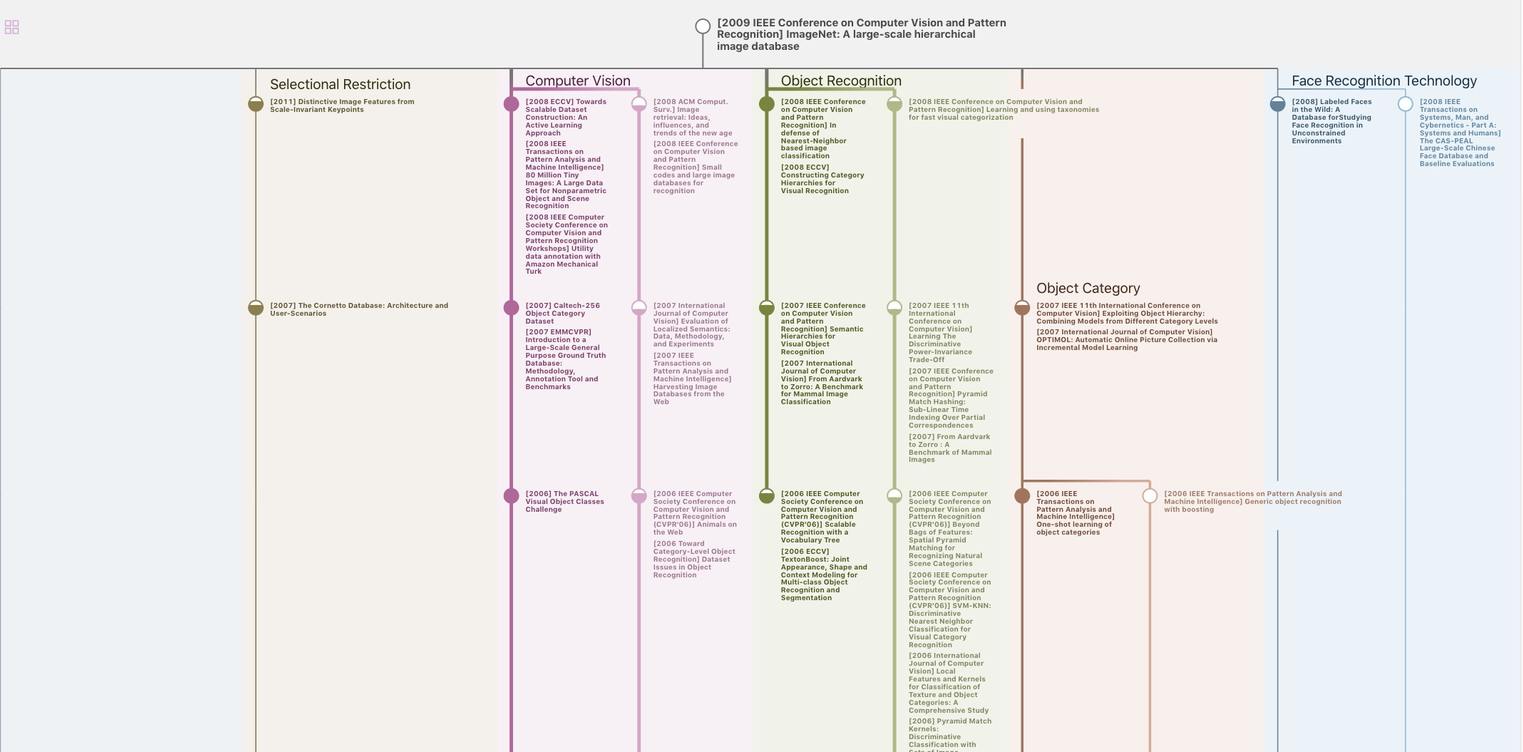
生成溯源树,研究论文发展脉络
Chat Paper
正在生成论文摘要