Risky Action Recognition in Lane Change Video Clips using Deep Spatiotemporal Networks with Segmentation Mask Transfer
2019 IEEE INTELLIGENT TRANSPORTATION SYSTEMS CONFERENCE (ITSC)(2019)
摘要
Advanced driver assistance and automated driving systems rely on risk estimation modules to predict and avoid dangerous situations. Current methods use expensive sensor setups and complex processing pipelines, limiting their availability and robustness. To address these issues, we introduce a novel deep learning based driving risk assessment framework for classifying dangerous lane change behavior in short video clips captured by a monocular camera. First, semantic segmentation masks were generated from individual video frames with a pre-trained Mask R-CNN model. Then, frames overlayed with these masks were fed into a time distributed CNN-LSTM network with a final softmax classification layer. This network was trained on a semi-naturalistic lane change dataset with annotated risk labels. A comprehensive comparison of state-of-the-art pre-trained feature extractors was carried out to find the best network layout and training strategy. The best result, with a 0.937 AUC score, was obtained with the proposed framework. Our code and trained models are available open-source.
更多查看译文
关键词
time distributed CNN-LSTM network,lane change behavior classification,pretrained feature extractors,pretrained mask R-CNN model,softmax classification layer,monocular camera,risk assessment,deep learning,risk estimation,automated driving systems,advanced driver assistance system,segmentation mask transfer,deep spatiotemporal networks,lane change video clips,risky action recognition
AI 理解论文
溯源树
样例
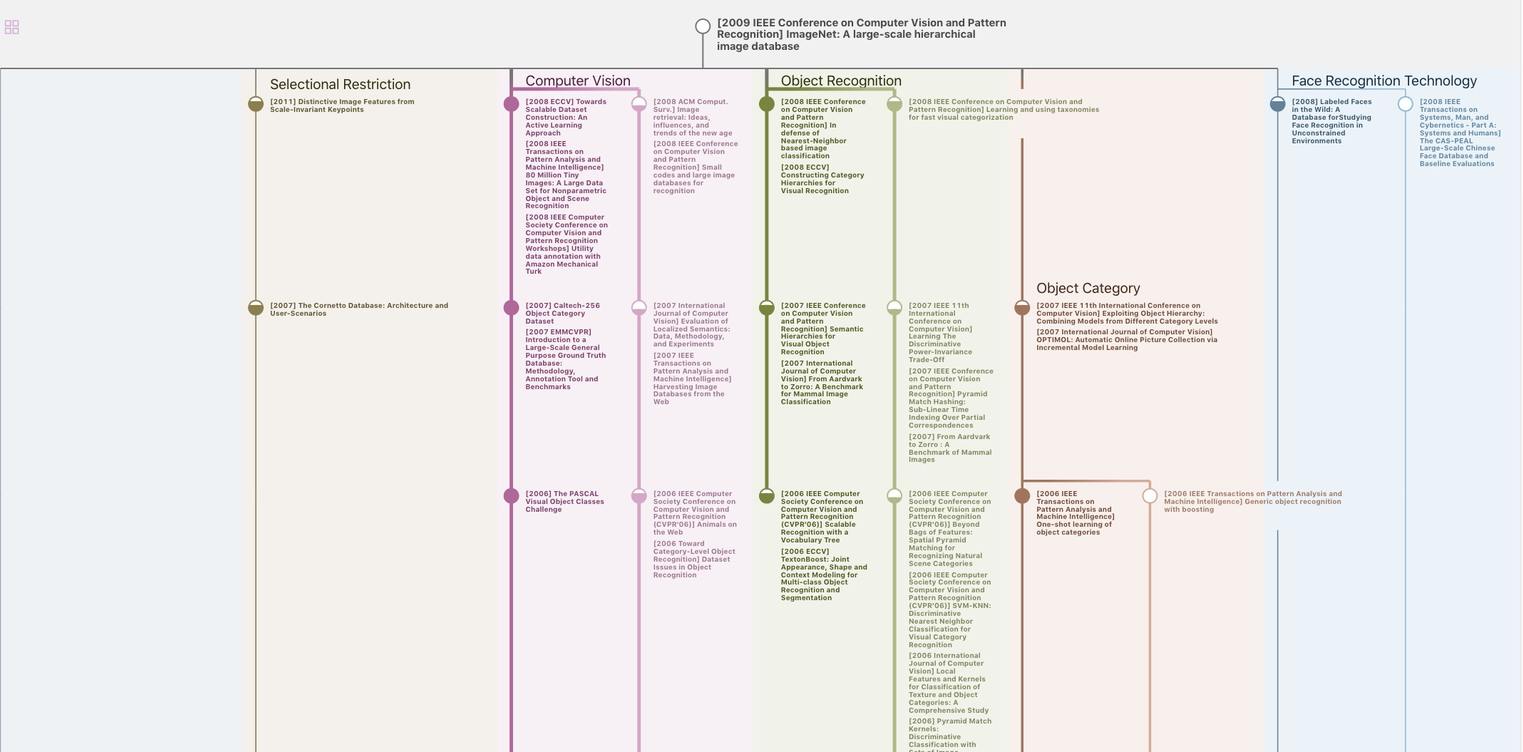
生成溯源树,研究论文发展脉络
Chat Paper
正在生成论文摘要