Motion compensation in extremity cone-beam computed tomography
Skeletal radiology(2019)
摘要
Objectives To evaluate the improvement in extremity cone-beam computed tomography (CBCT) image quality in datasets with motion artifact using a motion compensation method based on maximizing image sharpness. Methods Following IRB approval, retrospective analysis of 308 CBCT scans of lower extremities was performed by a fellowship-trained musculoskeletal radiologist to identify images with moderate to severe motion artifact. Twenty-four scans of 22 patients (18 male, four female; mean, 32 years old, range, 21–74 years old) were chosen for inclusion. Sharp (bone) and smooth (soft tissue) reconstructions were processed using the motion compensation algorithm. Two experts rated visualization of trabecular bone, cortical bone, joint spaces, and tendon on a nine-level Likert scale with and without motion compensation (a total of 96 datasets). Visual grading characteristics (VGC) was used to quantitatively determine the difference in image quality following motion compensation. Intra-class correlation coefficient (ICC) was obtained to assess inter-observer agreement. Results Motion-compensated images exhibited appreciable reduction in artifacts. The observer study demonstrated the associated improvement in diagnostic quality. The fraction of cases receiving scores better than “Fair” increased from less than 10% without compensation to 40–70% following compensation, depending on the task. The area under the VGC curve was 0.75 (tendon) to 0.85 (cortical bone), confirming preference for motion compensated images. ICC values showed excellent agreement between readers before (ICC range, 0.8–0.91) and after motion compensation (ICC range, 0.92–0.97). Conclusions The motion compensation algorithm significantly improved the visualization of bone and soft tissue structures in extremity CBCT for cases exhibiting patient motion.
更多查看译文
关键词
Extremity cone-beam CT,Motion compensation,Visual grading characteristic,Weight-bearing
AI 理解论文
溯源树
样例
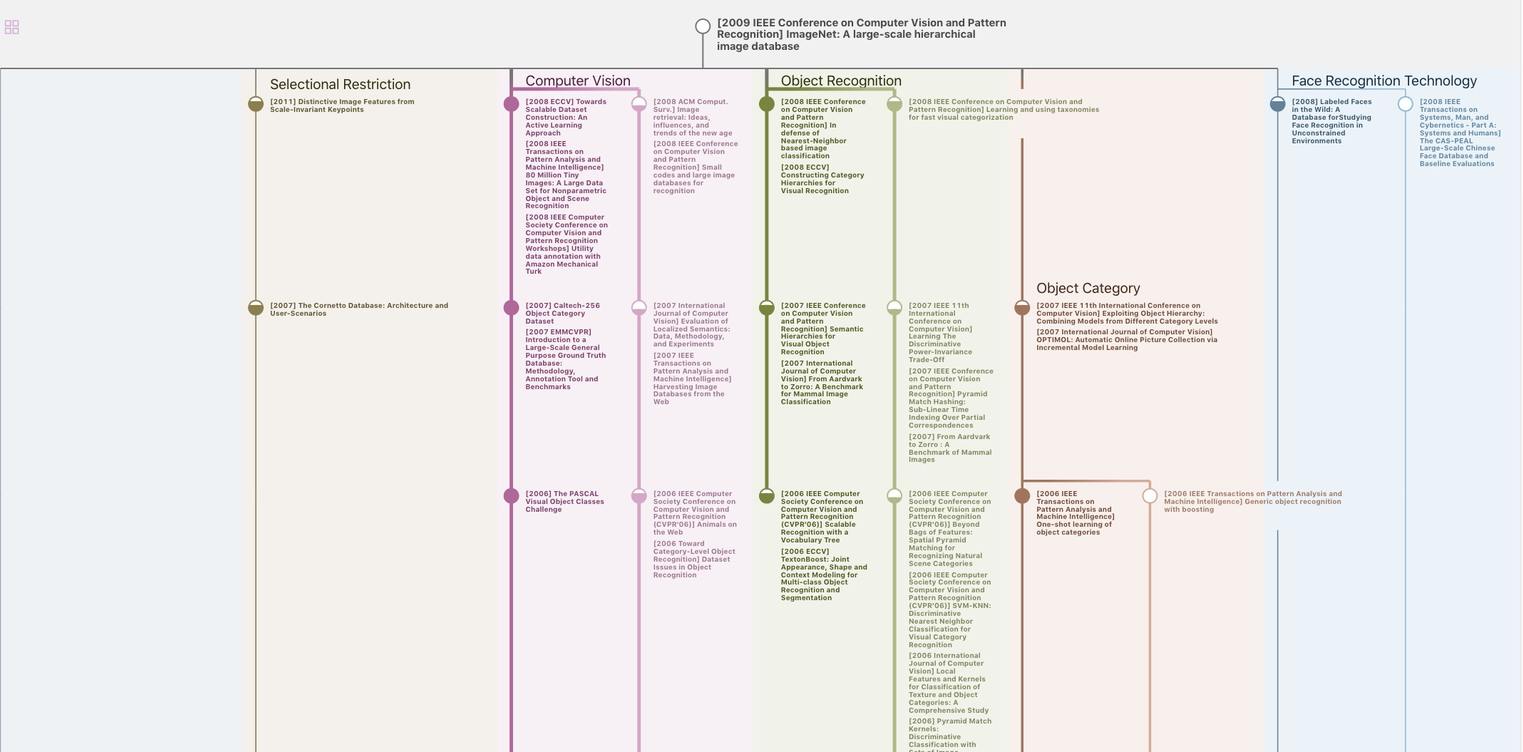
生成溯源树,研究论文发展脉络
Chat Paper
正在生成论文摘要