Robustifying models against adversarial attacks by Langevin dynamics
Neural Networks(2021)
摘要
Adversarial attacks on deep learning models have compromised their performance considerably. As remedies, a number of defense methods were proposed, which however, have been circumvented by newer and more sophisticated attacking strategies. In the midst of this ensuing arms race, the problem of robustness against adversarial attacks still remains a challenging task. This paper proposes a novel, simple yet effective defense strategy where off-manifold adversarial samples are driven towards high density regions of the data generating distribution of the (unknown) target class by the Metropolis-adjusted Langevin algorithm (MALA) with perceptual boundary taken into account. To achieve this task, we introduce a generative model of the conditional distribution of the inputs given labels that can be learned through a supervised Denoising Autoencoder (sDAE) in alignment with a discriminative classifier. Our algorithm, called MALA for DEfense (MALADE), is equipped with significant dispersion—projection is distributed broadly. This prevents white box attacks from accurately aligning the input to create an adversarial sample effectively. MALADE is applicable to any existing classifier, providing robust defense as well as off-manifold sample detection. In our experiments, MALADE exhibited state-of-the-art performance against various elaborate attacking strategies.
更多查看译文
关键词
Adversarial examples,Robustness,Langevin dynamics
AI 理解论文
溯源树
样例
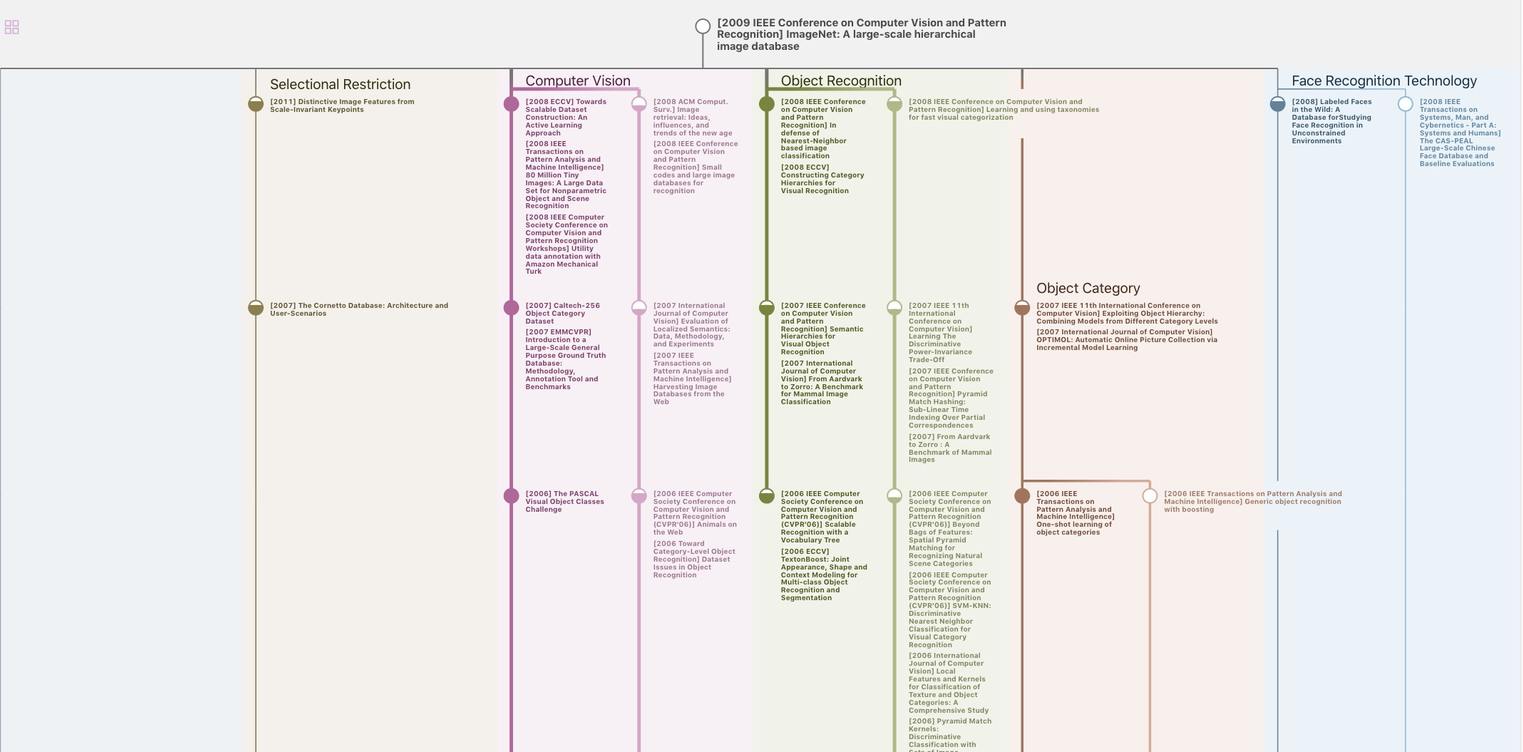
生成溯源树,研究论文发展脉络
Chat Paper
正在生成论文摘要