DeepDirect: Learning Directions of Social Ties with Edge-Based Network Embedding (Extended Abstract)
2019 IEEE 35th International Conference on Data Engineering (ICDE)(2019)
摘要
This paper presents the problem of tie direction learning which learns the directionality function of directed social networks. One way is based on hand-crafted features; the other called DeepDirect learns the social tie representation through the network topology. DeepDirect directly maps social ties to low-dimensional embedding vectors by preserving network topology, utilizing labeled data, and generating pseudo-labels based on observed directionality patterns. Experimental results on two tasks, i.e., direction discovery on undirected ties and direction quantification on bidirectional ties, demonstrate the proposed methods are effective and promising.
更多查看译文
关键词
Social networking (online),Network topology,Task analysis,Knowledge engineering,Topology,Conferences,Data engineering
AI 理解论文
溯源树
样例
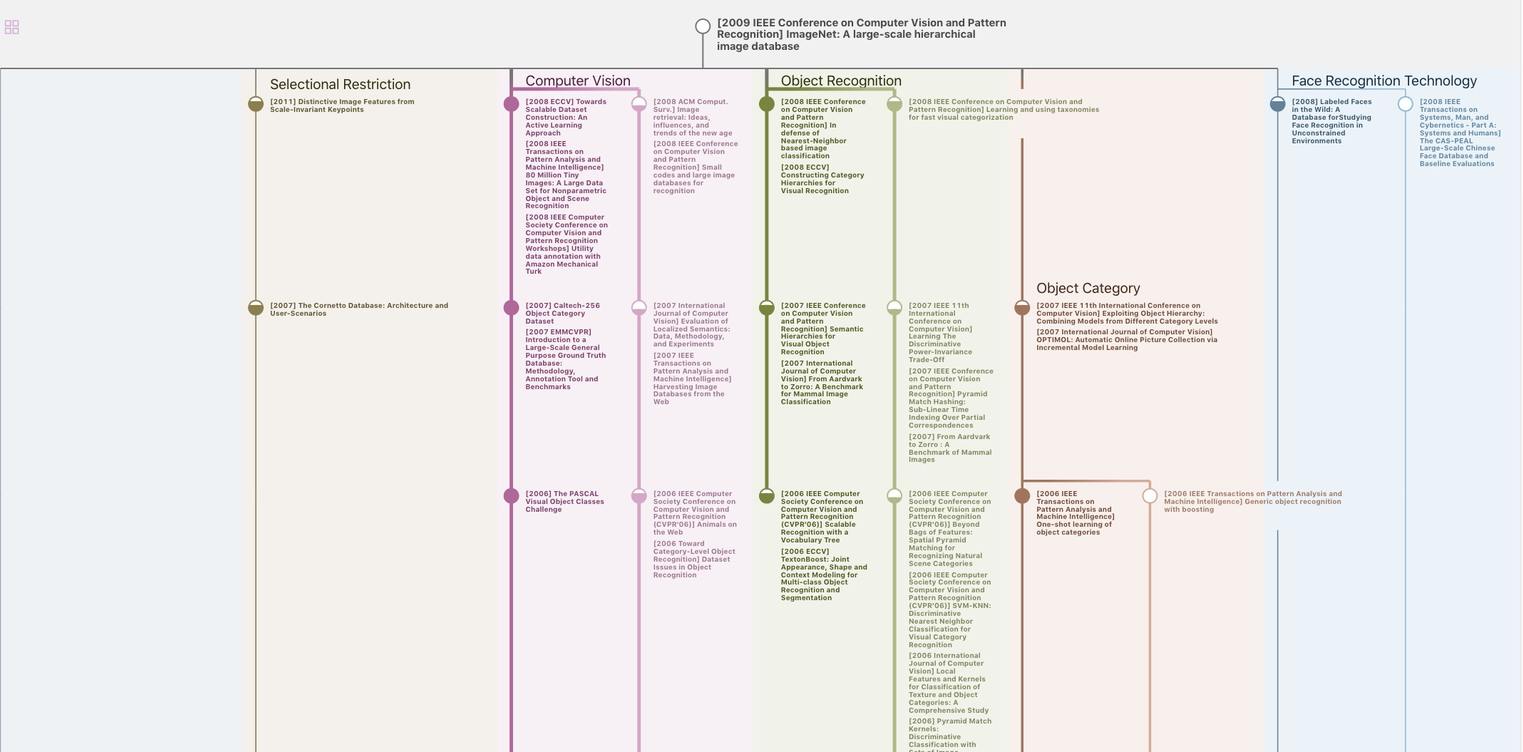
生成溯源树,研究论文发展脉络
Chat Paper
正在生成论文摘要