On-line AUV Survey Planning for Finding Safe Vessel Paths through Hazardous Environments*
2018 IEEE/OES Autonomous Underwater Vehicle Workshop (AUV)(2018)
摘要
Autonomous underwater vehicles (AUVs) are robotic platforms that are commonly used to map the sea floor, for example for benthic surveys or for naval mine countermeasures (MCM) operations. AUVs create an acoustic image of the survey area, such that objects on the seabed can be identified and, in the case of MCM, mines can be found and disposed of. The common method for creating such seabed maps is to run a lawnmower survey, which is a standard method in coverage path planning. We are interested in exploring alternate techniques for surveying areas of interest, in order to reduce mission time and/or assess feasible actions, such as finding a safe path through a hazardous region. In this paper, we use Gaussian Process (GP) regression to build models of seabed complexity data. We evaluate five commonly used kernels to assess modeling performance. Our results show that an additive Matérn kernel is most suitable for modeling seabed complexity data. On top of the GP model, we use adaptations of two standard path planning methods, A* and RRT*, to find potentially safe paths for marine vessels through the modeled areas. We evaluate the planned paths in terms of length and complexity, and run a vehicle dynamics simulator to assess potential performance by a marine vessel. Finally, we propose three approaches for on-line AUV survey path planning. The AUV decides on-line where to survey, based on the GP model it is creating and the GP’s uncertainty along potential safe paths for marine vessels. Our simulation results show that using onboard on-line AUV survey planning greatly reduces survey time needed by the AUV compared to running a full lawnmower survey with off-line vessel path planning.
更多查看译文
关键词
Kernel,Complexity theory,Path planning,Data models,Planning,Adaptation models,Additives
AI 理解论文
溯源树
样例
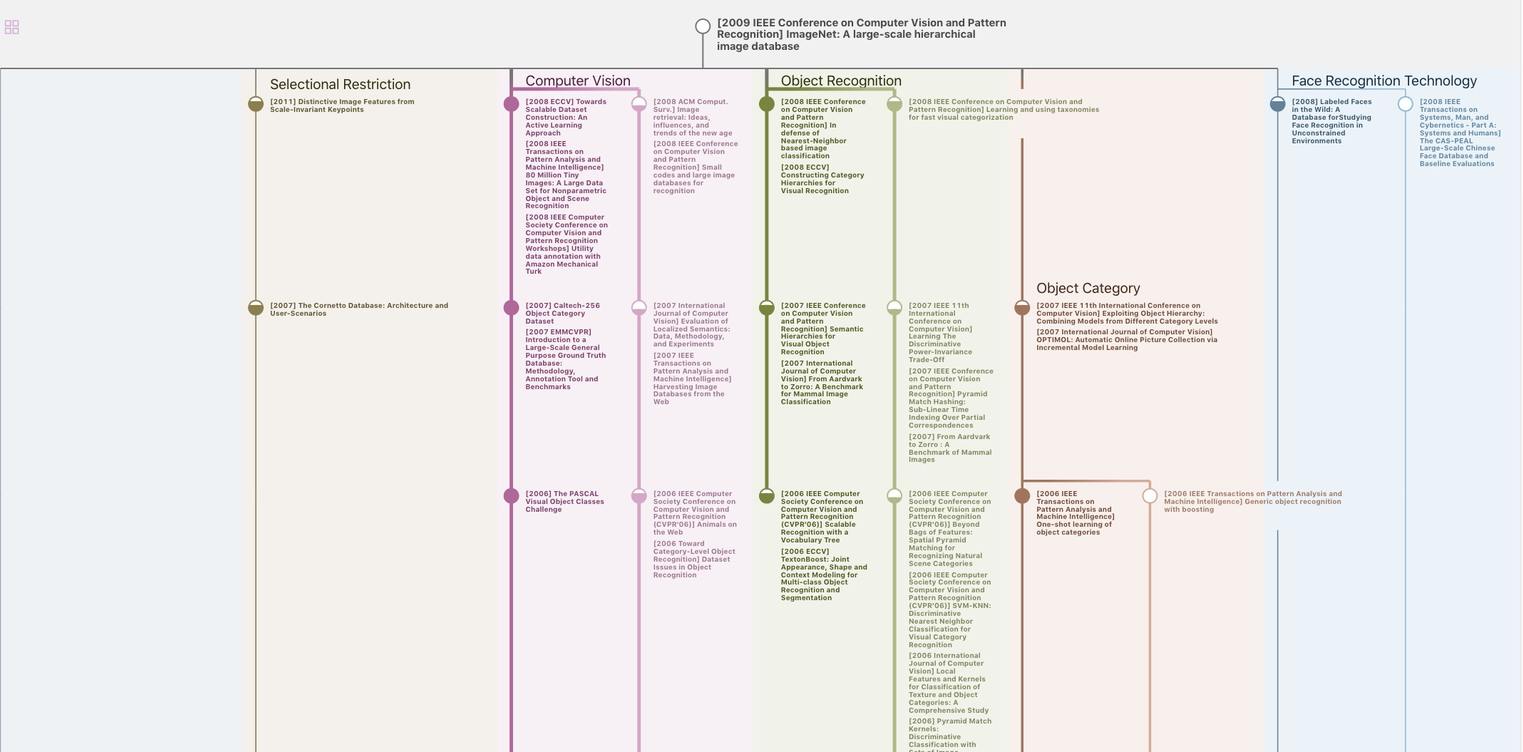
生成溯源树,研究论文发展脉络
Chat Paper
正在生成论文摘要