Triple-To-Text: Converting Rdf Triples Into High-Quality Natural Languages Via Optimizing An Inverse Kl Divergence
PROCEEDINGS OF THE 42ND INTERNATIONAL ACM SIGIR CONFERENCE ON RESEARCH AND DEVELOPMENT IN INFORMATION RETRIEVAL (SIGIR '19)(2019)
摘要
Knowledge base is one of the main forms to represent information in a structured way. A knowledge base typically consists of Resource Description Frameworks (RDF) triples which describe the entities and their relations. Generating natural language description of the knowledge base is an important task in NLP, which has been formulated as a conditional language generation task and tackled using the sequence-to-sequence framework. Current works mostly train the language models by maximum likelihood estimation, which tends to generate lousy sentences. In this paper, we argue that such a problem of maximum likelihood estimation is intrinsic, which is generally irrevocable via changing network structures. Accordingly, we propose a novel Triple-to-Text (T2T) framework, which approximately optimizes the inverse Kullback-Leibler (KL) divergence between the distributions of the real and generated sentences. Due to the nature that inverse KL imposes large penalty on fake-looking samples, the proposed method can significantly reduce the probability of generating low-quality sentences. Our experiments on three real-world datasets demonstrate that T2T can generate higher-quality sentences and outperform baseline models in several evaluation metrics.
更多查看译文
关键词
Natural Language Generation,Sequence to Sequence Generation,Knowledge Bases
AI 理解论文
溯源树
样例
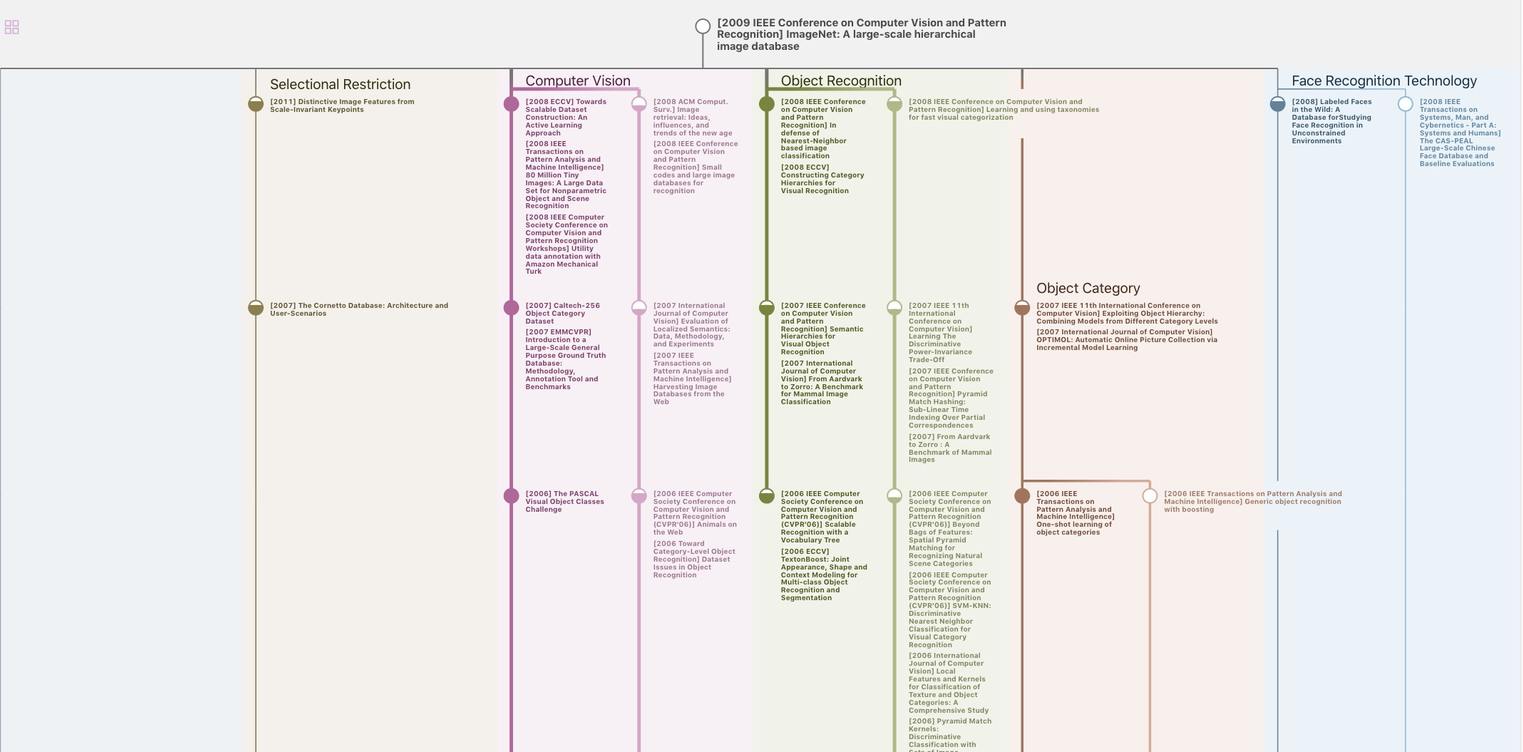
生成溯源树,研究论文发展脉络
Chat Paper
正在生成论文摘要