Dynamic Neural Network Decoupling.
CoRR(2019)
摘要
Convolutional neural networks (CNNs) have achieved a superior performance by taking advantages of the complex network architectures and huge numbers of parameters, which however become uninterpretable and challenge their full potential to practical applications. Towards better understand the rationale behind the network decisions, we propose a novel architecture decoupling method, which dynamically discovers the hierarchical path consisting of activated filters for each input image. In particular, architecture controlling module is introduced in each layer to encode the network architecture and identify the activated filters corresponding to the specific input. Then, mutual information between architecture encoding and the attribute of input image is maximized to decouple the network architecture, and subsequently disentangles the filters by limiting the outputs of filter during training. Extensive experiments show that several merits have been achieved based on the proposed architecture decoupling, i.e., interpretation, acceleration and adversarial attacking.
更多查看译文
关键词
decoupling,neural network
AI 理解论文
溯源树
样例
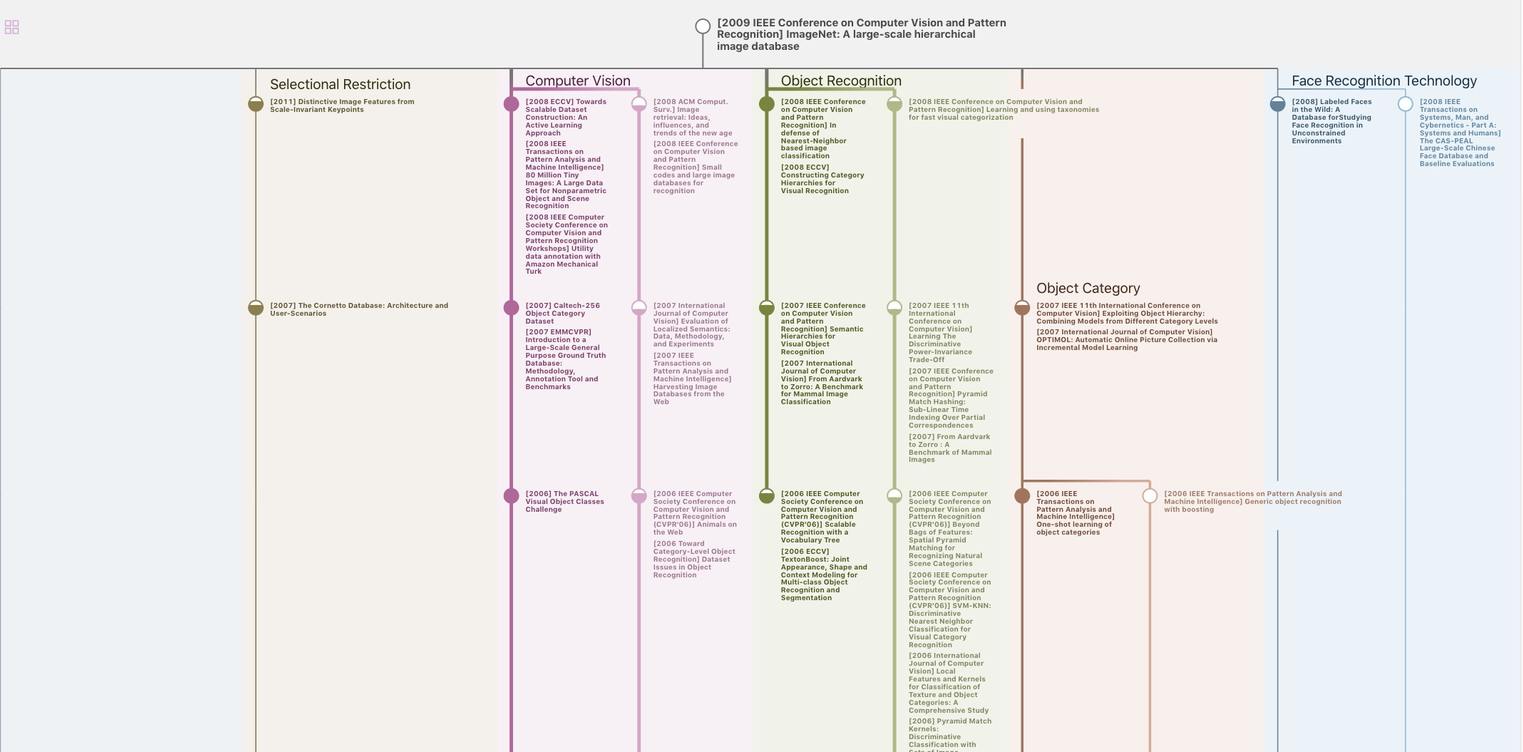
生成溯源树,研究论文发展脉络
Chat Paper
正在生成论文摘要