A Low Complexity Orthogonal Least Squares Algorithm for Sparse Signal Recovery
2018 International Conference on Signal Processing and Communications (SPCOM)(2018)
摘要
Recently, multiple orthogonal least squares (mOLS) was proposed as an extension of the well known orthogonal least squares (OLS) algorithm, which generalizes the support identification strategy of OLS by selecting multiple columns per iteration, thereby enhancing the convergence rate significantly. In this paper, we propose a modified multiple least squares algorithm, termed as m
2
OLS, which refines the mOLS iteration step by first preselecting a subset of columns of the measurement matrix by a suitable “greedy” principle. The selected subset is then used for identification of multiple, possibly “true” support indices using mOLS. Restricting the computationally demanding mOLS calculations only to a smaller subset of the columns reduces the overall computational overhead substantially. Further, like mOLS, preselection in m
2
OLS is also based on correlation of the columns of the measurement matrix with the residual vector, thus enabling m
2
OLS to achieve similar recovery performance as mOLS - a fact that is also borne out by simulation studies. The paper presents convergence conditions for the proposed m
2
OLS for both noise free and noisy observations. It also provides a comparative complexity analysis of mOLS and m
2
OLS demonstrating the superior computational performance of m
2
OLS vis-a-vis mOLS.
更多查看译文
关键词
Convergence,Matching pursuit algorithms,Correlation,Noise measurement,Mathematical model,Indexes,Sparse matrices
AI 理解论文
溯源树
样例
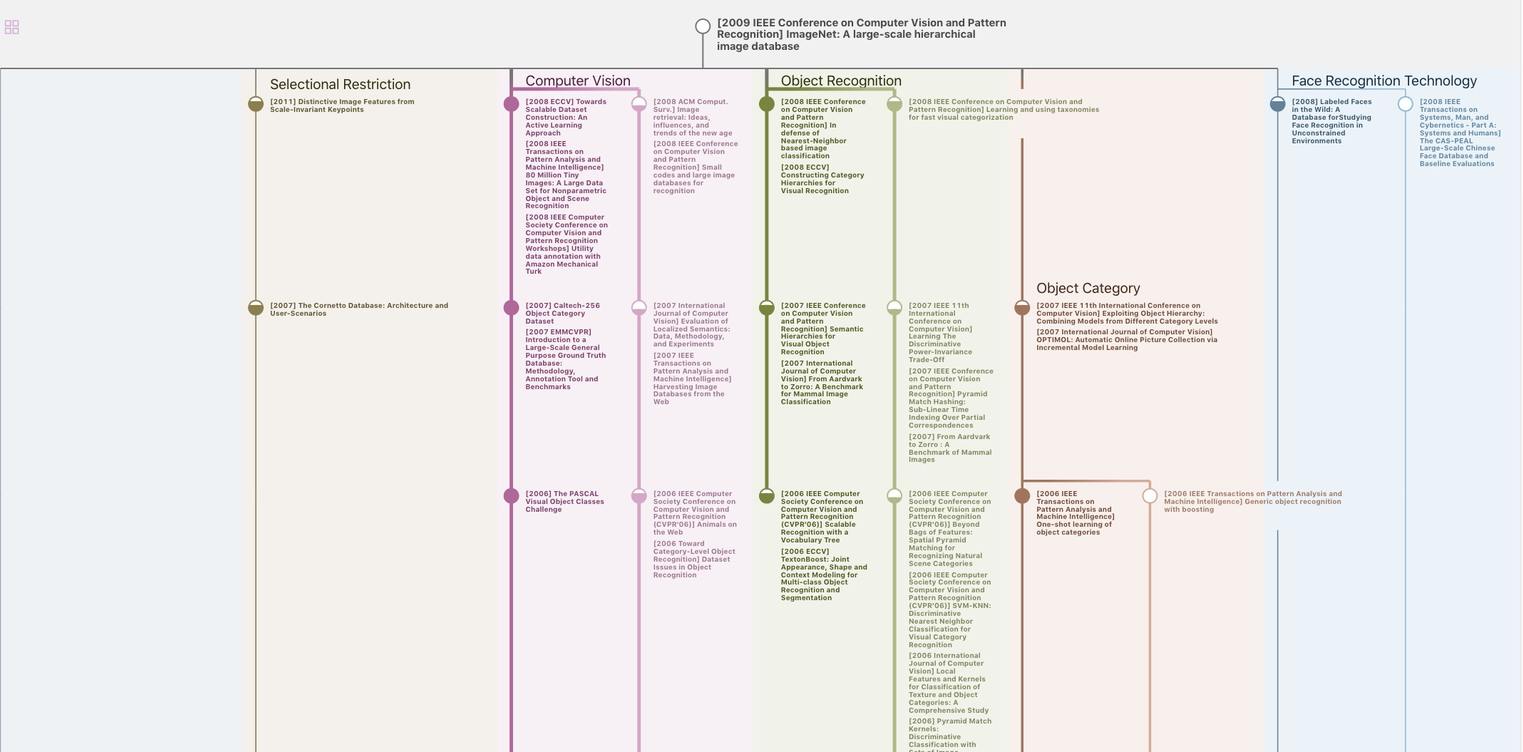
生成溯源树,研究论文发展脉络
Chat Paper
正在生成论文摘要