Neural Entropic Estimation: A faster path to mutual information estimation.
CoRR(2019)
摘要
We point out a limitation of the mutual information neural estimation (MINE) where the network fails to learn at the initial training phase, leading to slow convergence in the number of training iterations. To solve this problem, we propose a faster method called the mutual information neural entropic estimation (MI-NEE). Our solution first generalizes MINE to estimate the entropy using a custom reference distribution. The entropy estimate can then be used to estimate the mutual information. We argue that the seemingly redundant intermediate step of entropy estimation allows one to improve the convergence by an appropriate reference distribution. In particular, we show that MI-NEE reduces to MINE in the special case when the reference distribution is the product of marginal distributions, but faster convergence is possible by choosing the uniform distribution as the reference distribution instead. Compared to the product of marginals, the uniform distribution introduces more samples in low-density regions and fewer samples in high-density regions, which appear to lead to an overall larger gradient for faster convergence.
更多查看译文
关键词
neural entropic estimation,mutual information estimation
AI 理解论文
溯源树
样例
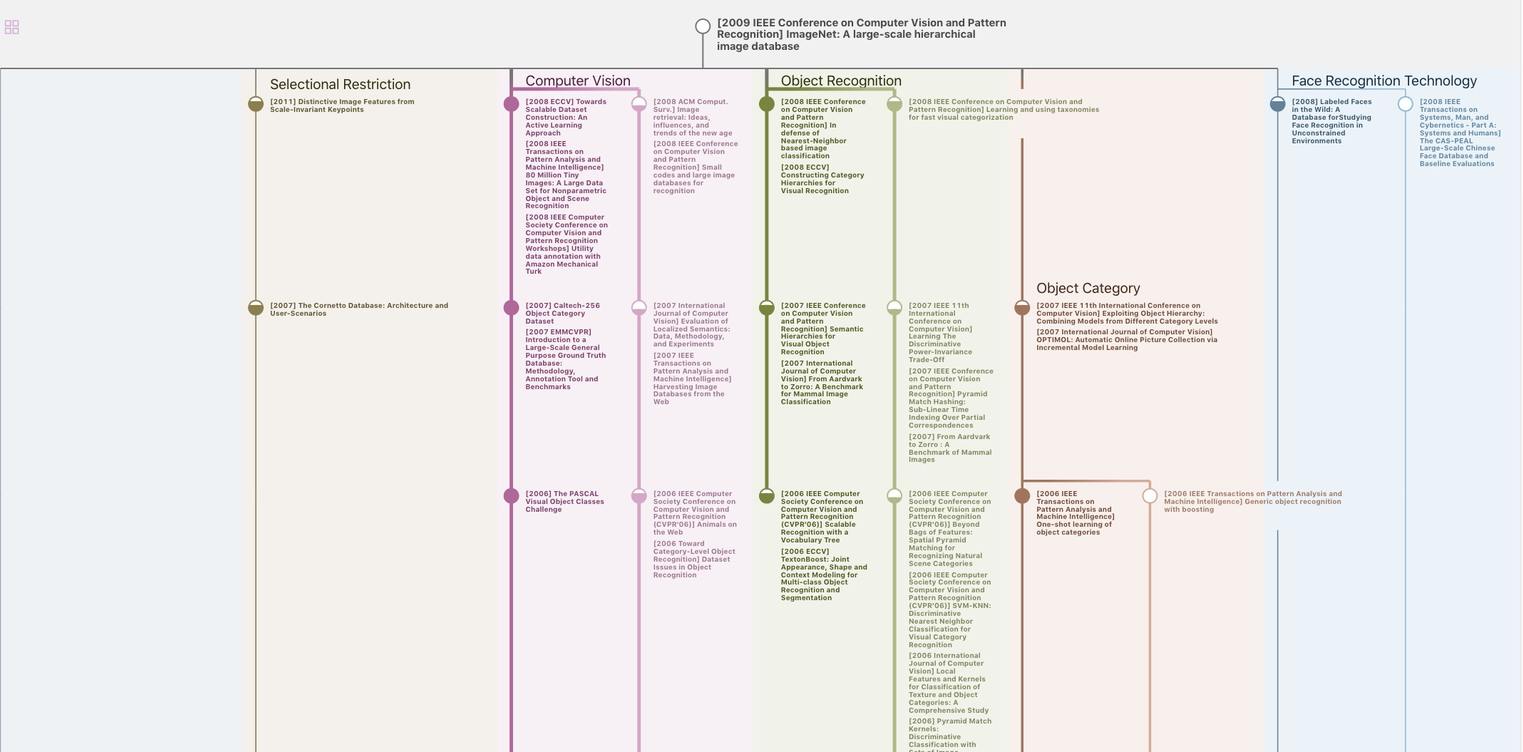
生成溯源树,研究论文发展脉络
Chat Paper
正在生成论文摘要