Controllable Unsupervised Text Attribute Transfer via Editing Entangled Latent Representation.
ADVANCES IN NEURAL INFORMATION PROCESSING SYSTEMS 32 (NIPS 2019)(2019)
摘要
Unsupervised text attribute transfer automatically transforms a text to alter a specific attribute (e.g. sentiment) without using any parallel data, while simultaneously preserving its attribute-independent content. The dominant approaches are trying to model the content-independent attribute separately, e.g., learning different attributes' representations or using multiple attribute-specific decoders. However, it may lead to inflexibility from the perspective of controlling the degree of transfer or transferring over multiple aspects at the same time. To address the above problems, we propose a more flexible unsupervised text attribute transfer framework which replaces the process of modeling attribute with minimal editing of latent representations based on an attribute classifier. Specifically, we first propose a Transformer-based autoencoder to learn an entangled latent representation for a discrete text, then we transform the attribute transfer task to an optimization problem and propose the Fast-Gradient-Iterative-Modification algorithm to edit the latent representation until conforming to the target attribute. Extensive experimental results demonstrate that our model achieves very competitive performance on three public data sets. Furthermore, we also show that our model can not only control the degree of transfer freely but also allow transferring over multiple aspects at the same time.
更多查看译文
关键词
optimization problem,optimization problems
AI 理解论文
溯源树
样例
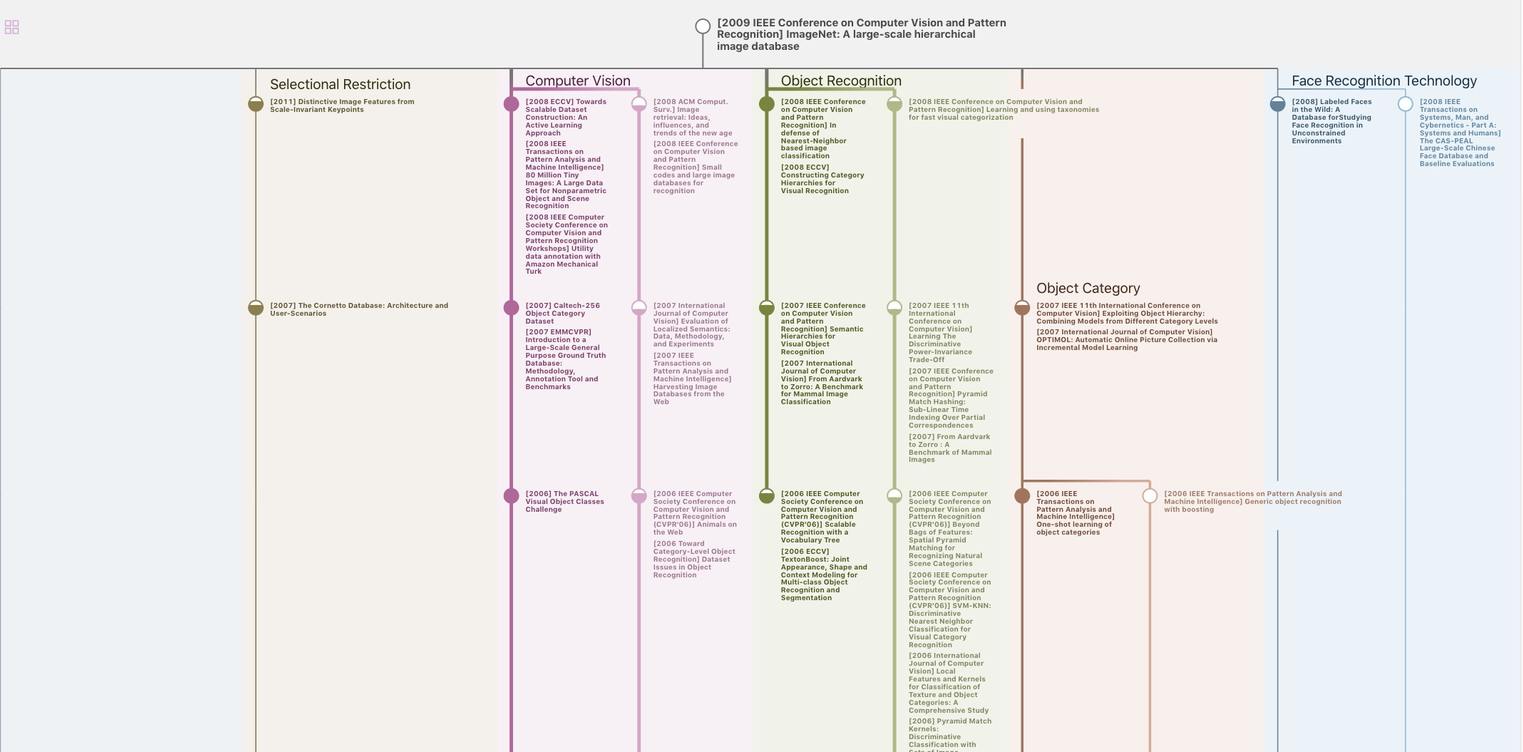
生成溯源树,研究论文发展脉络
Chat Paper
正在生成论文摘要