Multivariate Distributionally Robust Convex Regression under Absolute Error Loss
ADVANCES IN NEURAL INFORMATION PROCESSING SYSTEMS 32 (NIPS 2019)(2019)
摘要
This paper proposes a novel non-parametric multidimensional convex regression estimator which is designed to be robust to adversarial perturbations in the empirical measure. We minimize over convex functions the maximum (over Wasserstein perturbations of the empirical measure) of the absolute regression errors. The inner maximization is solved in closed form resulting in a regularization penalty involves the norm of the gradient. We show consistency of our estimator and a rate of convergence of order (O) over tilde (n(-1/d)), matching the bounds of alternative estimators based on square-loss minimization. Contrary to all of the existing results, our convergence rates hold without imposing compactness on the underlying domain and with no a priori bounds on the underlying convex function or its gradient norm.
更多查看译文
关键词
closed form
AI 理解论文
溯源树
样例
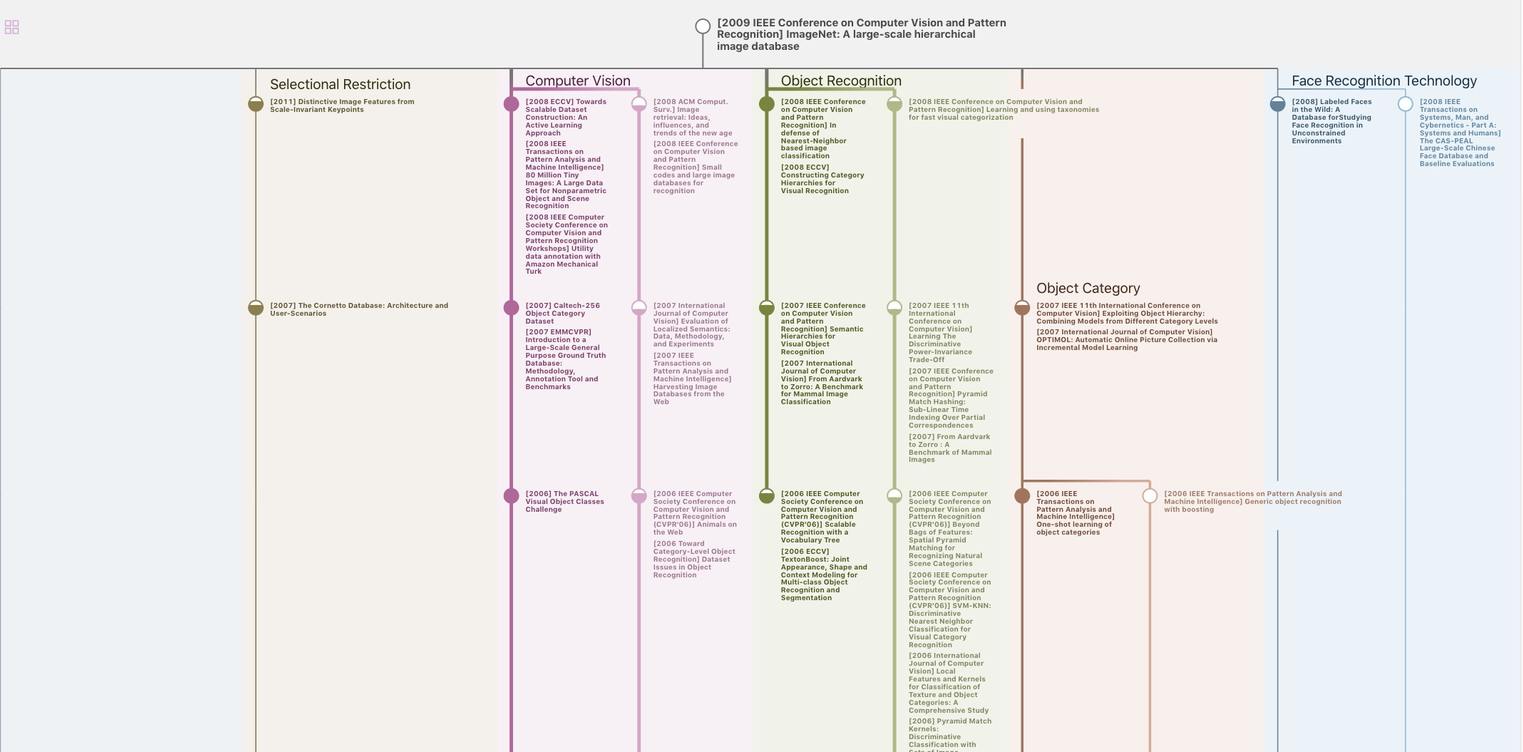
生成溯源树,研究论文发展脉络
Chat Paper
正在生成论文摘要